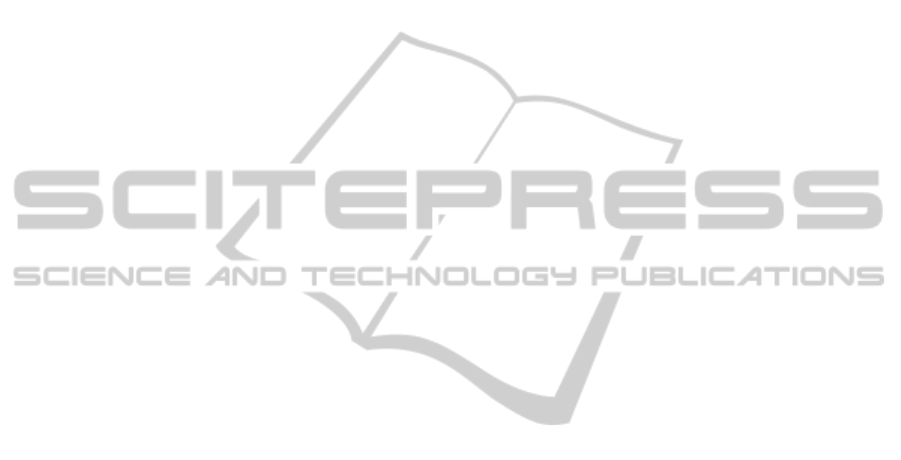
by Strötgen and Gertz (2010). In that work,
spatiotemporal information of a document is
extracted through the processing of the text and can
be explored by users after a query. However, the
means to evaluate the temporal ranking of each
document are not supplied.
The analysis of the above studies shows that the
temporal information retrieval is still an open
problem. This analysis also shows that many studies
explore the temporal dimension during the
resolution of queries, but do not use (or use
superficially) this information to establish the
ranking of the retrieved results. This highlights the
need for a more specific ranking, generated from a
deeper analysis of this kind of information.
Moreover, we can notice the lack of effective
solutions to retrieve temporal data in the geospatial
domain. This limitation, allied to the key importance
that the time represents for this domain, highlights
the importance of the work presented in this paper.
7 CONCLUSIONS
The temporal dimension has great importance for the
retrieval of geographic data. However, the retrieval
of geographic data with basis on temporal criteria is
still a hard task for the present SDIs. The absence of
a detailed description of the temporal extension of
the services and the lack of a temporal ranking are
some of the characteristics that cause this limitation.
Aiming to overcome those limitations, this paper
described a new temporal search engine. The main
contributions consist in the development of a new
model that improves the description of the temporal
extension at service and feature type levels, and the
development of a ranking for the feature types
retrieved during a query.
Some future works still should be undertaken to
improve our research. An important task to be
developed consists of extending our approach to
handle others types of temporal information, such as
imprecise temporal information. Besides, we should
improve the integration of our temporal search
engine with the other similarity metrics. This task
will enable us to evaluate the performance of our
tool when solving queries concerning more then one
dimension. Finally, other important improvement to
be undertaken consists of integrating our solution to
the current catalog service interface.
REFERENCES
Allen, J. F., (1998). Maintaining Knowledge about
Temporal Intervals. Communications of the ACM,
26(11), 832-843.
Alonso, O., Gertz, M. and Baeza-Yates, R. A., (2006).
Clustering of search results using temporal attributes.
In 29th Annual International ACM SIGIR Conference
on Research and Development in Information
Retrieval. ACM Press.
Andrade, F.G. and Baptista, C. S., (2011). Using Semantic
Similarity to Improve Information Discovery in
Spatial Data Infrastructures. Journal of Information
and Data Management, 2(2), 181-194.
Baeza-Yates, R. and Ribeiro-Neto, B., (1999). Modern
information Retrieval. Wokingham: Addison-Wesley.
Bernard, L., and Craglia, M. (2005). SDI - From Spatial
Data Infrastructure to Service Driven Infrastructure. In
Workshop on Cross-learning between Spatial Data
Infrastructures, and Information Infrastructures.
Fox, E. A. and Shaw, J. A., (1993). Combination of
Multiple Searches. In Second Text Retrieval
Conference. NIST Special Publications.
Hübner, S. and Visser, U., (2003). Temporal
Representation and Reasoning for the Semantic Web.
In Twenty-First International Florida Artificial
Intelligence Research Society Conference. AAAI
Press.
Jin, P., Li, X., Chen, H., and Yue, L., (2010). CT-Rank: A
Time-aware Ranking Algorithm for Web Search.
Journal of Convergence Information Technology, 5(6),
99-111.
Manica, E., Dorneles, C. F., and Galante, R. M., (2010).
Supporting Temporal Queries on XML Keyword
Search Engines. Journal of Information and Data
Management, 1(3), 471-486.
Open Geospatial Consortium. (2004). OGC Web Map
Service Interface. Retrieved February 26, 2012, from:
http://portal.opengeospatial.org/files/?artifact_id=4756
Open Geospatial Consortium, (2005). Web Feature
Service implementation specification. Retrieved
February 26, 2012, from: https://portal.opengeospatial.
org/files/?artifact_id=8339.
Pustejovsky J., Castaño J., Ingria R., Saurí R., Gaizauskas
R., Setzer A. and Katz G., (2006). TimeML: Robust
Specification of Event and Temporal Expressions in
Text. In Fifth International Workshop on
Computational Semantics.
Strötgen, J. and Gertz, M., (2010). A System for Exploring
Spatio-Temporal Information in Documents.
Proceedings of the VLDB Endowment, 3(1), 1569-
1572.
Tversky A., (1977). Features of similarity. Psychological
Review, 84 (4), 327-352.
Vögele, T., Hübner, S. and Shuster, G., (2003). BUSTER -
An Information Broker for the Semantic Web.
Künstliche Intelligenz, 17(3), 31- 34.
Williamson I., Rajabifard, A. and Feeney, M. F., (2003).
Developing Spatial Data Infrastructures: From
Concept to Reality. London: Taylor & Francis.
ATemporalSearchEnginetoImproveGeographicDataRetrievalinSpatialDataInfrastructures
65