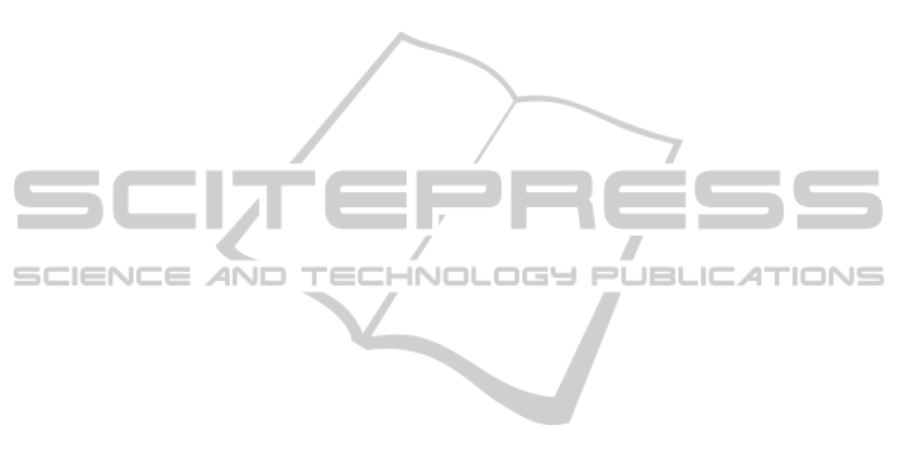
ACM, Springer and Web of Science. This action is
not supported by the tool and the search results must
be imported into StArt, As the studies are being
imported into the tool, it assigns a score for each
study according to the occurrences of the keywords
defined in the protocol, in the studies title, abstract
and keywords list. This score can be used, for
example, to establish an order of reading once studies
with higher scores should be more relevant to the SR.
Also, if the studies with higher scores are not relevant
to the research question, it is possible that the strings
should be revisited and improved. The string
definition is an important point to the success of SRs,
and its quality can be accessed through visualization
provide by StArt, which is explored and presented in
Section 4.
The second step is Studies Selection (Step 6 of
Figure 1). In this step, the researcher should use the
inclusion and exclusion criteria, defined in the
protocol, to classify the studies as accepted or
rejected. Duplicated studies are automatically
identified by the tool. When the study is accepted, the
researcher can attribute to it a relevance level (Very
High, High, Low or Very Low).
The third step is Extraction (Steps 7, 8 and 9 of
Figure 2). At this step, the researcher must read the
full version of each study “Accepted study”, elaborate
a summary and fill in the Information Extraction
Form of each study (Figure 4-B).
Aiming to facilitate this step, it is possible to link
the studies full text file (e.g. PDF files) with their
record in the tool.
2.3 Summarization
In this phase (Step 10 of Figure 2), StArt provides
the following facilities:
Easy access to the information of all studies
accepted in Extraction Step. Comments and
information extracted in previous steps can be
accessed and copied to a text editor added in the
tool. After collecting that information, the
researcher can transfer this initial version of the
summary to a more powerful text editor.
Generation of charts that support a quantitative
SR characterization. For example: the percentage
of studies identified by each search engine, the
percentage of studies accepted, rejected and
duplicated in Extraction step, the times that each
inclusion and exclusion criterion was used for
classifying the studies as accepted or rejected
(Figure 11). In fact, this kind of quantitative data
is particularly relevant for Systematic Mappings
(Petersen et al, 2008). In case the researcher
choose to do meta-analysis, carry out statistical
tests or elaborate other charts, StArt can generate,
among other reports, a spreadsheet that allows
data manipulation outside the tool. These reports
can be generated according to researchers’ needs,
based on options that allow grouping data in
different ways, (Figure 5-A), applying different
filters (Figure 5-B) and choosing specific
characteristics of the studies (Figure 5-C). Figure
5-D shows a preview of the report.
Deal with a large volume of data to discover
features, patterns and hidden trends through
visualization. When an SR or SM process is
finished, there is a large amount of data related to
the research topic that can show trends in the
evolution of the topic over time, which is
interesting information to explain the state of the
art. As mentioned before, the information
visualization is a helpful tool for knowledge
integration activities.
3 VISUALIZATION IN StArt
Considering the importance of quantitative data for
both the SR and SM and the fact that information
visualization explores the natural visual ability of
humans aiming to facilitate information processing
(Gershon, Eick, Card, 1998), StArt uses
visualization to facilitate knowledge management
about literature reviews. Using effective visual
interfaces, it is possible to quickly manipulate large
volumes of data to discover characteristics, patterns
and hidden trends.
Based on visualization, for example, it is easier
to realize how a specific research topic evolved over
time. See Figure 6 where the researcher’s interest
was to understand how the topic “traceability” was
explored by the academic community, in relation to
the question investigated in this example. It is easy
to identify that in 2005 and 2006 there was only one
study published; in 2007 and 2008 there were few
additional studies, but in 2009, 2010 and 2011, the
number of studies that mentioned the research topic
was more significant than in the previous years.
To build this visualization, the researcher should
select the following options (Figure 6): green
rectangle representing an accepted study; part of the
study title nearby the rectangle, the publication year
as the grouping filter, and the Radial Graph as the
visualization technique.
Now, suppose that the researcher would like to
identify appropriated places for submitting a study
or for publishing results of a literature review. In this
ManagingLiteratureReviewsInformationthroughVisualization
39