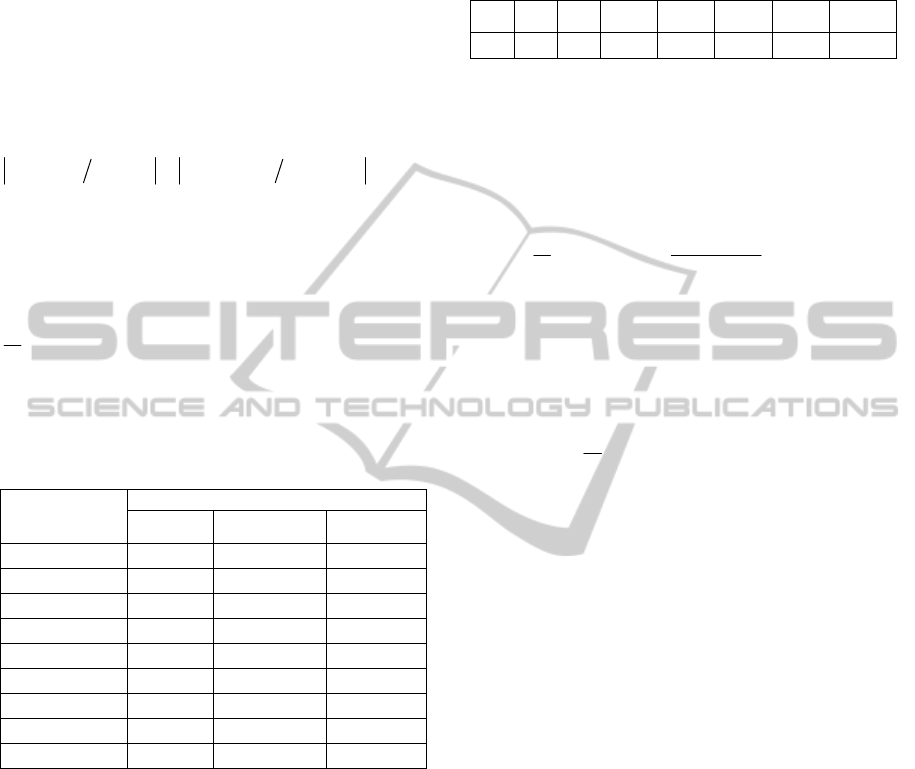
window position kp involves values of the input
signal x
k
as well as the DCT's coefficients computed
in two previous positions (k-1)p and (k-2)p of the
moving window. If p=1 the recursive equation is
simplified to one given in (4).
The number of arithmetic operations required for
computing the DCT with p>1 at a given window
position is evaluated as follows: if N is odd, the
complexity is N(p+1) multiplication operations and
N(p+1)+5(p-1) addition operations; if N is even,
from the property of symmetry of the functions
()()
)( )
sin sin sin sin
αα α α
−−
=
s s Ns Ns
mm
and
() ()
11,1,...1,
−
−=− = −
sNs
sN
1,...=mp, the
computational complexity with respect to
multiplication operations is reduced to N(p+3)/2.
The total number of required arithmetic operations
can be estimated as follows: 2N(p+1)+5(p-1) and
()()
355 1
2
++ −
N
pp
when N is odd and even,
respectively.
Table 1: Comparison of algorithms for computing running
DCT in terms of arithmetic operations flops (real adds and
mults), N=256.
p-step of running
window
Algorithms
Fast DCT Recursive DCT Proposed DCT
1 3708 1024 1024
2 3708 2048 1413
3 3708 3072 1802
4 3708 4096 2191
5 3708 5120 2580
6 3708 6144 2969
7 3708 7168 3358
8 3708 8192 3747
9 3708 9216 4136
The recursive running DCT algorithm (Rosendo
et al., 1998); (Kober and Cristobal, 1999) requires
approximately 4Np arithmetic operations for
computing the DCT spectra in equally spaced
window positions kp. One can observe that the
proposed algorithm with p>1 is more effective than
the use of the sliding recursive algorithm. Table 1
shows numerical results of computational
complexity for the proposed, fast DCT (Johnson and
Frigo, 2007); (Shao and Johnson, 2008), and running
recursive algorithms (Rosendo et al., 1998); (Kober
and Cristobal, 1999) for N=256 when p is varied.
We see that the proposed algorithm yields better
results when the window step p is less than a
boundary value of 8. The boundary values for the
step versus the window length N are provided in
Table 2.
Table 2: Boundary values of the step p for the proposed
algorithm versus N.
N 16 32 64 128 256 512 1024
p 3 4 6 7 8 9 10
The length of a moving window for the proposed
algorithm may be an arbitrary integer value
determined by characteristics of the signal to be
processed.
The inverse algorithms for the sliding DCTs can
be written as follows:
1
1
0
1
1/ 2
1
2cos
N
ks
s
kk
Ns
xX X
NN
π
−
=
+
=+
⎛⎞
⎛⎞
⎜⎟
⎜⎟
⎝⎠
⎝⎠
∑
,
(7)
where N=N
1
+N
2
+1. The computational complexity is
N multiplication operations and N addition
operations. If x
k
is the central pixel of the window,
that is, N
1
=N
2
and N=2N
1
+1, then the inverse
transform is given by
()
1
20
1
1
21
=
⎛⎞
=−+
⎜⎟
⎝⎠
∑
N
s
ks
s
kk
xXX
N
,
(8)
So, in the computation only the spectral coefficients
with even indices are involved. The computation
requires one multiplication operation and N
1
+1
addition operations.
3 CONCLUSIONS
A rapid recursive algorithm for computing the DCT
in a window running on a signal with an arbitrary
step was proposed. The algorithm is based on a
recursive relationship between three consequent
local DCT spectra which are computed in equally
spaced signal windows. The computational
complexity of the algorithm was compared with that
of common fast and running DCT algorithms.
Intervals for the window step in which the proposed
algorithm is more effective than the fast DCT
algorithms were calculated. The algorithm can be
used for local signal processing with an arbitrary
resolution in the short-time DCT domain.
REFERENCES
Jain, A. K., 1979. ‘A sinusoidal family of unitary
transforms’, IEEE Trans. Pattern Anal. Machine
Intell., vol. PAMI-1, pp. 356-365.
FastAlgorithmofShort-timeDCTforLowResolutionSignalProcessing
73