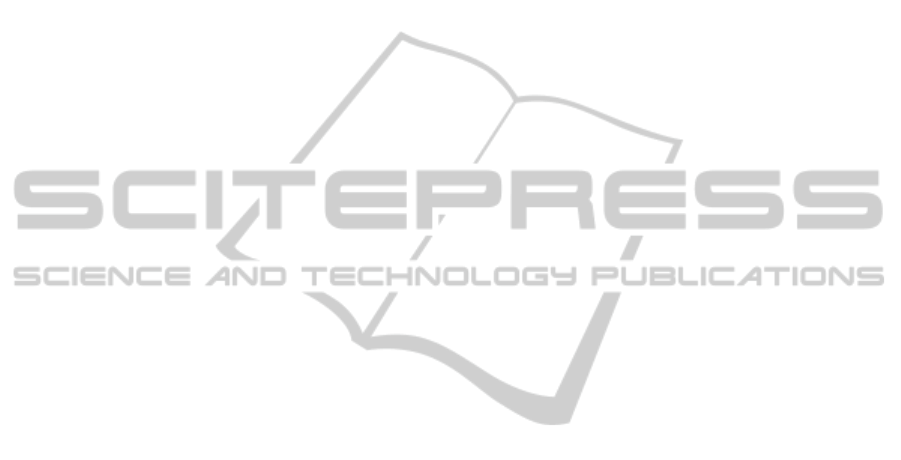
methodologies to generate more PSD programs.
ACKNOWLEDGEMENTS
This research is supported by Samsung Research
Fund (2010-0683-000) of Sungkyunkwan University,
Suwon, Republic of Korea.
REFERENCES
Chang, C. C. and Lin, C. J., 2011. LIBSVM: A library for
support vector machines. ACM Transactions on
Intelligent Systems and Technology, Vol. 2, No. 27, pp.
1-27, Software available at http://www.csie.ntu.edu.
tw/~cjlin/libsvm
Cristianini, N. and Shawe-Taylor, J., 2000.An Introduction
to Support Vector Machines. Cambridge University
Press.
Friedrichs, F. and Igel, C., 2005.Evolutionary tuning of
multiple SVM parameters, Neurocomputing, Vol.64,
pp.107–117.
Gagne, C., Schoenauer, M., Sebag, M. and Tomassini, M.,
2006. Genetic programming for kernel based learning
with co-evolving subsets selection, Proceedings of
Parallel Problem Solving in Nature, LNCS, No. 4193,
pp. 1006–1017.
Howley, T. and Madden, M. G., 2005. The genetic kernel
support vector machine: Description and evaluation,
Artif. Intell. Rev., Vol.24, No.3–4, pp.379–395.
Hsu, C. W., Chang, C. C. and Li, C. J., 2010, A Practical
Guide to Support Vector Classification, National
Taiwan University, Taiwan.
Huang, C. L. and Wang,C. J., 2006. A GA-based feature
selection and parameter optimization for support
vector machines, Expert Systems with Applications,
Vol. 31, No. 2, pp. 231–240.
Huang, C. L., Chen, M. C.and Wang, C. J., 2007. Credit
scoring with a data mining approach based on support
vector machines, Expert Systems with Applications,
Vol. 33, No. 4, pp. 847–856.
Kim, Y. K, 2011. Evolution Algorithms, Chonnam
National University Press, Republic of Korea.
Lessmann, S., Stahlbock, R. and Sven, F., 2006.Genetic
algorithms for support vector machine model selection,
Proceedings of International Joint Conference on
Neural Networks, pp. 3063–3069.
Methasate, I. and Theeramunkong, T., 2007.Kernel Trees
for Support Vector Machines, IEICE Trans. Inf. &
Syst., Vol. E90-D, No 10, pp. 1550–1556.
Mierswa, I., 2006. Evolutionary learning with kernels: A
generic solution for large margin problems,
Proceedings of the Genetic and Evolutionary
Computation Conference, pp. 1553–1560.
Phienthrakul, T. and Kijsirikul, B., 2005.Evolutionary
strategies for multiscale radial basis function kernels
in support vector machines, Proceedings of
Conference on Genetic and Evolutionary Computation,
pp.905–911.
Phienthrakul, T and Kijsirikul, B., 2008. Adaptive
stabilized multi-RBF kernel for support vector
regression, Proceedings of International Joint
Conference on Neural Networks, pp. 3545–3550.
Runarsson, T. P. and Sigurdsson, S., 2004.Asynchronous
parallel evolutionary model selection for support
vector machines,Neural Information Processing –
Letters and Reviews, Vol. 3, No. 3, pp. 59–67.
Keerthi, S., Sindhwani, V. and Chapelle, O., 2007.An
efficient method for gradient-based adaptation of
hyperparameters in SVM models, Advances in Neural
Information Processing Systems 19, MIT Press,
Cambridge, MA, pp. 674–480.
Simian, D., 2008.A model for a complex polynomial SVM
kernel, Proceeding of the 8th WSEAS International
Conference on Simulation, Modelling and
optimization, pp. 164–169
Simian, D. and Stoica, F., 2009.An evolutionary method
for constructing complex SVM kernels, Proceedings
of the 10th WSEAS International Conference on
Mathematics and Computers in Biology and
Chemistry, pp. 172–177.
Souza, B. F., Carvalho, A. C., R. Calvo and Ishii, R. P.,
2006.Multiclass SVM model selection using particle
swarm optimization, Proceedings of the Sixth
International Conference on Hybrid Intelligent
Systems, pp. 31–34.
Sullivan, K. and Luke, S., 2007. Evolving Kernels for
Support Vector Machine Classification, Proceedings
of the 9
th
annual conference on Genetic and
evolutionary computation, GECCO, pp. 1702–1707.
DATA2012-InternationalConferenceonDataTechnologiesandApplications
62