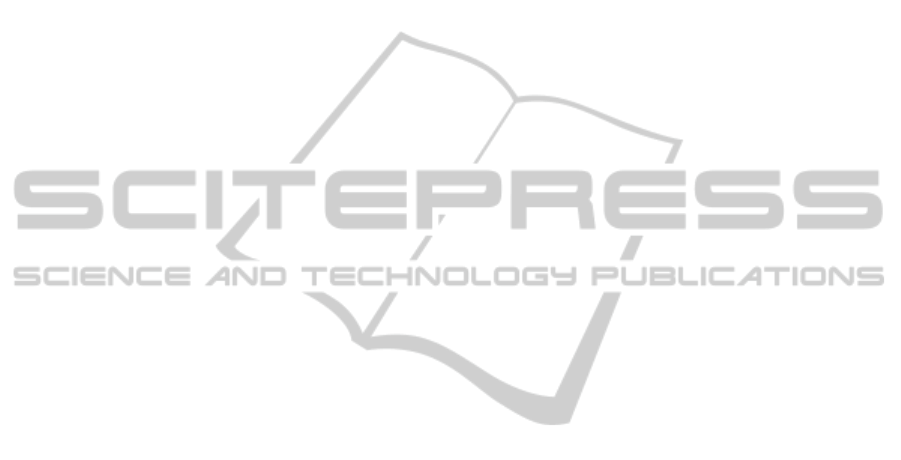
not profitable to operate the engine in this situation.
On Monday (10.17.2011), Tuesday (18.10.2011)
and Thursday (20.10.2011), the engine shuts down
before the end of the evening peak, which is due to
low load and thus the low efficiency of electricity gen-
eration. A similar situation occurred on Saturday and
Sunday (22.10.2011 and 23.10.2011), when the en-
gine did not run continuously during the peaks and
sometimes electricity was purchased from the grid.
The cost of electricity production (Fig. 6) for the
previously predicted load ranged from $0.13/kWh (in
peaks) to $0.27/kWh (in valleys). The cost of elec-
tricity at the optimum operating strategy (Fig. 6) dur-
ing both the peaks and the valleys could not be higher
than the cost specified in the tariff G12r of the compa-
nies “ENERGA-OBRÓT S.A.” and “Energa Operator
S.A”.
In order to compare the cost-effectiveness of the
proposed solution it should be compared with the
single-zone electricity tariff by subtracting from each
the sum of both the variable and fixed costs for the
considered time period. As a reference point the
G11 tariff was assumed, relating to the power com-
panies “ENERGA-OBRÓT S.A.” and “Energa Oper-
ator S.A” (fixed cost – $3.49/month gross and variable
cost – $0.19/kWh gross).
For the considered week the difference in variable
costs was $287/week. After taking into account the
fixed costs, the income associated with the proposed
solution was $278/week.
4 CONCLUSIONS
The neural network used to predict the load was pro-
posed and the control strategy for the NG piston en-
gine as a DG source of power is presented. From
the investigations performed, it was determined that
the most appropriate objective function of the strat-
egy is to operate the engine for maximum profit (de-
fined as avoided costs of buying electricity from the
grid). On average, the NG piston engine is started up
two times a day: during both the morning and evening
peak loads.
Profits from operation of the NG piston engine de-
pend strictly on the load profile and for the case at
hand it was $278/week.
Currently, many buildings (e.g. office buildings)
have piston engines as emergency power units, but
mainly fueled by liquid fuels (gasoline, oil) – which
are more expensive than NG. Those units are not used
for power generation. If as expected there is further
inflation in electricity prices, power units might be
considered for power generation exclusively during
peak loads. In those cases, investment (installation)
costs are incurred, but in the case of large buildings
(with a range of MW), the profits could be quite sub-
stantial.
ACKNOWLEDGEMENTS
Scientific work financed from funds for science in
2010–2012 as a research project
REFERENCES
Adam, N. B., Elahee, M., and Dauhoo, M. (2011). Forecast-
ing of peak electricity demand in mauritius using the
non-homogeneous gompertz diffusion process. En-
ergy, 36:6763–6769.
Al-Sulaiman, F., Dincer, I., and Hamdullahpur, F. (2010).
Energy analysis of a trigeneration plant based on solid
oxide fuel cell and organic rankine cycle. Inter-
national Journal of Hydrogen Energy, 35(10):5104–
5113.
Amjady, N. and Keynia, F. (2009). Short-term load fore-
casting of power systems by combination of wavelet
transform and neuro-evolutionary algorithm. Energy,
34:46–57.
Azadeh, A., Ghaderi, S., and Sohrabkhani, S. (2008). A
simulated-based neural network algorithm for fore-
casting electrical energy consumption in iran. Energy
Policy, 36:2637–2644.
Azadeh, A., Ghaderi, S., Tarverdian, S., and Saberi, M.
(2007). Integration of artificial neural networks and
genetic algorithm to predict electrical energy con-
sumption. Applied Mathematics and Computation,
186:1731–1741.
Beccali, M., Cellura, M., Brano, V. L., and Marvuglia, A.
(2004). Forecasting daily urban electric load profiles
using artificial neural networks. Energy Conversion
and Management, 45:2879–2900.
Budzianowski, W. (2011). Opportunities for bioenergy
in poland: Biogas and solid biomass fuelled power
plants. Rynek Energii, 94(3):138–146. cited By (since
1996) 1.
Cai, Y., zhou Wang, J., Tang, Y., and chen Yang, Y. (2011).
An efficient approach for electric load forecasting us-
ing distributed art (adaptive resonance theory) & hs-
artmap (hyper-spherical artmap network) neural net-
work. Energy, 36:1340–1350.
Chaichana, K., Patcharavorachot, Y., Chutichai, B., Sae-
bea, D., Assabumrungrat, S., and Arpornwichanop, A.
(2012). Neural network hybrid model of a direct in-
ternal reforming solid oxide fuel cell. International
Journal of Hydrogen Energy, 37(3):2498–2508.
Corria, M. E., Cobas, V. M., and Lora, E. S. (2006). Per-
spectives of stirling engines use for distributed gener-
ation in brazil. Energy Policy, 34:3402–3408.
Demuth, H., Beale, M., and Hagan, M. Neural network
toolbox
TM
6 user’s guide matlab
R
. Technical report.
OptimalControlStrategyofNGPistonEngineasaDGUnitObtainedbyanUtilizationofArtificialNeuralNetwork
175