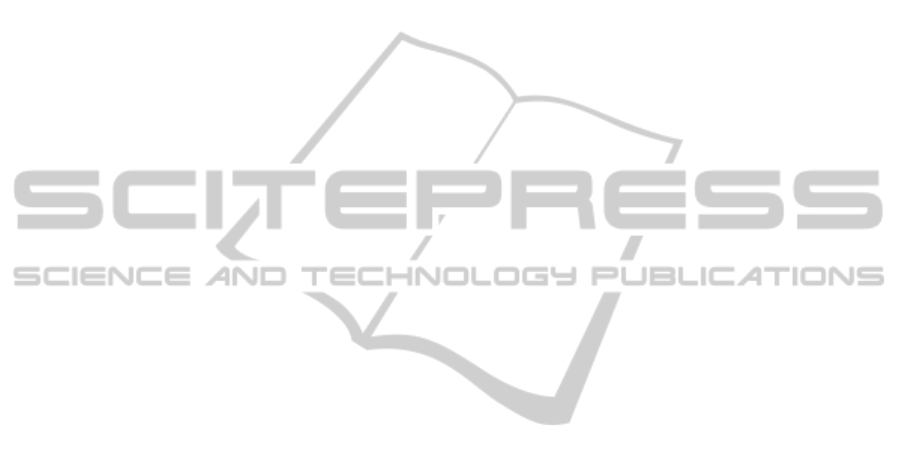
Chang, Chih-Chung and Lin, Chih-Jen (2011). Libsvm: A
library for support vector machines. In ACM Transac-
tions on Intelligent Systems and Technology. Software
available at http://www.csie.ntu.edu.tw/ cjlin/libsvm.
Chiang, C. J. and Chen, C. L. (2010). Constrained control
of homogeneous charge compression ignition (hcci)
engines. In 5th IEEE Conference on Industrial Elec-
tronics and Applications (ICIEA).
Chitralekha, S. B., Shah S. L. (2010). Application of sup-
port vector regression for developing soft sensors for
nonlinear processes. In The Canadian Journal of
Chemical Engineering. Wiley.
Christensen, M., Einewall P. and Johansson, B. (1997). Ho-
mogeneous charge compression ignition (hcci) using
iso-octane, ethanol and natural gas- a comparison to
spark ignition operation. In SAE Technical Paper
972874.
Clarke, S. M., Griebsch J. H. and Simpson, T. W. (2005).
Analysis of support vector regression for approxima-
tion of complex engineering analyses. In Journal of
Mechanical Design.
Drezet, P. M. L. and Harrison, R. F. (1998). Support vector
machines for system identification. In UKACC Inter-
national Conference on Control.
Drucker, H., Burges C. J. C. Kaufman L. Smola A. Vap-
nik V. (1996). Support vector regression machines.
Gretton, A., Douce A. Herbrich R. Rayner P. J. W. and
Scholkop, B. (2001). Support vector regression for
black-box system identification. In Proceedings of the
11th IEEE Signal Processing Workshop on Statistical
Signal Processing.
Hammer, B. and Gersmann, K. (2003). A note on the uni-
versal approximation capability of support vector ma-
chines. In Neural Processing Letters. Kluwer Aca-
demic Publishers.
Hastie, Tibshirani and Friedman (1995). The Elements
of Statistical Learning: Data Mining, Inference, and
Prediction. Springer, 2 edition.
Kim, K. J. (2003). Financial time series forecasting using
support vector machines. In Neurocomputing. Else-
vier.
Kruse, T., Kurz S. and Lang, T. (2010). Modern statistical
modelling and evolutionary optimisation methods for
the broad use in ecu calibration. In 6th IFAC Sympo-
sium Advances in Automotive Control.
Mller, K. R., Smola A. J. Ratsch G. Scholkopf B. Kohlmor-
gen J. and Vapnik, V. (1997). Predicting time series
with support vector machines. In Artificial Neural
Networks ICANN’97. Springer Berlin / Heidelberg.
Ramon, M. M., Alvarez J. L. R. Valls G. C. Mari J. M.
Vazquez A. N. Olivas E. S. Vidal A. R. F. (2006). Sup-
port vector machines for nonlinear kernel arma system
identification. In IEEE Transactions on Neural Net-
works.
Ravi, N., Roelle M. J. Liao H. H. Jungkunz A. F. Chang
C. F. Park S. and Gerdes, J. C. (2009). Model-based
control of hcci engines using exhaust recompression.
In IEEE Transactions on Control Systems Technology.
Schlkopf, B., Bartlett P. Smola A. and Williamson, R.
(1998). Support vector regression with automatic ac-
curacy control. In Proceedings of ICANN’98, Perspec-
tives in Neural Computing.
Schlkopf, B., Smola A. J. Williamson R. C. and Bartlett,
P. L. (2000). New support vector algorithms. In Neu-
ral Computation. MIT Press.
Scholkopf, B. and Smola, A. J. (2001). Learning with Ker-
nels: Support Vector Machines, Regularization, Opti-
mization, and Beyond. MIT Press, Cambridge, MA,
USA.
Smola, A. J. and Schlkopf, B. (2003). A tutorial on sup-
port vector regression. Technical report, Statistics and
Computing.
Sun, J., Zhou Y. Bai Y. and Luo, J. (2006). Nonlin-
ear noise reduction of chaotic time series based on
multi-dimensional recurrent least squares support vec-
tor machines. In Neural Information Processing, Lec-
ture Notes in Computer Science. Springer Berlin / Hei-
delberg.
Thring, R. (1989). Homogeneous-charge compression-
ignition (hcci) engines. In SAE Technical Paper
892068.
Trejo, J. A. R., Yu W. Li X. (2006). Support vector ma-
chine for nonlinear system on-line identification. In
2006 3rd International Conference on Electrical and
Electronics Engineering.
Vapnik, V., Golowich S. E. and Smola, A. (1996). Support
vector method for function approximation, regression
estimation, and signal processing. In Advances in
Neural Information Processing Systems.
Vapnik, V. (1995). The Nature of Statistical Learning The-
ory. Springer, New York.
Wang, X., Du Z. Chen J. and Pan, F. (2009). Dynamic mod-
eling of biotechnical process based on online support
vector machine. In Journal of Computers. Academy
Publisher.
SupportVectorMachinesforIdentificationofHCCICombustionDynamics
393