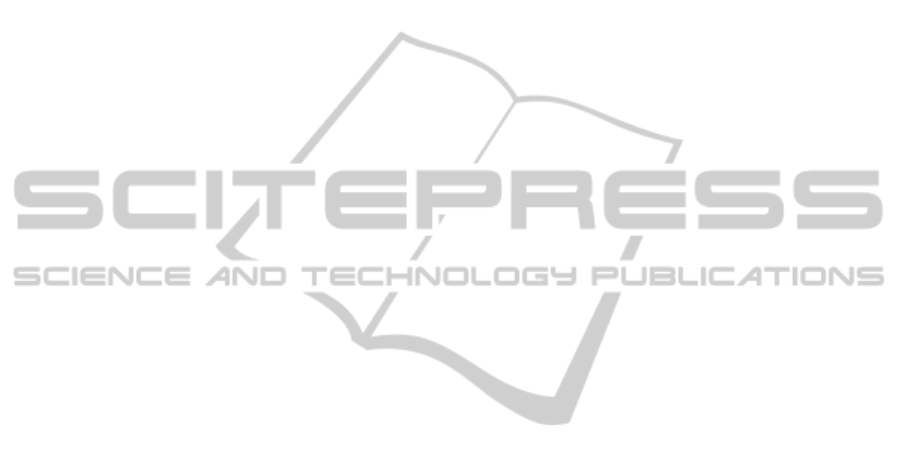
12 systems which represented the following classes
of non-stiff problems: linear/nonlinear, in each of
them simple/moderate/complex, in each of them
with/without uncertainty. This classification makes
sense since there exist theoretical results about dif-
ferences in performance of solvers for linear and non-
linear problems. The presence of uncertainty and the
dimension of the problem (which is one of the fac-
tors defining the numeric complexity) seem to play
an important role. These claims can be corroborated
empirically if the problem database is big enough.
As of now, there are over 45 examples in our
database, mainly simple and moderate systems in
both linear and nonlinear classes. In Figure 1, we
show work-precision diagrams (WPD) for three avail-
able solvers (RIOT on the left, VALENCIA-IVP in
the middle, VNODE-LP on the right) and three sets
of parameters for each. We tested the solvers on all
nonlinear problems from the classes simple/with un-
certainty (top), simple/without uncertainty (middle),
moderate/without uncertainty (bottom). For more
information about the used problems (given by the
number in the figure) and the conditions of the test,
visit
vericomp.inf.uni-due.de
. The parameter
sets we vary for the three tools are different in their
nature. Therefore, the CPU time always increases
from parameter set one to parameter set three for
VALENCIA-IVP (the only solver without an auto-
matic step size control) since we decrease the step-
size, which is not always true for the other two solvers
where we modify the order of the Taylor expansion.
From the figure, we observe that all three solvers
perform differently for problems with and without un-
certainty (the top and middle WPDs). Whereas VN-
ODE is best with respect to CPU times in both cases,
it produces considerably less tight enclosures than
RIOT for uncertain problems. For the same exam-
ples without uncertainty, the quality of enclosures of
these two solvers is almost equal. There is also a dif-
ference between the classes of simple and moderate
problems: for example, RIOT with the third set of
settings is mostly better with respect to the enclosure
width for the bottom figure, which does not hold for
the middle one. The figures show that each class gen-
erates a distinct solver behavior, giving us a basis for
employing the classification as the similarity measure
while recommending a solver for a specific example.
4 CONCLUSIONS
We presented process-oriented guidelines for verifi-
cation of biomechanical problems. In the scope of
software quality analysis, we described an online sys-
tem for comparison of verified initial value problem
solvers. This provides broader user support in the
area of numerical verification with (extended) interval
methods, helping to raise awareness of verified tools
in engineering.
REFERENCES
AIAA (1998). Guide for the Verification and Validation of
Computational Fluid Dynamics Simulations. Ameri-
can Institute of Aeronautics and Astronautics.
ASME (2006). Guide for Verification and Validation in
Computational Solid Mechanics. American Society of
Mechanical Engineers, pages 1–15.
Auer, E., Chuev, A., Cuypers, R., Kiel, S., and Luther, W.
(2011). Relevance of Accurate and Verified Numerical
Algorithms for Verification and Validation in Biome-
chanics. In EUROMECH Colloquium 511, Ponta Del-
gada, Azores, Portugal.
Auer, E. and Luther, W. (2009). Numerical Verification As-
sessment in Computational Biomechanics. In Proc. of
Dagstuhl Seminar 08021, LNCS, pages 145–160.
Auer, E., Luther, W., and Cuypers, R. (2012). Process-
Oriented Verification in Biomechanics: A Case Study.
In Proc. of SUM 2012, Marburg, Germany. Submitted.
Auer, E. and Rauh, A. (2012). VERICOMP: A System to
Compare and Assess Verified IVP Solvers. Comput-
ing, 94(2):163–172.
Berz, M. (1995). Modern Map Methods for Charged Par-
ticle Optics. Nuclear Instruments and Methods A363,
pages 100–104.
de Figueiredo, L. H. and Stolfi, J. (2004). Affine Arith-
metic: Concepts and Applications. Numerical Algo-
rithms, 34(1-4):147–158.
Ferson, S., Kreinovich, V., Ginzburg, L., Myers, D. S.,
and Sentz, K. (2003). Constructing Probability Boxes
and Dempster-Shafer Structures. Technical Report
SAND2002-4015, Sandia National Laboratories.
Henninger, H. and Reese, S. (2010). Validation of Compu-
tational Models in Biomechanics. In Proc. of the In-
stitution of Mechanical Engineers, volume 224, pages
801–812.
Hull, T. E., Enright, W. H., Fellen, B. M., and Sedgwick,
A. E. (1972). Comparing Numerical Methods for Or-
dinary Differential Equations. SIAM Journal on Nu-
merical Analysis, 9(4):603–637.
Mazzia, F. and Iavernaro, F. (2003). Test Set for Initial
Value Problem Solvers. Technical Report 40, Depart-
ment of Mathematics, University of Bari, Italy.
Moore, R. E., Kearfott, R. B., and Cloud, M. J. (2009). In-
troduction to Interval Analysis. Society for Industrial
and Applied Mathematics, Philadelphia.
Oberkampf, W. and Trucano, T. (2007). Verification and
Validation Benchmarks. Nuclear Engineering and
Design, 238(3):716–743.
Schlesinger, S. (1979). Terminology for Model Credibility.
Simulation, 32(3):103–104.
ICINCO2012-9thInternationalConferenceonInformaticsinControl,AutomationandRobotics
518