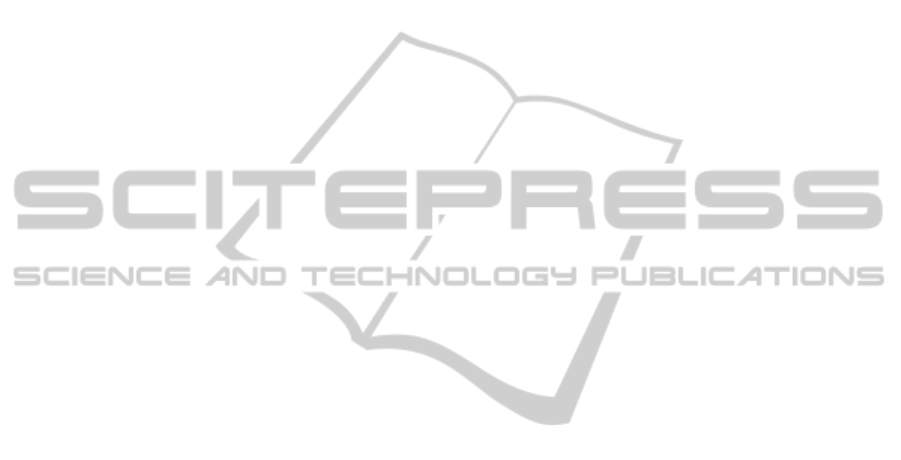
The purpose of our algorithm is to reduce the
incorrect matches rate and improve the accuracy of
the registration phase. Our scenes contain some
moving objects but the transformation has to
estimate the motion of the image background. The
existing methods of feature matching try to reject
outliers. But, in our case, we need to reject false
positives matches which are detected on the moving
objects. This can biases the estimation of the
background motion. So we introduce a geometric
criterion to eliminate false positive and false
negative matches which are respectively: incorrect
matched points and correct matched points attached
to moving objects.
The paper is divided as follows: in section 2 we
have an overview of global and local image
registration techniques. In section 3 we present a
formulation of the registration problem. A
description of the traditional image registration
algorithm and our proposed amelioration which adds
geometric criterion are explained in section 4.
Section 5 presents some experimental results.
2 PREVIOUS WORKS
Image registration can be approached with global or
local method. The global one consists in optimizing
a certain criterion until obtaining a geometric
transformation which fits correctly the two
registered frames. Used criterion is usually the sum
of squared difference (SSD) of the whole image
luminance, the correlation or the mutual
informationetc. (Whawahre et al., 2009). These
methods need textured surface and are very time
consuming since they work on the total number of
pixels of the image. They can also be sensitive to the
luminosity change of the image (SSD), can not
handle a very large rotation, translation or scale
changes and can easily fall into a local minimum.
Local methods are usually divided into four
steps: feature detection, feature matching,
transformation function estimation and image re-
sampling. A review of image registration approaches
can be find in (Zitova and Flusser, 2003; Xiong and
Zhang, 2009a; Xiong and Zhang, 2010). Features
can be edges, corners, lines, regions or a
combination between them. These methods are less
consuming time as they work with some relevant
and reliable part of the image. Many features points
have been proposed and improved all over the time:
Moravec, Harris and Stephens, Trajkovic, SUSAN
detector.
Every time the detectors try to be less sensitive
to noise, invariant to affine transformation and
rotation or scale changes. Laplacian of Gaussian and
Difference of Gaussian are invariant scale blobs
detector, on which, is based the most known and
robust features detector: SIFT (Scale invariant
feature transform) (Lowe, 2004). Govender, 2009
showed that SIFT is one of the best distinctive
detector. The requirements of a feature detector are:
Every ected; No false alarm
must be detected; Points must be well localized,
Detector must have a high repeatability rate (stable
between different images); Detector must be
insensitive to noise, Invariant to rotation and scale
changes and finally; Detector must have a reduced
algorithmic complexity for the real time application.
Once the features are detected, the next step is
feature matching. Matching can be done using a
similarity criterion between two window centred on
the feature point like SSD, NCC (normalized
co But this
window is only adapted for distortion caused by a
translation. Those similarity criterion can also be
sensitive to noise and illumination change, as well
that they need textured regions.
Invariant descriptors are well adapted to describe a
feature point. Schmid, 1992 proposed an eight
components descriptor based on different derivatives
order of the luminance function. Lowe proposed, in
addition to the features detector SIFT, a 128 elements
descriptor estimated from gradient oriented
histograms. Many other variant of the SIFT
descriptors has been also proposed: RIFT (Lazebnik
et al., 2004), PCA-SIFT (Ke and Sukthankar, 2004),
GLOH (Mikolajczyk and Schmid, 2005), GRIFT
(Sungho et al., 2006) and SURF (Bay et al., 2006).
These variants have increased its robustness,
distinctiveness and even reduced its descriptor size
(PCA-Sift and GLOH). Mikolajczyk and Schmid,
2005 compared some local descriptors and proved
that SIFT and GLOH present the highest matching
accuracy.
Feature descriptor must be: Invariant: the same
point in different frame must have the same
descriptor; Unique: two different points must have
two different descriptors; Stable: the descriptor of
the same primitive but with some scale or rotation
change must be the same as the original one;
Independent: if the descriptor is a vector, its
elements have to be independent (generally not
feasible).
False Positive Outliers Rejection for Improving Image Registration Accuracy - Application to Road Traffic Aerial
Sequences
275