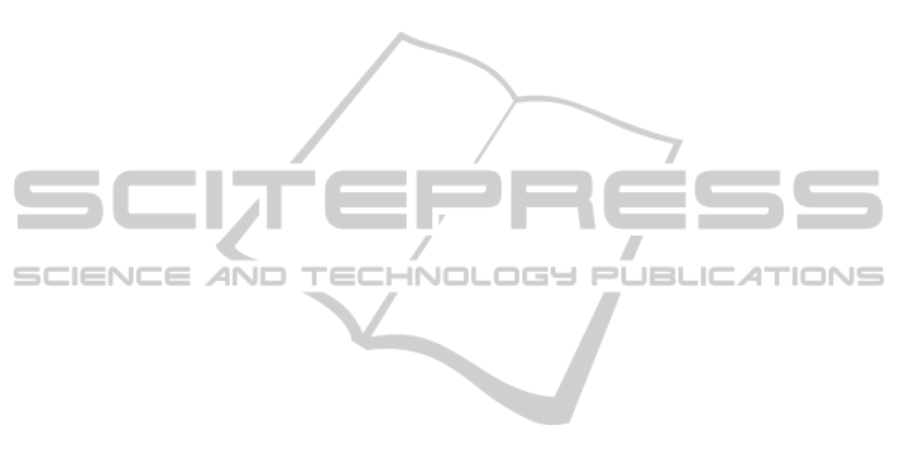
5 CONCLUSIONS
In this work, we proposed document
recommendation methods based on hybrids of
personal and group trust models. Such hybrid
models are used to compute users’ trust values from
the personal and group perspectives in order to
discover reliable and trustworthy users in the
recommendation process. In considering these two
perspectives, three trust models are proposed,
namely the hybrid personal trust (HPT), item-level
group trust (IGT), and a hybrid of HPT and IGT
(HPT-IGT). From the personal perspective, HPT
adaptively not only takes users’ ratings on co-rated
documents, but also the role relationship trust into
account in trust computation. From the group
perspective, IGT derives the trust value of a target
user’s group on a recommender by using users’ role
weights to aggregate the opinions of the target user’s
group members on a specific item.
Moreover, to take advantage of the merits of both
HPT and IGT models, we also propose a hybrid of
HPT and IGT (HPT-IGT) models in order to obtain
trust values by considering both the personal and
group aspects. A target user usually has preferences
similar to his group members’, such that a
recommender trusted by his group members may
also be trusted by the user. The experiment result
shows that the trust value of IGT can indeed
complement the trustworthiness of personal
perspective. Additionally, the prediction accuracy of
recommendation is indeed improved using the HPT,
IGT, and HPT-IGT models. Our proposed methods
not only intensify the prediction accuracy of trust,
but also offer better improvement of
recommendation quality than other trust-based CF
methods.
ACKNOWLEDGEMENTS
This research was partially supported by the
National Science Council of the Taiwan under the
grant NSC 100-2410-H-033-037.
REFERENCES
Cho, J., Kwon, K. & Park, Y. 2007. Collaborative filtering
using dual information sources.
IEEE Intelligent
Systems,
22, 30-38.
Hwang, C.-S. & Chen, Y.-P. 2007. Using trust in
collaborative filtering recommendation. In: OKUNO,
H. & Ali, M. (eds.)
New Trends in Applied Artificial
Intelligence.
Springer Berlin / Heidelberg.
Jain, A. K., Murty, M. N. & Flynn, P. J., 1999. Data
clustering: a review. ACM Computing Surveys (CSUR),
31, 264-323.
Kim, D. J., Ferrin, D. L. & Rao, H. R., 2008. A trust-based
consumer decision-making model in electronic
commerce: The role of trust, perceived risk, and their
antecedents.
Decision Support Systems, 44, 544-564.
Konstan, J. A., Miller, B. N., Maltz, D., Herlocker, J. L.,
Gordon, L. R. & Riedl, J., 1997. GroupLens: Applying
collaborative filtering to Usenet news.
Communications of the ACM, 40, 77-87.
Lathia, N., Hailes, S. & Capra, L., 2008. Trust-based
collaborative filtering. In: Karabulut, Y., Mitchell, J.,
Herrmann, P. & Jensen, C. (eds.)
Trust Management II.
Springer Boston.
Liu, D.-R., Lai, C.-H. & Chiu, H., 2011. Sequence-based
trust in collaborative filtering for document
recommendation. International Journal of Human-
Computer Studies,
69, 587-601.
Massa, P. & Avesani, P., 2004. Trust-aware collaborative
filtering for recommender systems. On the Move to
Meaningful Internet Systems: CoopIS, DOA, and
ODBASE.
Springer Berlin / Heidelberg.
Massa, P. & Avesani, P., 2007a. Trust-aware
recommender systems. Proceedings of the ACM
Conference on Recommender Systems.
Minneapolis,
MN, USA: ACM.
Massa, P. & Avesani, P., 2007b. Trust metrics on
controversial users: Balancing between tyranny of the
majority. International Journal on Semantic Web &
Information Systems,
3, 39-64.
Massa, P. & Bhattacharjee, B., 2004. Using trust in
recommender systems: An experimental analysis. In:
JENSEN, C., POSLAD, S. & DIMITRAKOS, T. (eds.)
Trust Management. Springer Berlin / Heidelberg.
O'donovan, J. & Smyth, B., 2005. Trust in recommender
systems.
Proceedings of the 10th International
Conference on Intelligent User Interfaces.
San Diego,
California, USA: ACM.
Resnick, P., Iacovou, N., Suchak, M., Bergstrom, P. &
Riedl, J., 1994. GroupLens: an open architecture for
collaborative filtering of netnews.
Proceedings of the
ACM Conference on Computer Supported Cooperative
Work.
Chapel Hill, North Carolina, United States:
ACM.
Riggs, T. & Wilensky, R., 2001. An algorithm for
automated rating of reviewers.
Proceedings of the 1st
ACM/IEEE-CS Joint Conference on Digital Libraries.
Roanoke, Virginia, United States: ACM.
Salton, G. & Buckley, C., 1988. Term weighting
approaches in automatic text retrieval.
Information
Processing and Management,
24, 513-523.
Schafer, J., Frankowski, D., Herlocker, J. & Sen, S. 2007.
Collaborative filtering recommender systems. In:
Brusilovsky, P., Kobsa, A. & Nejdl, W. (eds.)
The
Adaptive Web.
Springer Berlin / Heidelberg.
DATA2012-InternationalConferenceonDataTechnologiesandApplications
38