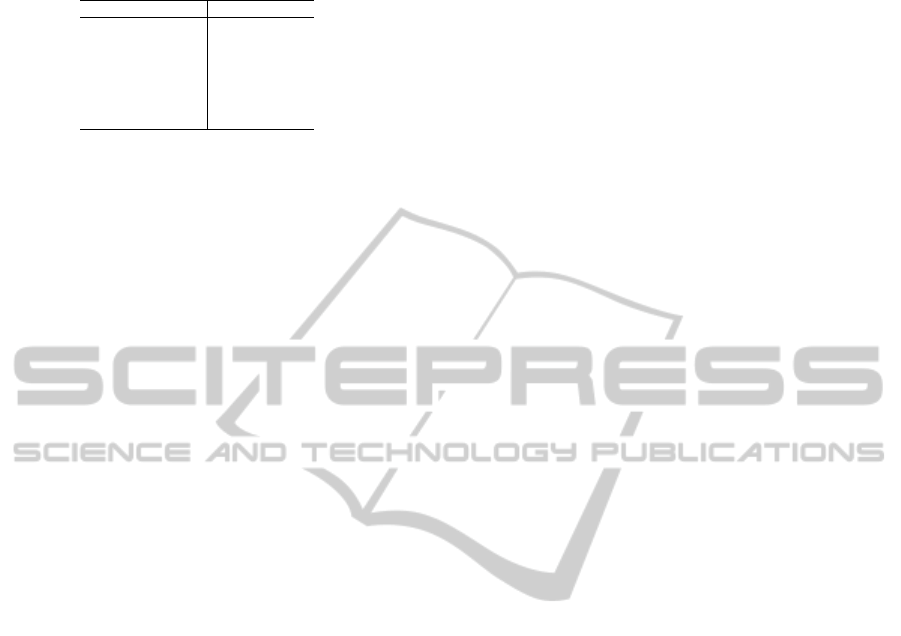
Table 2: Evaluation of the hybrid visualization and anomaly
detection approach.
hybrid approach
high dimensionality +
time +
continuous data +
discrete data +
hybrid data +
loss of information +
cyclic processes +
may be unconsidered by choosing the first two princi-
pal components only.
The approach was also evaluated in order to de-
tect anomalies. In most cases the anomalous behavior
could be detected and the anomalous module and sig-
nal could be determined correctly.
A minor disadvantage of the proposed approach
is that still some expert knowledge is needed to ana-
lyze the plant’s behavior in detail. Nonetheless it is
possible to detect anomalies and the anomalous pro-
duction module(s) and signal(s) without any expert
knowledge.
Another disadvantage is that the proposed ap-
proach works well for the cyclic process, but not for
extended production plants which deal with different
variants of products. This will be improved in future
work.
6 CONCLUSIONS
In this paper a visual analytics approach to the vi-
sualization of technical processes is presented. The
discrete state encoding gives a neat overview of the
observed process and shows the main process states
over the time line. The principal component analy-
sis gives a more abstract overview of the process and
additionally includes continuous data. Both methods
were connected to combine their advantages.
Further it was shown how the visualization and
anomaly detection approach can be used to analyze
a technical process. In three steps the operator is
guided through the observation of the current behav-
ior and the corresponding reference behavior. This
side-by-side visualization enables to detect an occur-
ring anomaly. In the further steps (by zooming into
the process) the operator is guided to the anomalous
module and finally to the anomalous signal.
In further work some other visualization ap-
proaches will be explored. These shall show the most
relevant data in a more intuitive way to give the pos-
sibility to analyze the process behavior without (or at
least with less) expert knowledge. To face the dis-
advantage of the DSE, continuous values can be dis-
cretized using an n-bit-discretization. This will also
be considered in future work.
Furthermore, the visualized reference process will
consider more than only one reference process. This
will provide a more generalized view on the plant’s
behavior.
REFERENCES
Alfred, I. (1985). The plane with parallel coordinates. The
Visual Computer, 1:69–91.
Bronstein, M. M., Bronstein, A. M., Kimmel, R., and
Yavneh, I. (2006). Multigrid multidimensional scal-
ing. Numerical Linear Algebra with Applications,
13(2-3):149–171.
Cleveland, W. (1993). Visualizing Data. AT&T Bell Labo-
ratories.
Frey, C. W. (2008). Diagnosis and monitoring of complex
industrial processes based on self-organizing maps
and watershed transformations. In IEEE International
Conference on Computational Intelligence for Mea-
surement Systems and Applications.
Huang, M. L., Liang, J., and Nguyen, Q. V. (2009). A vi-
sualization approach for frauds detection in financial
market. In Proceedings of the 2009 13th International
Conference Information Visualisation, IV ’09, pages
197–202, Washington, DC, USA. IEEE Computer So-
ciety.
Jolliffe, I. T. (2002). Principal Component Analysis.
Springer.
Keim, D. A. (2002). Information visualization and visual
data mining. IEEE Transactions on Visualization and
Computer Graphics, 8(1):1–8.
Keim, D. A., Kohlhammer, J., Ellis, G., and Mansmann, F.,
editors (2010). Mastering The Information Age - Solv-
ing Problems with Visual Analytics. Eurographics.
Niggemann, O., Stein, B., Voden
ˇ
carevi
´
c, A., Maier, A., and
Kleine B
¨
uning, H. (2012). Learning behavior models
for hybrid timed systems. In Twenty-Sixth Conference
on Artificial Intelligence (AAAI-12), Toronto, Ontario,
Canada.
Shneiderman, B. (1996). The eyes have it: A task by data
type taxonomy for information visualizations. IEEE
Symposium on Visual Languages, page 336.
Thomas, J. J. and Cook, K. A. (2006). A visual analytics
agenda. IEEE Computer Graphics and Applications,
26:10–13.
Tufte, E. (2001). The visual display of quantitative infor-
mation. Graphics Press.
Tufte, E. R. (1997). Visual Explanations: Images and
Quantities, Evidence and Narrative. Graphics Press
LLC.
VisualAnomalyDetectioninProductionPlants
75