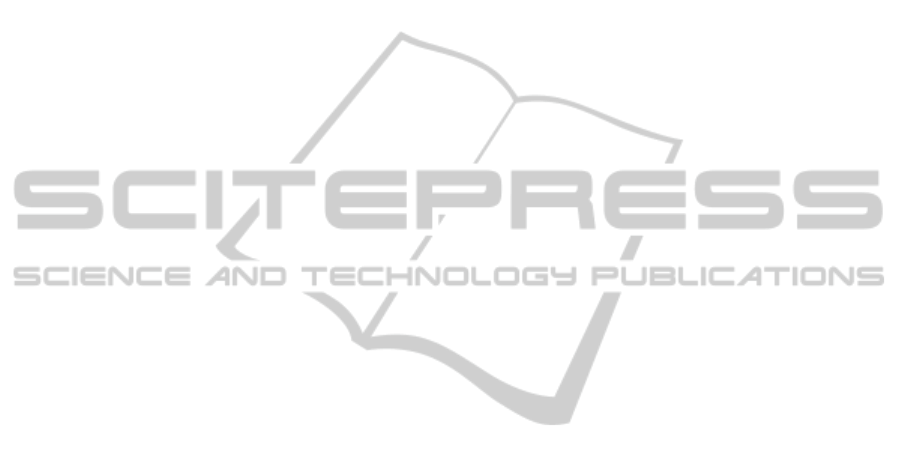
to investigate the issues arising when the methodol-
ogy proposed in this paper have to deal with indica-
tors and scenarios requiring an estimation of several
indicators to address inconsistencies (including possi-
ble complexity issues). We are also considering how
to exploit statistical information about the consistent
part of a dataset to narrow the bounds of the estimated
indicator. The dataset that has been described in this
paper cannot be shared due to privacy related issues.
We are working on building a fictitious dataset that
can be used as a testbed for comparing formal meth-
ods with other approaches (e.g. learning based meth-
ods) in the context of inconsistency detection and res-
olution.
ACKNOWLEDGEMENTS
The authors would like to thank the anonymous re-
viewers for their valuable comments and suggestions.
REFERENCES
Arasu, A. and Kaushik, R. (2009). A grammar-based en-
tity representation framework for data cleaning. In
Proceedings of the 35th SIGMOD international con-
ference on Management of data, pages 233–244.
Arenas, M., Bertossi, L., Chomicki, J., He, X., Raghavan,
V., and Spinrad, J. (2003). Scalar aggregation in in-
consistent databases. Theoretical Computer Science,
296(3):405–434.
Arenas, M., Bertossi, L. E., and Chomicki, J. (1999). Con-
sistent query answers in inconsistent databases. In
ACM Symp. on Principles of Database Systems, pages
68–79. ACM Press.
Batini, C., Cappiello, C., Francalanci, C., and Maurino, A.
(2009). Methodologies for Data Quality Assessment
and Improvement. ACM Comput. Surv., 41:16:1–
16:52.
Batini, C. and Scannapieco, M. (2006). Data Quality: Con-
cepts, Methodologies and Techniques. Data-Centric
Systems and Applications. Springer.
Chomicki, J. and Marcinkowski, J. (2005). Minimal-change
integrity maintenance using tuple deletions. Informa-
tion and Computation, 197(1-2):90–121.
Csiszar, I. and K¨orner, J. (1981). Information theory: cod-
ing theorems for discrete memoryless systems, volume
244. Academic press.
Embury, S., Brandt, S., Robinson, J., Sutherland, I., Bisby,
F., Gray, W., Jones, A., and White, R. (2001). Adapt-
ing integrity enforcement techniques for data recon-
ciliation. Information Systems, 26(8):657–689.
Fan, W., Geerts, F., and Jia, X. (2008). A Revival of In-
tegrity Constraints for Data Cleaning. Proc. VLDB
Endow., 1:1522–1523.
Fellegi, I. and Holt, D. (1976). A systematic approach to au-
tomatic edit and inputation. Journal of the American
Statistical association, 71(353):17–35.
Galhardas, H., Florescuand, D., Simon, E., and Shasha, D.
(2000). An extensible framework for data cleaning.
In Proceedings of ICDE ’00, pages 312–. IEEE Com-
puter Society.
Iosifescu, M. (1980). Finite Markov processes and their
applications. Wiley.
Maletic, J. and Marcus, A. (2000). Data cleansing: beyond
Integrity Analysis. In Proceedings of the Conference
on Information Quality, pages 200–209.
Martini, M. and Mezzanzanica, M. (2009). The Federal Ob-
servatory of the Labour Market in Lombardy: Models
and Methods for the Costruction of a Statistical In-
formation System for Data Analysis. In Larsen, C.,
Mevius, M., Kipper, J., and Schmid, A., editors, Infor-
mation Systems for Regional Labour Market Monitor-
ing - State of the Art and Prospectives. Rainer Hampp
Verlag.
Mayfield, C., Neville, J., and Prabhakar, S. (2009). A Sta-
tistical Method for Integrated Data Cleaning and Im-
putation. Technical Report CSD TR-09-008, Purdue
University.
Mezzanzanica, M., Boselli, R., Cesarini, M., and Merco-
rio, F. (2011). Data quality through model checking
techniques. In Gama, J., Bradley, E., and Hollm´en, J.,
editors, IDA, volume 7014 of Lecture Notes in Com-
puter Science, pages 270–281. Springer.
M¨uller, H. and Freytag, J.-C. (2003). Problems, Meth-
ods and Challenges in Comprehensive Data Cleans-
ing. Technical Report HUB-IB-164, Humboldt-
Universit¨at zu Berlin, Institut f¨ur Informatik.
Rahm, E. and Do, H. (2000). Data cleaning: Problems and
current approaches. IEEE Data Engineering Bulletin,
23(4):3–13.
Redman, T. C. (1998). The impact of poor data quality on
the typical enterprise. Commun. ACM, 41:79–82.
Sang Hyun, P., Wesley, W., et al. (2001). Discovering and
matching elastic rules from sequence databases. Fun-
damenta Informaticae, 47(1-2):75–90.
Strong, D. M., Lee, Y. W., and Wang, R. Y. (1997). Data
quality in context. Commun. ACM, 40(5):103–110.
Wang, R., Kon, H., and Madnick, S. (1993). Data quality
requirements analysis and modeling. In Data Engi-
neering, 1993. Proceedings. Ninth International Con-
ference on, pages 670–677.
Weidema, B. P. and Wesns, M. S. (1996). Data quality man-
agement for life cycle inventoriesan example of using
data quality indicators. Journal of Cleaner Produc-
tion, 4(34):167 – 174.
DATA2012-InternationalConferenceonDataTechnologiesandApplications
108