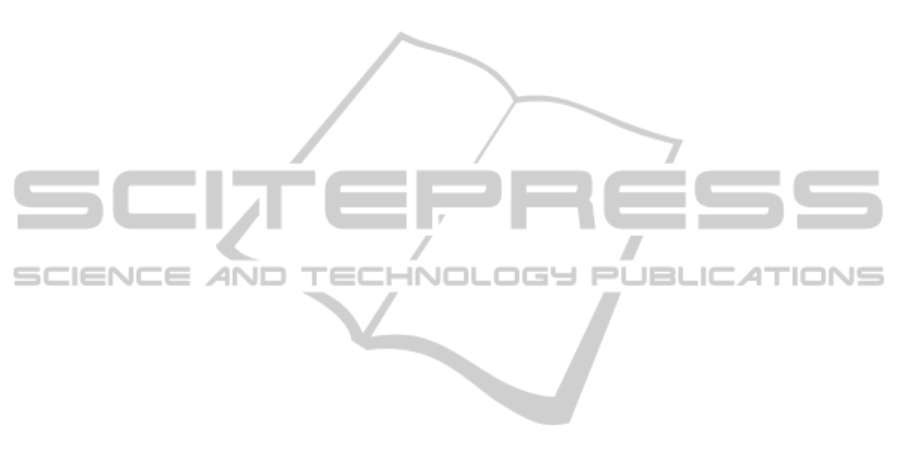
ures, since the Kalamn estimated covariance is negli-
gible (small), the OGK algorithm is not implemented
in the initial iterations. As the vehicle moves, the un-
certainties in the system noise will rapidly increas;
this requires the necessitates the implementation of
the data fusion algorithm. It is also observed that
the Kalman estimated covariance is emerging bigger
in each of the iterations by increasing the region of
uncertainties. Whenever the data fusion algorithm is
applied the region of this uncertainties is rapidly re-
duced by performing the measurement-update in the
Kalman filter. The last stages of iterations show that
the OGK algorithm is robust when the Kalman esti-
mates are unpredictable within the assumed distribu-
tions. In nutshell, the presented algorithms are com-
plementary in the sense that they compensate for each
other’s limitations, so that the resulting performance
is much better than of its individual techniques, which
in turn, provide more accurate position information of
the vehicle.
6 CONCLUSIONS
The paper presents robust data fusion techniques via
CI and a particular class of OGK covariance esti-
mators for fusion of information from two different
sources namely EKF and IA. The CI approach uses
the covariance matrices of the data sources whereas
the OGK uses an estimate of the joint covariance and
information from the measurements themselves. The
comparison between the two estimators is based on
the 2-norms. This measure combines information re-
lated to the volume of the error ellipsoids and their
eccentricity. The analysis on the relevant bounds for
the two measures shows that, in worst case conditions,
there are regions of the spectrum of the covariance
matrices in which each of the estimators outperforms
the other. For generic applications, a hybrid of the
two estimators may then provide the best results.
The application of this OGK estimation technique
to 3D needs a recalculation of the bounds involved.
Still, the same basic principles apply. The ongoing
work also includes the testing of classes of OGK es-
timators in the information fusion problem that are
applied to a number of ground and aerial vehicle in-
volved in a mapping mission.
REFERENCES
Ashokaraj, I. A. R. (2004). Feature based navigation and
localisation using interval analysis. Phd thesis, Cran-
field University.
Ashokaraj, I. A. R., Silson, P. M. G., Tsourdos, A., and
White, B. A. (2009). Robust sensor-based navigation
for mobile robots. IEEE Transactions on Instrumen-
tation and Measurement, 58(3):551–556.
Carvalho, K. F. A. C. D. and Rezende, S. O. (2004). Com-
bining intelligent techniques for sensor fusion. Ap-
plied Intelligence, 20:199213.
Copt, S. and Victoria-Feser, M. (2003). Fast algorithms for
computing high breakdown covariance matrices with
missing data.
Gnanadesikan, R. and Kettenring, J. (1972). Robust esti-
mates, residuals, and outlier detection with multire-
sponse data. Biometrics, 28(1):81–124.
Jaulin, L., Kieffer, M., Didrit, O., and Walter, E. (2001).
Applied Interval Analysis with examples in parame-
ter and state estimation robust control and robotics.
Springer-Verlag, London.
Jaulin, L. and Walter, E. (1993). Set inversion via interval
analysis for nonlinear bounded-error estimation. Au-
tomatica, 29(4):1053 to 1064.
Julier, S. J. and Uhlmann, J. K. (1997). A non-divergent
estimation algorithm in the presence of unknown cor-
relations. In American Control Conference, pages
2369–2373, Albuquerque, New Mexico.
Kieffer, M., Jaulin, L., Walter, E., and Meizel, D. (2000).
Robust autonomous robot localization using interval
analysis. Reliable Computing, 3(6):337 to 361.
Lazarus, S. B., Tsourdos, A., Silson, P., White, B. A.,
and Zbikowski, R. (2008). Unmanned aerial vehi-
cle navigation andmapping. Proc.IMechE. Part G: J.
Aerospace Engineering, 222(246):531 – 548.
L´evˆeque, O., Jaulin, L., Meizel, D., and Walter, E. (1997).
Vehicle localization from inaccurate telemetric data: a
set-inversion approach. In 5th IFAC Symp on Robot
Control SY.RO.CO. ’97, Nantes, France, volume 1,
pages 179 – 186.
Montemerlo, M., Thrun, S., Koller, D., and Wegbreit, B.
(2002). FastSLAM: A Factored Solution to the Si-
multaneous Localization and Mapping Problem. In
Procs. of the 8th AAAI National Conf. on Artificial In-
telligence. Edmonton, Alberta, Canada.
Mourikis, A. I. and Roumeliotis, S. I. (2006). Predicting
the Performance of Cooperative Simultaneous Local-
ization and Mapping (C-SLAM). The International
Journal of Robotics Research, 25(12):1273–1286.
Vadakkepat, P. and Jing, L. (2006). Improved particle filter
in sensor fusion for tracking randomly moving object.
IEEE Transactions on Instrumentation and Measure-
ment, 55(5):1823–1832.
Walter, M. and Leonard, J. (2004). An Experimental Inves-
tigation of Cooperative SLAM. In Procs. of the 5th
IFAC Symposium on Intelligent Autonomous Vehicles,
(IAV 2004). Lisbon, Portugal.
EKFbasedDataFusionusingIntervalAnalysisviaCovarianceIntersection,MLandaClassofOGKCovariance
Estimators
613