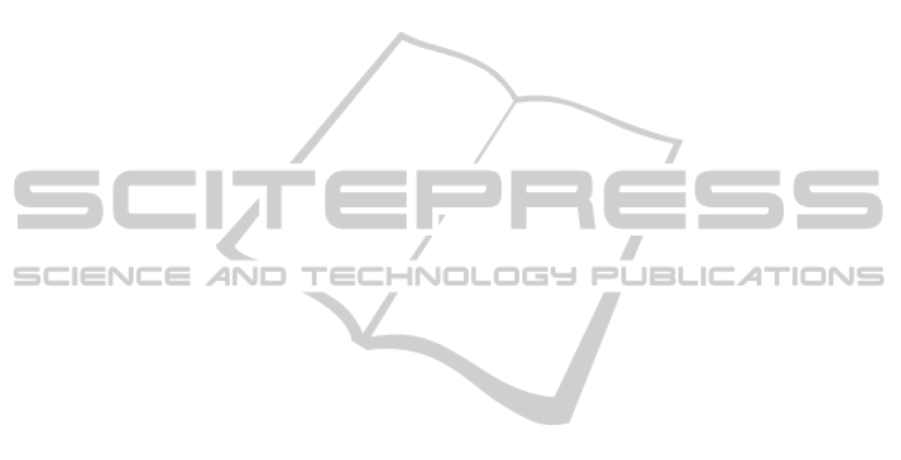
variance of 8×10
−4
[m
2
] for x, 2.3×10
−3
[m
2
] for y,
2.9 × 10
−3
[rad
2
] for the yaw. Even having collected
few samples, error distribution fits quite well with a
Gaussian (p ≤ 0.2). Considering a range of values
µ+3σ for the localization, with accuracy (p ≤ 0.003),
the platform has to maintain a distance greater than 9
cm along x and 15 cm along y from the target position
to avoid collisions.
Then we examined the accuracy of the table ap-
proach procedure. Both table and robot position
changed between tests. The results of 10 tests show a
variance of 1.12 × 10
−4
[m
2
] for x, 1.14 × 10
−4
[m
2
]
for y, 4.4 × 10
−4
[rad
2
] for the yaw. Considering a
range of values µ + 3σ for the localization, the plat-
form has to maintain a distance about 3 cm along x
and y, taking into account a yaw error slightly greater
than 3 degrees, to approach the table without colli-
sions. An error of 3 cm does not invalidate the plat-
form capability of grasping (Figure 4).
The last set of experiments determines the good-
ness and the accuracy of objects grasping. We carried
out 10 tests placing the object in different areas of
the table and starting the robot always with the table
markers visible. The arm grasps the object in 9 of 10
tests (90%). The tests show a variance of 3.2 × 10
−7
[m
2
] for x, 2.4 × 10
−5
[m
2
] for y. Considering a range
of values µ + 3σ for the object recognition, we can
estimate that the error made in recognizing an object
falls within the limits of about 0.17 cm along x and
1.48 cm along y. The error obtained, being smaller
than the opening wideness of the gripper ( 2.3 cm),
does not invalidate the grasp capability of the plat-
form. No phase is secure/robust, but the consequen-
tiality of phases ensures a progressive refinement that
avoids collisions and gives capability to grasp.
6 CONCLUSIONS AND FUTURE
WORK
We presented and validated a learning by demonstra-
tion system. It integrates action and perception algo-
rithms to learn and execute household tasks adapting
them to its physicality. We validated every module
efficiency and integration through a series of experi-
mental tests. We plan to adapt the system to be con-
trollerd with biometric readings to use the robot as an
auxiliary body for the human user and to have arm
movements learned through demonstrated examples
(Avizzano, 2012).
REFERENCES
Avizzano, C. A. (2012). Guided latent space regression
for human motion generation. In Robotic and Au-
tonomous Systems, Special Issue on SKILLS.
Beetz, M., Tenorth, M., Jain, D., and Bandouch, J. (2010).
Towards automated models of activities of daily life.
In Technology and Disability. IOS Press.
Bischoff, R., Huggenberger, U., and Prassler, E. (2011).
Kuka youbot - a mobile manipulator for research and
education. In In Proc. of the IEEE International Con-
ference on Robotics and Automation.
Ciocarlie, M., Hsiao, K., et al. (2010). Towards reli-
able grasping and manipulation in household environ-
ments. In In Proc. of Intl. Symposium on Experimental
Robotics (ISER).
Di Fava, A., Peppoloni, L., Avizzano, C. A., and Ruffaldi,
E. (2012). A cognitive learning architecture for a mo-
bile robotic manipulator. In Robot and Human Inter-
active Communication, 2012. Proceedings. RO-MAN
2012 (to be submitted).
Fox, D., Burgard, W., Dellaert, F., and Thrun, S. (1999).
Monte carlo localization: Efficient position estimation
for mobile robots. In In Proc. of the National Confer-
ence on Artificial Intelligence (AAAI).
Fox, D., Burgard, W., and Thrun, S. (1997). The dynamic
window approach to collision avoidance. In in IEEE
Robotics and Automation, Vol. 4, No. 1.
Khoshelham, K. (2011). Accuracy analysis of kinect depth
data. In In Proc. of ISPRS Workshop Laser Scanning
2011.
Lai, K., Bo, L., Ren, X., and Fox, D. (2011). A large-
scale hierarchical multi-view rgb-d object dataset. In
In Proc. of the IEEE International Conference on
Robotics and Automation.
Malbezin, P., Piekarski, W., and Thomas, B. (2002). Mea-
suring artootkit accuracy in long distance tracking
experiments. In In Proc. of the Augmented Reality
Toolkit, The First IEEE International Workshop.
Marder-Eppstein, E., Berger, E., et al. (2010). The office
marathon: Robust navigation in an indoor office envi-
ronment. In In Proc. of the IEEE International Con-
ference on Robotics and Automation.
Miller, A. and Allen, P. K. (2004). Graspit!: A versatile
simulator for robotic grasping. In in IEEE Robotics
and Automation Magazine, V. 11, No.4.
Pangercic, D., Tenorth, M., Jain, D., and Beetz, M. (2010).
Combining perception and knowledge processing for
everyday manipulation. In In Proc. of Intelligent
Robots and Systems (IROS), 2010 IEEE/RSJ Interna-
tional Conference on.
Quigley, M., Conley, K., et al. (2009). Ros: an open-source
robot operating system. In In Proc. of the ICRA Work-
shop on Open Source Software.
Rusu, R. B., Sucan, I. A., et al. (2009). Real-time
perception-guided motion planning for a personal
robot. In In Proc. of Intelligent Robots and Systems
(IROS), 2009 IEEE/RSJ International Conference on.
ProgrammingofaMobileRoboticManipulatorthroughDemonstration
471