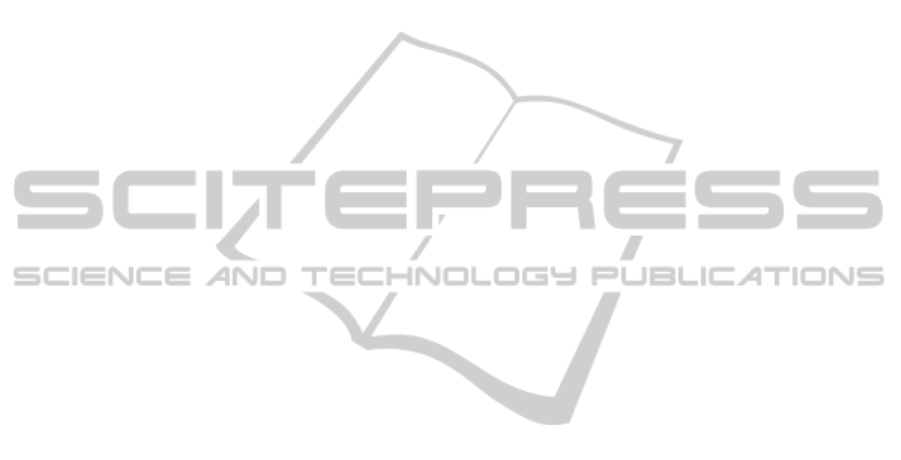
regardless the relative position of the nearby objects.
The increase in the number of false positives to
higher velocities is based on the fact that, at higher
velocities, the difference between successive frames
is higher, leading to the production of high
excitation levels and, consequently, a bigger number
of collision detection alarms.
Although the difference verified in relation to
correct collision detections between different
velocities, the results obtained are very satisfactory, as
the number of correct detections are always higher
than the sum of missed and false positive detections.
4 CONCLUSIONS
In this paper, we propose a modified LGMD model
based on the identified LGMD neuron of the locust
brain. The model proved to be a robust collision
detector for autonomous robots. This model has a
mechanism that favours grouped excitation, as well as
two cells with a particular behaviour that provide
additional information on the depth direction of
movement.
For applications as collision detectors in
robotics, the model proposed is able to remove the
noise captured by the camera, as well as enhance its
ability to recognize the direction of the object
movement and, by this way, remove the false
collision alarms produced by the previous models
when a nearby object is moving away.
Experiments with a DRK8000 robot showed that
with these two new
procedures, the robot was able to
travel autonomously in real time and within a real
arena.
The results illustrate the benefits of the LGMD
based neural network here proposed, and, in the
future, we will continue to use and enhance this
approach, using, for that, a combination of
physiological and anatomical studies of the locust
visual system, in order to improve our understanding
about the relation between the LGMD neuron output
and the locust muscles related to the avoidance
manoeuvres.
ACKNOWLEDGEMENTS
Work supported by the Portuguese Science
Foundation (grant PTDC/EEA-CRO/100655/2008).
Ana Silva is supported by PhD Grant
SFRH/BD/70396/2010, granted by the Portuguese
Science Foundation.
REFERENCES
Badia, S. B. i., Bernardet, U. & Verschure, P. F. M. J.,
2010. Non-Linear Neuronal Responses as an Emergent
Property of Afferent Networks: A Case Study of the
Locust Lobula Giant Movement Detector. PLoS
Comput Biol, 6(3), p. e1000701.
Blanchard, M., Rind, F. C. & Verschure, P. F. M. J., 2000.
Collision avoidance using a model of the locust
LGMD neuron. Robotics and Autonomous Systems,
30(1), pp. 17-37.
Gabbiani, F., Krapp, H. G., Koch, C. & Laurent, G., 2002.
Multiplicative computation in a visual neuron sensitive
to looming. Nature, Volume 420, pp. 320-324.
Gabbiani, F., Krapp, H. & Laurent, G., 1999. Computation
of object approach by a wide-field motion-sensitive
neuron. J. Neurosci., Volume 19, 1122-1141.
Gabbiani, F., Mo , C. & Laurent, G., 2001. Invariance of
Angular Threshold Computation in a Wide-Field
Looming-Sensitive Neuron. The Journal of
Neuroscience, 21(1), p. 314–329.
Gray, John R , J. R., Lee, J. K. & Robertson, R., 2001.
Activity of descending contralateral movement detector
neurons and collision avoidance behaviour in response
to head-on visual stimuli in locusts. Journal of
Comparative Physiology A, Volume 187, pp. 115-129.
Gray, J. R., Blincow, E. & Robertson, R., 2010. A pair of
motion-sensitive neurons in the locust encode approaches
of a looming object. Journal of Comparative Physiology
A: Neuroethology, Sensory, Neural, and Behavioral
Physiology, 196(12), pp. 927-938.
Guest, B. B. & Gray, J. R., 2006. Responses of a looming-
sensitive neuron to compound and paired object
approaches. Journal of Neurophysiology, 95(3), pp.
1428-1441.
MATLAB, 2011. version 7.12.0 (R2011a). Natick,
Massachusetts: The MathWorks Inc..
Meng, H., Yue, S., Hunter, A. Appiah, K., Hobden, M.
Priestley, N., Hobden, P. & Pettit, C., 2009. A
modified neural network model for the Lobula Giant
Movement Detector with additional depth movement
feature. Atlanta, Georgia: Proceedings of International
Joint Conference on Neural Networks, 14-19 June.
Rind, F., 1987. Non-directional, movement sensitive neurones
of the locust optic lobe. Journal of Comparative
Physiology A: Neuroethology, Sensory, Neural, and
Behavioral Physiology, Volume 161, pp. 477-494.
Rind, F. C. & Bramwell, D. I., 1996. Neural Network
Based on the Input Organization of an Identified
Neuron Signaling Impeding Collision. Journal of
Neurophysiology, 75(3), pp. 967-985.
Stafford, R., Santer, R. D. & Rind, F. C., 2007. A bio-
inspired visual collision detection mechanism for cars:
combining insect inspired neurons to create a robust
system. BioSystems, Volume 87, pp. 164-171.
Yue, S. & Rind, F.C., 2006. Collision detection in
complex dynamic scenes using an LGMD-based
visual neural network with feature enhancement. IEEE
transactions on neural networks. 17 (3), 705-716.
ISSN 1045-9227.
ICINCO2012-9thInternationalConferenceonInformaticsinControl,AutomationandRobotics
140