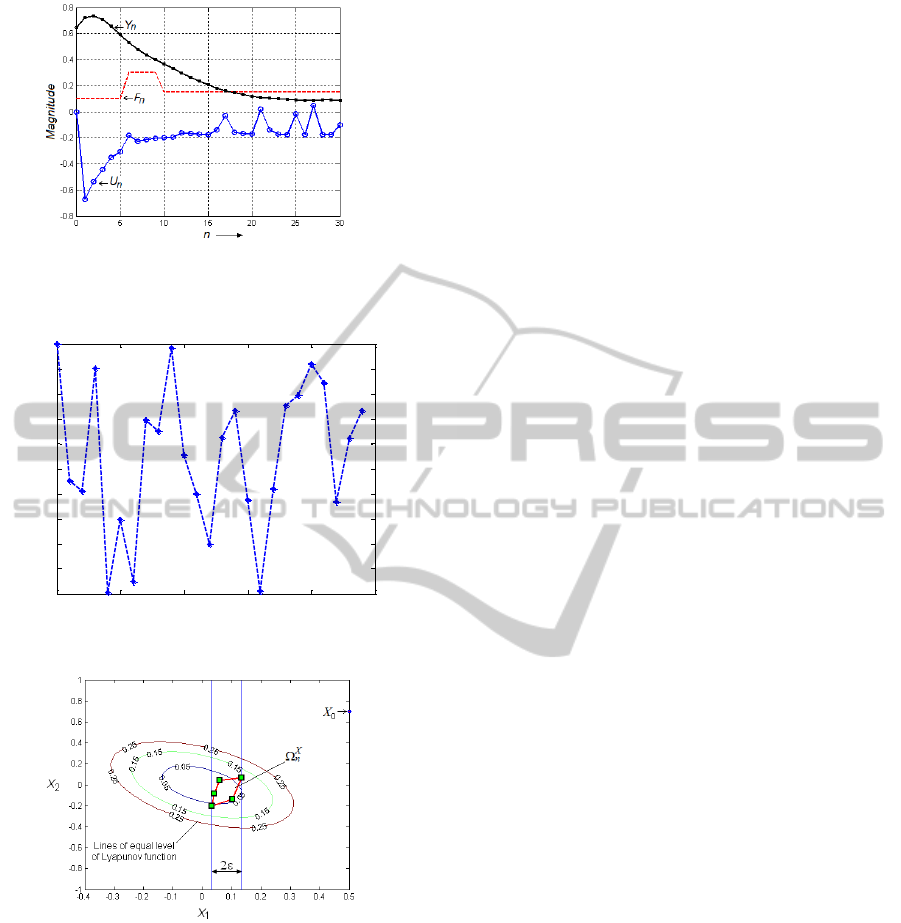
Figure 4: Graphics of: a) modification of system output Y
n
,
disturbance F
n
and control U
n
.
0 5 10 15 20 25
-0.025
-0.02
-0.015
-0.01
-0.005
0
0.005
0.01
0.015
0.02
0.025
measurement noise
Figure 5: The measurement noise.
Figure 6: Set
of potential EMR’s states at n=30.
As evident from Fig. 4-6 the proposed controller
ensures enough control quality. For system quality
improvement it is necessary to use the observer
giving a specified multiple rating of possible
perturbations at each control step.
7 CONCLUSIONS
In this paper we considered the problem of robust
control of EMR. The unique technical vision system
for EMR’s sensory system, providing real-time
continuous feedback in Cartesian coordinates, was
proposed. A new algorithm was given for the state
filtering for robust control determination.
The presented algorithm allows intersect convex
polyhedrons. As the actual systems are nonlinear, it
is necessary to be able to intersect non-convex sets.
It is an objective for future work. Also expedient to
note that additional increase of technical vision
resolution in future can be reached by
implementation of our original method of scales
binding described in (Sergiyenko, 2011).
ACKNOWLEDGEMENTS
This work has been supported by the Ministry of
Science and Innovation (MICINN) of Spain under
the research project TEC2010-17429, and the
Universidad Politecnica de Madrid under the
research project AL12-PID-31.
REFERENCES
Eryemenko, I., Gurko, A., 2009. Realization of the Game
Approach to Control of Second Order Linear Objects.
Journal of Automation and Information Sciences,
Begell House Inc., NY, USA, Vol.: 41, pp.10-22.
Gurko, A., Eryemenko, I., 2011. Control of discrete
system under bounded disturbances. Journal of
Automation and Information Sciences, Begell House
Inc., NY, USA, Vol.: 43, Issue 11, pp. 18-29.
Lamon, P., Siegwart, R., 2007. 3D Position Tracking in
Challenging Terrain. The International Journal of
Robotics Research, Vol.: 26, pp.167-188.
Miller, W. T., Sutton, R. S., Werbos, P. J., 1996. Neural
Networks for Control, MIT Press, 5
th
edition, 542 p.
Rivas, M., et al., 2008. Spatial data acquisition by laser
scanning for robot or SHM task. In ISIE’08, IEEE-IES
International Symposium on Industrial Electronics,
Cambridge, United Kingdom, pp.1458-1463.
Selekwa, M. F., Dunlap, D. D., Shi, D., Collins, E. G.,
2008. Robot navigation in very cluttered environments
by preference-based fuzzy behaviors. Robotics and
Autonomous Systems, Elsevier, Vol: 56, pp. 231-246.
Sergiyenko, O., Hernandez, W., et al., 2009. Remote
Sensor for Spatial Measurements by Using Optical
Scanning. Sensors, Basel, Switzerland, 9(7), pp. 5477-
5492.
Sergiyenko, О., Tyrsa, V., Devia, L., Hernandez, W., et al,
2009. Dynamic Laser Scanning method for Mobile
Robot Navigation. In ICCAS-SICE’09, ICROS-SICE
International Joint Conference, Fukuoka, Japan,
August 18-21, pp. 4884-4889.
Sergiyenko, O., et al., 2011. Analysis of jitter influence in
fast frequency measurements. Measurement, Elsevier,
Vol. 44, Issue 7, pp. 1229-1242.
ICINCO 2012 - 9th International Conference on Informatics in Control, Automation and Robotics
484