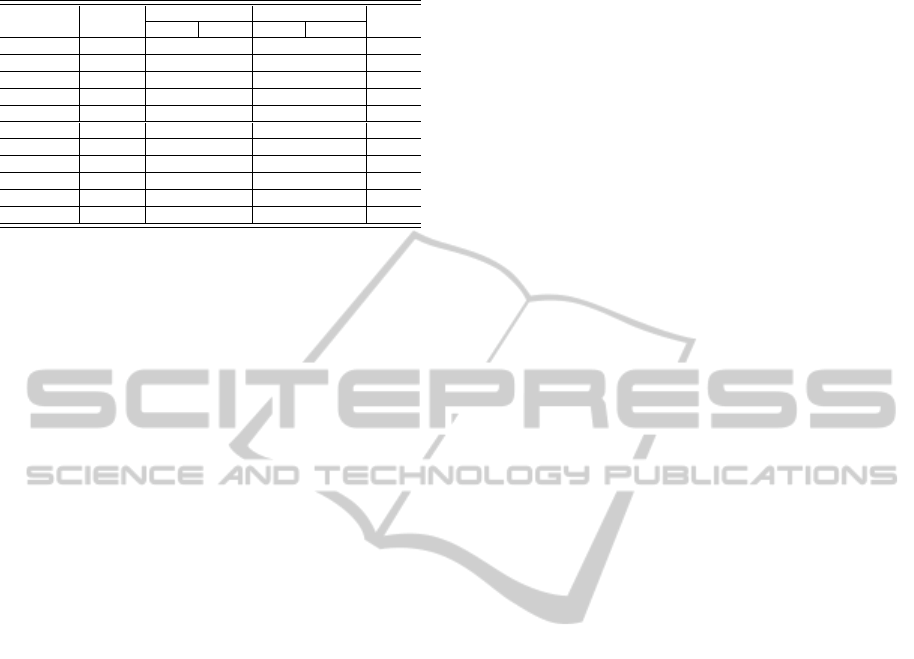
Table 6: Validation Test 5.
Brazilian English HMM HMM GFIS
Digits Digits sn=3 pn=8 sn=3 pn=12 pn=4
ZERO (zero) 4 5 8
UM (one) 5 9 4
DOIS (two) 9 9 4
TRES (three) 3 4 3
QUATRO (four) 4 5 5
CINCO (five) 9 7 10
SEIS (six) 5 6 5
SETE (seven) 8 6 8
OITO (eight) 9 8 10
NOVE (nine) 6 6 10
Total(%) 62% 65% 67%
lar technique of noise reduction, such as those com-
monly used in HMM-based recognizers, was not used
during the development of this paper. It is believed
that with proper treatment of the signal to noise ratio
in the process of training and testing, the GFIS Rec-
ognizer may improve its performance:
1. Increase the speech bank with different accents;
2. Improve the performance of genetic algorithm to
100% recognition in the training process;
3. Use Nonlinear Predicitve Coding for feature ex-
traction in speech recognition;
4. Use Digital Filter in the speech signal to be rec-
ognized.
5. Increase the parameters number used.
ACKNOWLEDGEMENTS
The authors would like to thank FAPEMA for fi-
nancial support, research group of computational in-
telligence applied to technology at the IFMA by its
infrastructure for this research and experimental re-
sults, and the Master and PhD program in Eletrical
Engineering at the Federal University of Maranh˜ao
(UFMA).
REFERENCES
Ahmed, N., Natajaran, T., and Rao, K. R. (1974). Discrete
cosine transform. IEEE Transaction on Computers,
C-23:90–93.
Andrews, H. C. (1971). Multidimensional rotations in fea-
ture selection. IEEE Transaction on Computers, C-
20:1045–1051.
Ariki, Y., Mizuta, S., Nagata, M., and Sakai, T. (1989).
Spoken- word recognition using dynamic features
analysed by two-dimensional cepstrum. IEEE Pro-
ceedings, 136(v.2):133–140.
Azar, M. Y. and Razzazi, F. (2008). A dct based nonlin-
ear predictive coding for feature extraction in speech
recognition systems. IEE International Conference on
Computational Intelligente for Measurement Systems
and Applications, pages 19 – 22.
Babuska, R. (1998). Fuzzy Modeling for Control. Kluwer
Academic Publishers.
Fissore, L., Laface, P., and Rivera, E. (1997). Using
word temporal structure in hmm speech recongnition.
ICASSP 97, v.2:975–978.
Fu, K. S. (1968). Sequential Methods in Pattern Recog-
nition and Machine Learning. Acadmic Press, New
York.
Gang, C. (2010). Discussion of approximation properties of
minimum inference fuzzy system. Proceedings of the
29th Chinese Control Conference, pages 2540–2546.
Gosztolya, G., Dombi, J., and Kocsor, A. (2009). Applying
the generalized dombi operator family to the speech
recognition task. Journal of Computing and Informa-
tion Technology, pages 285–293.
Haupt, R. L. and Haupt, S. E. (2004). Pratical Genetic Al-
gorithms. John Wiley and Sons, New York.
Milner, B. P., Conner, P. N., and Vaseghi, S. V. (1994).
Speech modeling using cepstral-time feature and hid-
den markov models. Communications, Speech and Vi-
sion, IEE Proceedings I, v.140(5):601–604.
Monserrat, M., Torrens, J., and Trillas, E. (2007). A survey
on fuzzy implication functions. IEEE Transactions on
Fuzzy Systems, v.15(6):1107–1121.
Picone, J. W. (1991). Signal modeling techiniques in speech
recognition. IEEE Transactions, v.79:1214–1247.
Rabiner, L. and Hwang, J. B. (1993). Fundamentals of
Speech Recognition. Prentice Hall, New Jersey.
Seki, H., Ishii, H., and Mizumoto, M. (2010). On the mono-
tonicity of fuzzy inference methods related to ts infer-
ence method. IEEE Transactions on Fuzzy Systems,
v.18(3):629–634.
Shenouda, S. D., Zaki, F. W., and Goneid, A. M. R. (2006).
Hybrid fuzzy hmm system for arabic connectionist
speech recognition. The 23rd National U.Jio Science
Conference (NRSC 2006), v.0:1–8.
Silva, W. L. S. and Serra, G. L. O. (2011). Proposta de
metodologia tcd-fuzzy para reconhecimento de voz. X
SBAI:Simposio Brasileiro de Automacao Inteligente,
pages 1054–1059.
Tang, C., Lai, E., and Wang, Y. C. (1997). Distributed fuzzy
rules for preprocessing of speech segmentation with
genetic algorithm. Fuzzy Systems, Proceedings of the
Sixth IEEE International Conference on, v.1:427–431.
Wachter, M., Matton, M., Demuynck, K., Wambacq, P.,
Cools, R., and Compernolle, D. V. (2007). Template-
based continuous speech recognition. IEEE Trans-
actions on Audio, Speech, and Language Processing,
v.15:1377–1390.
Wang, L. X. (1994). A course in Fuzzy Systems and Control.
Prentice Hall.
Zhou, J. and Chen, P. (2009). Generalized discrete cosine
transform. Circuits, Communications and Systems,
PACCS 2009, Pacific Asia Conference on, pages 449–
452.
GFIS:GeneticFuzzyInferenceSystemforSpeechRecognition
541