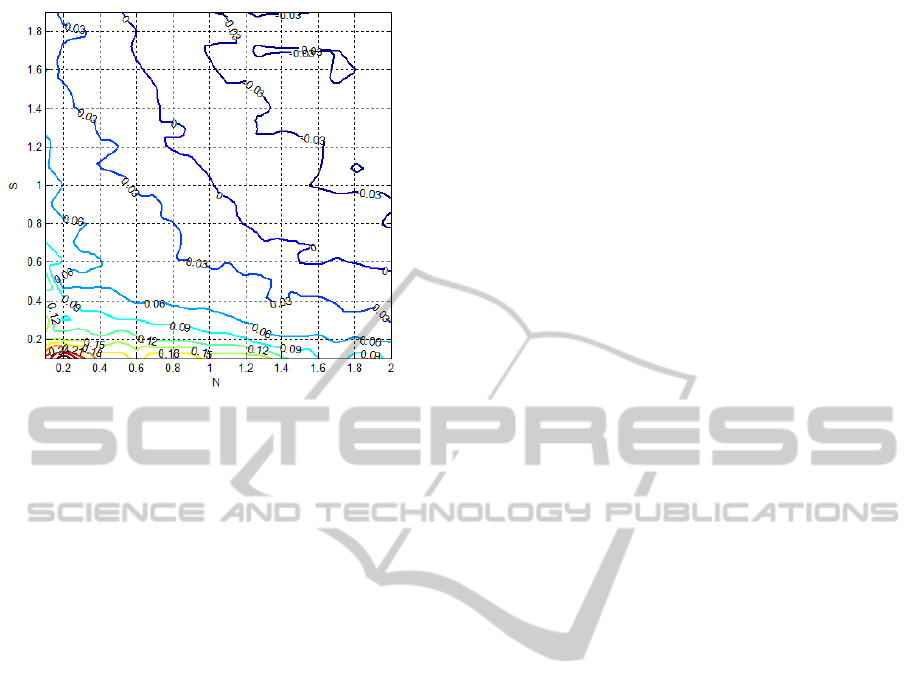
Figure 5: Surface that represents the behavior of the system
for diferent parameters of N and S.
application of the visualization model is based on very
simple assumptions. In fact, all the interactions are
based on proportionality of the considered types of
interacting parameters. This makes some of the re-
sults of a social evolution quite predictable in terms
of common cense most of humans have in terms of
society behavior. The application of the visualization
method to such case showed consistent results accord-
ing to those common cense, which gives validation to
the proposed method inside the scope of the consid-
ered case.
As a future work, the visualization model shall
permit to interfere on the evolution of a MAS in real-
time by the possibility of modification of individual
parameters to interfere on the system’s fate before
the asymptotic state is achieved. We intend to im-
prove the user interaction to permit select an agent
or a group of agents and change their parameters dur-
ing simulation and analyze how the system as a whole
would react. In this way, we could know what agents
or groups of agents are crucial to desired certain con-
vergence of the system. We also are working to im-
prove S parameter to consider it as a n-dimensional
vector to make it possible to include other agents char-
acteristics and choi ces related to its gender, work,
hobbies, etc.
REFERENCES
Deutsch, M. and Gerard, H. B. (1955). A study of norma-
tive and informational social influences upon individ-
ual judgment. The Journal of Abnormal and Social
Psychology, 51:629–636.
Guy, S. J., Chhugani, J., Curtis, S., Dubey, P., Lin, M., and
Manocha, D. (2010). Pledestrians: a least-effort ap-
proach to crowd simulation. In Proceedings of the
2010 ACM SIGGRAPH/Eurographics Symposium on
Computer Animation, SCA ’10, pages 119–128, Aire-
la-Ville, Switzerland, Switzerland. Eurographics As-
sociation.
Helbing, D. (2009). Managing complexity in socio-
economic systems. European Review, 17:423–438.
Macy, M. W. and Willer, R. (2002). From factors to actors:
Computational sociology and agent-based modeling.
Annual Review of Sociology, 28:143–166.
Mark, N. (1998). Beyond individual differences: Social
differentiation from first principles. American Socio-
logical Review, 63(3):pp. 309–330.
Narain, R., Golas, A., Curtis, S., and Lin, M. C. (2009). Ag-
gregate dynamics for dense crowd simulation. ACM
Trans. Graph., 28:122:1–122:8.
Newman, M. E. J. (2003). The structure and function of
complex networks. SIAM REVIEW, 45:167–256.
Ondrej, J., Pettre, J., Olivier, A.-H., and Donikian, S.
(2010). A synthetic-vision-based steering approach
for crowd simulation. SIGGRAPH ’10: ACM SIG-
GRAPH 2010 Papers.
Prazak, M., Kavan, L., McDonnell, R., Dobbyn, S., and
O’Sullivan, C. (2009). Moving crowds: a linear ani-
mation system for crowd simulation. In Proceedings
of the 2009 symposium on Interactive 3D graphics and
games, I3D ’09, pages 9:1–9:1, New York, NY, USA.
ACM.
Qiu, F. and Hu, X. (2010). Modeling group structures in
pedestrian crowd simulation. Simulation Modelling
Practice and Theory, 18(2):190 – 205.
Resnick, M. (1994). Turtles, termites, and traffic jams: ex-
plorations in massively parallel microworlds. MIT
Press, Cambridge, MA, USA.
Reynolds, C. W. (1987). Flocks, herds and schools: A
distributed behavioral model. SIGGRAPH Comput.
Graph., 21(4):25–34.
Vigueras, G., Lozano, M., Ordu
˜
na, J. M., and Grimaldo,
F. (2010). A comparative study of partitioning meth-
ods for crowd simulations. Appl. Soft Comput.,
10(1):225–235.
Villamil, M. B., Oliveira, L. P. L., and Musse, S. R. (2005).
A model for generating competitive groups based on
virtual agents progression. In International Con-
ference on Computer Animation and Social Agents,
pages 133–138. Hong Kong Polytechnic University.
ICINCO2012-9thInternationalConferenceonInformaticsinControl,AutomationandRobotics
344