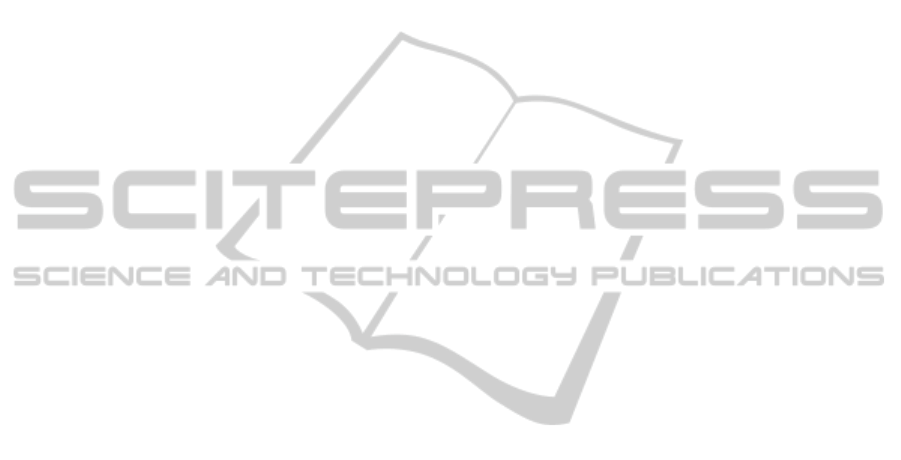
given in a 1-5 scale, where 1 stands for ‘I strongly
disagree’ and 5 for ‘I strongly agree’.
With respect to the overall quality of the Dicode
Workbench, the evaluators agreed (median: 4, mode:
4) that its objectives are met, that it is novel to their
knowledge, that are satisfied with its performance
and that they are overall satisfied with it. The
evaluators were neutral (median: 3, mode: 3) with
respect to whether the Workbench addressed the
data intensive decision making issues. As long as its
acceptability is concerned, the evaluators agreed
(median: 4, mode: 4) that the Workbench has the full
set of functions they expected, that its interface is
pleasant and that they will recommend it to their
peers/community.
6 CONCLUSIONS
Taking into account the feedback received from the
first evaluation phase of the Dicode project, we
argue that our overall approach offers an innovative
solution that reduces the data-intensiveness and
overall complexity of real-life collaboration and
decision making settings to a manageable level, thus
permitting stakeholders to be more productive and
concentrate on creative activities. Towards this
direction, the project provides a suite of innovative,
adaptive and interoperable services that satisfies the
requirements reported in Section 2.
A major future work direction concerns the
improvement of Dicode services in terms of their
documentation, user interfaces and performance.
Another one concerns testing of these services in
various data-intensive contexts towards further
assessing their applicability and potential.
ACKNOWLEDGEMENTS
This publication has been produced in the context of
the EU Collaborative Project “DICODE - Mastering
Data-Intensive Collaboration and Decision Making”,
which is co-funded by the European Commission
under the contract FP7-ICT-257184. This
publication reflects only the authors’ views and the
Community is not liable for any use that may be
made of the information contained therein.
REFERENCES
Adomavicius, G., Tuzhilin, A., 2005. Toward the Next
Generation of Recommender Systems: A Survey of
the State-of-the-Art and Possible Extensions. IEEE
Transactions on Knowledge and Data Engineering
17(6), pp. 734–749.
Eppler, M. J., Mengis, J., 2004. The Concept of
Information Overload: A Review of Literature from
Organization Science, Accounting, Marketing, MIS,
and Related Disciplines. The Information Society 20,
pp. 325–344.
Grosskreutz, H., Rüping, S., Wrobel, S., 2008. Tight
Optimistic Estimates for Fast Subgroup Discovery. In:
Proceedings of the European Conference on Machine
Learning and Principles and Practice of Knowledge
Discovery in Databases. Springer LNAI.
Hara, N., Solomon, P., Kim, S., Sonnenwald, H., 2003. An
emerging view of scientific collaboration: Scientists’
perspectives on collaboration and factors that impact
collaboration. Journal of the American Society for
Information Science and Technology 54(10), pp. 952–
965.
Karacapilidis, N., Papadias, D., 2001. Computer
Supported Argumentation and Collaborative Decision
Making: The HERMES system. Information Systems
26(4), pp. 259-277.
Kloesgen, W., 1996. Explora: A Multi-pattern and Multi-
strategy Discovery Assistant. In: Advances in
Knowledge Discovery and Data Mining. MIT Press.
Kunz, W., Rittel, H., 1970. Issues as Elements of
Information Systems. Technical Report 0131,
Universität Stuttgart, Institut für Grundlagen der
Planning.
Rüping, S., 2009. Ranking Interesting Subgroups. In: L.
Bottou and M. Littman (Eds.), Proceedings of the 26th
International Conference on Machine Learning (ICML
2009). Omnipress, pp. 913-920.
Schur, A., Keating, K. A., Payne, D. A., Valdez, T., Yates,
K. R., Myers, J. D., 1998. Collaborative suites for
experiment-oriented scientific research. Interactions
3(5), pp. 40–47.
Shipman, F. M., McCall, R., 1994. Supporting knowledge-
base evolution with incremental formalization. In:
Proceedings of the CHI ’94 Conference. pp. 285-291.
Trajkovski, J., Zelezny, F., Tolar, J., Lavrac, N., 2006.
Relational Subgroup Discovery for Descriptive
Analysis of Microarray Data. In: M. Berthold, R.
Glen, I. Fischer (Eds.), Computational Life Sciences
II. Springer, pp. 86-96.
DATA2012-InternationalConferenceonDataTechnologiesandApplications
146