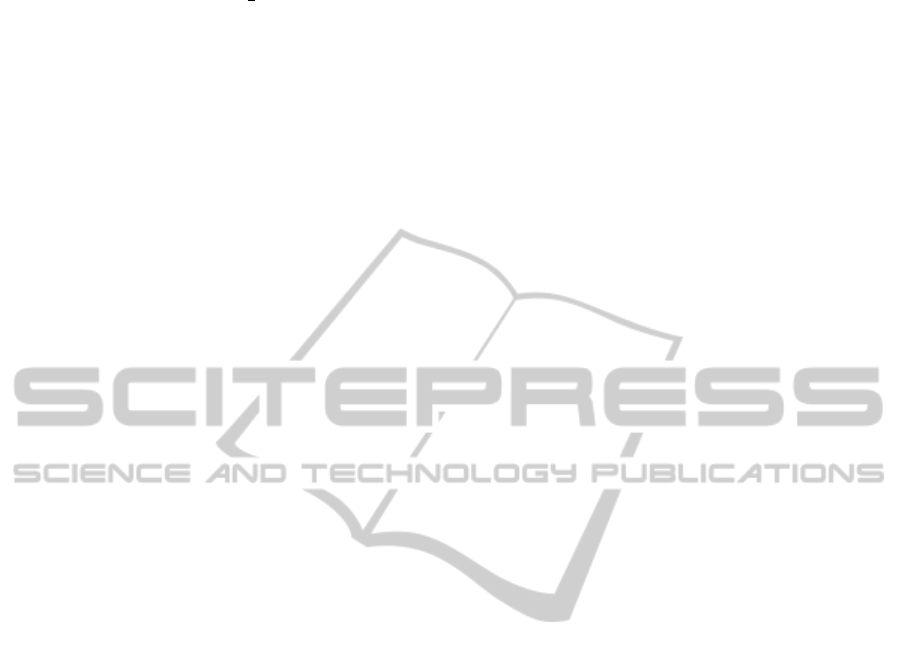
effective.
Considering the setting with s
c
= 0.2 (Table 2.b),
the higher minimum support threshold obtains, as an
effect, that only strongly representative itemsets actu-
ally contribute to the value of APRV. Consequently,
movie Little Fockers now occupies position 7, thus
significantly improving its position.
Of course, these are preliminary results and a
deeper study must be performed, evaluating precision
and recall of the different settings.
6 CONCLUSIONS
In this paper we presented a novel retrieval model for
a product search engine based on user-reviews taken
from the internet.
The two basic ideas that reside under the search
engine are (1) itemset computation at query time
from frequent terms in user-reviews and (2) a ranking
model that permits to weight these itemsets. The work
is at an early stage, but based on our experiments we
can say that the whole idea seems to be quite promis-
ing.
Future Works. Our retrieval model is very context-
dependent so we have to develop automatic criteria
to enrich a generic stop-words list with those terms
that are too much context frequent. A too much con-
text frequent term has no relevant semantic value, but
nonetheless it can affect ranking in a distorted way.
For examples, in the case we showed in this paper the
terms movie and movies are considered stop-words.
Details apart, the next step is to improve our rank-
ing model including the concept of term-closeness.
At the moment while matching a query in a user-
review we do not consider term position inside the
review. We do believe that matching closer terms can
lead closer to the real meaning of the query. So we
want to develop an index based on term-closeness to
affect our ranking model.
A further step is to hook our search engine to a
dictionary or an ontology, like Wordnet, in order to
better characterize words.
REFERENCES
Agrawal, R., Imielinski, T., and Swami, A. (1993).
Database mining: A performance perspective. IEEE
Transactions on Knowledge and Data Engineering,
5(6):914–925.
Agrawal, R. and Srikant, R. (1994). Fast algorithms for
mining association rules in large databases. In Pro-
ceedings of the 20th VLDB Conference, Santiago,
Chile.
Chaovalit, P. and Zhou, L. (2005). Movie review mining:
a comparison between supervised and unsupervised
classification approaches. In HICSS. IEEE Computer
Society.
Dave, K., Lawrence, S., and Pennock, D. M. (2003). Min-
ing the peanut gallery: opinion extraction and seman-
tic classification of product reviews. In WWW, pages
519–528.
Hu, M. and Liu, B. (2004a). Mining and summarizing cus-
tomer reviews. In Kim, W., Kohavi, R., Gehrke, J.,
and DuMouchel, W., editors, KDD, pages 168–177.
ACM.
Hu, M. and Liu, B. (2004b). Mining opinion features in
customer reviews. In McGuinness, D. L. and Fergu-
son, G., editors, AAAI, pages 755–760. AAAI Press /
The MIT Press.
Kim, W., Ryu, J., Kim, K. I., and Kim, U.-M. (2009). A
method for opinion mining of product reviews using
association rules. In Sohn, S., Chen, L., Hwang, S.,
Cho, K., Kawata, S., Um, K., Ko, F. I. S., Kwack, K.-
D., Lee, J. H., Kou, G., Nakamura, K., Fong, A. C. M.,
and Ma, P. C. M., editors, Int. Conf. Interaction Sci-
ences, volume 403 of ACM International Conference
Proceeding Series, pages 270–274. ACM.
Lee, D., Jeong, O.-R., and goo Lee, S. (2008). Opinion
mining of customer feedback data on the web. In Kim,
W. and Choi, H.-J., editors, ICUIMC, pages 230–235.
ACM.
Liu, B., Hu, M., and Cheng, J. (2005). Opinion observer:
analyzing and comparing opinions on the web. In El-
lis, A. and Hagino, T., editors, WWW, pages 342–351.
ACM.
Liu, D. T. and Xu, X. W. (2001). A review of web-based
product data manageement systems. 44(1):251–262.
Ly, D. K., Sugiyama, K., Lin, Z., and Kan, M.-Y. (2011).
Product review summarization based on facet identifi-
cation and sentence clustering. CoRR, abs/1110.1428.
Sandvig, J. J., Mobasher, B., and Burke, R. D. (2007). Ro-
bustness of collaborative recommendation based on
association rule mining. In Konstan, J. A., Riedl, J.,
and Smyth, B., editors, RecSys, pages 105–112. ACM.
Zhuang, L., Jing, F., and Zhu, X. (2006). Movie review
mining and summarization. In Yu, P. S., Tsotras, V. J.,
Fox, E. A., and Liu, B., editors, CIKM, pages 43–50.
ACM.
DATA2012-InternationalConferenceonDataTechnologiesandApplications
228