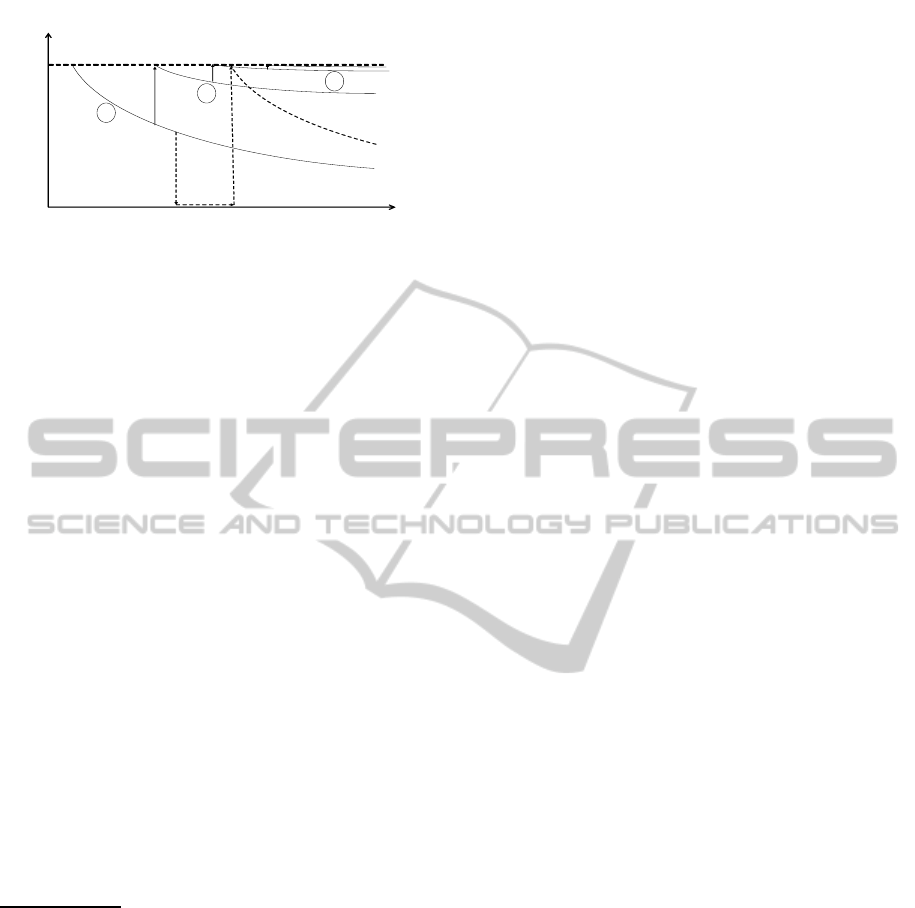
1
0
t
Retention
probability
1
2
3
Figure 6: Ebbinghous’s retention function.
This problem is related to Ebbinghous’ (Ebbing-
hous, 1964, 1885) retention function which was used
to describe experimental results of memory of a list of
nonsense syllables. No distinction is made between a
short-term memory and a long-term memory. The re-
tention function is typically considered as taking the
shape of any curved line depicted in Figure 6, where
the height denotes the probability of retaining a mem-
ory and it is diminishing with time
4
.
This direction may become more fruitful with
an experimental study such as in (Takeuchi, Funaki,
Kaneko, and Kline, 2011).
Aspect 2: Two or More Learners. We have concen-
trated our focus on the example of Mike’s Bike Com-
muting. We are interested in learning in game situa-
tions with two or more learners (players)
5
. This has
other new features like the relevant learning time. For
example, one may learn over his life time, but only
interact with another player for a shorter time span.
Also, how does his learning affect the other’s learn-
ing? We might start with the “small and partial views”
setting of (Kaneko and Kline, 2007), but expect that
communication and role switching will likely be im-
portant.
These are straightforward extensions but may ex-
pect a lot of implications to our study. We can even in-
4
His experiments are interpreted as implying that the
retention function may be expressed as an exponential
function. By careful evaluations of Ebbinghous’ data,
Anderson-Schooler (Anderson and Schooler, 1991) reached
the conclusion that the retention function can be better ap-
proximated as a power function, i.e., the probability of re-
taining a memory after time t is expressed as P = At
−b
.
5
(Hanaki, Ishikawa, Akiyama, 2009) studied the con-
vergence of behaviors in a 2-person game, where each
player’s learning of payoffs is formulated in the way of the
present paper but his behavior is formulated as a mechanical
statistical process following the learning literature. Then,
they studied behavior of outcomes in life spans of middle
range. Their approach did not take purely the viewpoint of
IGT in that a player consciously makes a behavior revision
once he has a better understanding of a game situation. Nev-
ertheless, it would give some hint to our further research on
IGT.
troduce more probabilistic factors related to decaying
of long-term as well as short-term memories. How-
ever, more essential extensions are related to the con-
sideration of internal structures of routes and induc-
tive derivations of individual views from experiences.
Simulation studies of those aspects provide a lot of
new directions for research and implications for IGT
as well as the extant game theory.
REFERENCES
Anderson J. R. and L. J. Schooler (1991), Reflections of
the environment in memory, American Psychological
Society 2, 396-408.
Cohen, G., M., (1989), Memory in the Real World,
Lawrence Erlbaum Associates Ltd. Toronto.
Ebbinghous, H., (1964, 1885), Memory: A contribution to
experimental psychology, Mieola, NY: Dover Publi-
cations.
Fudenberg, D., and D.K. Levine, (1998), The Theory of
Learning in Games, MIT Press, Cambridge.
Hanaki, N., R. Ishikawa, E. Akiyama, (2009), Learning
Games, Journal Economic Dynamics & Control 33,
1739-1756.
Harsanyi, J. C., (1967/68), Games with Incomplete Infor-
mation Played by ‘Bayesian’ Players, Parts I,II, and
III, Management Sciences 14, 159-182, 320-334, and
486-502.
Linton, M., (1982), Transformations of memory in every-
day life. In U, Neisser ed. Memory Observed: Rem-
bering in natural contexts. Freeman, San Francisco.
Kaneko, M., (2002), Epistemic Logics and their Game The-
oretical Applications: Introduction. Economic Theory
19, 7-62.
Kaneko, M., and J. J. Kline, (2007), Small and Partial
Views derived from Limited Experiences, University
of Tsukuba, SSM.DP.1166, University of Tsukuba.
Kaneko, M., and J. J. Kline, (2008), Inductive Game The-
ory: A Basic Scenario, Journal of Mathematical Eco-
nomics 44, 1332-1363.
Kaneko, M., and J. J. Kline, (2009), Partial Memories,
Inductively Derived Views, and their Interactions
with Behavior, to appear in Economic Theory, DOI:
10.1007/s00199-010-0519-0
Kaneko, M., and A. Matsui, (1999), Inductive Game The-
ory: Discrimination and Prejudices, Journal of Public
Economic Theory 1, 101-137. Errata: JPET 3, 347.
Malinvaud, E., (1972), Lectures on Microeconomic Theory,
North-Hollond. Amsterdam.
Takeuchi, A., Y. Funaki, M. Kaneko, and J. J. Kline,
(2011), An Experimental Study of Behavior and Cog-
nition from the Perspective of Inductive Game Theory,
SSM.DP.No.1267, University of Tsukuba.
Tulving, E., (1983), Elements of Episodic Memory, Oxford
University Press, London.
Weibull, J. W., (1995), Evolutionary Game Theory, MIT
Press. London.
ASimulationStudyofLearningaStructure-Mike'sBikeCommuting
217