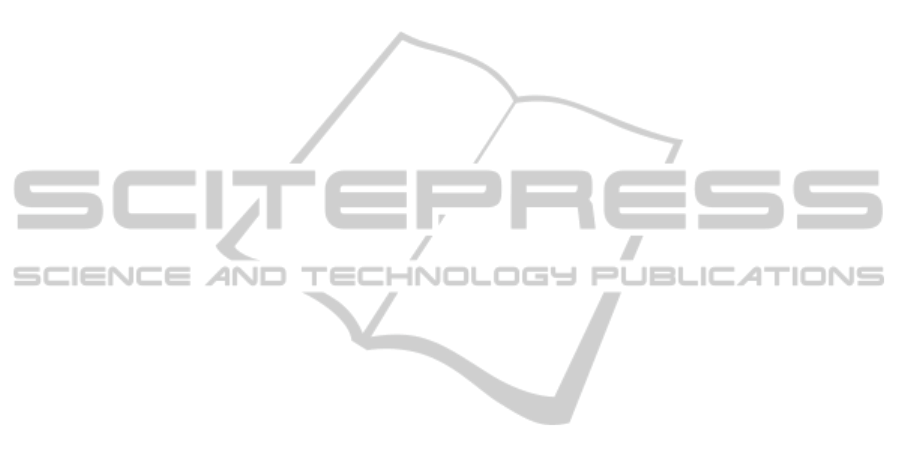
Table 1). Obviously, model 2 could not select the
ER variable because was not a possible input
variable at that time. Therefore, with all the features
available the FIR methodology decides that the three
features with the higher prediction power are COEV,
IC and ER. It is important to mention that these three
variables represent the 50% of the final mark
evaluation (the weighted formula used to compute
the final mark of the didactic planning course is:
MARK = 0.05*MAIL + 0.20*COEV + 0.05*F +
0.05*FCP + 0.20*FC + 0.10*IC + 0.20*ER +
0.15*BR). Notice that there are some variables such
as FC and BR that, by themselves, constitute the
35% of the final mark, but are not included in the
FIR model. This is an important and interesting
result, as it suggests that the information included in
these variables already exists in the selected ones
(COEV, IC and ER). Therefore, these variables are
redundant from the final mark prediction point of
view. The logical rules obtained are comprehensive,
readable and provide useful explanations (not only
assumptions) about the learning behaviour followed
by the students. They have high values in the
standard metrics, i.e. Spec.: 0.78; Sens.: 0.87; Acc.:
0.87. These rules were validated by the course
coordinator, the teachers and the educative experts
of the CECTE, concluding that the obtained results
were consistent with their own perception of the
didactic planning course students’ learning
behaviour.
4 CONCLUSIONS
In this work a framework that provides useful
knowledge in e-learning environments is presented
and used in a real course, i.e. the didactic planning
course. The main objective of the framework is to
alleviate the virtual tutors’ workload and to provide
an effective and valuable feedback to learners. The
fuzzy logic algorithms (FIR, LR-FIR and CR-FIR)
that are the data mining core of the framework are
able to offer valuable knowledge to both, teachers
and students that can be used to enhance course
performance and that opens new possibilities for the
pedagogical and instructional designers, who create
and organize the learning contents
.
The framework is presented in this paper by
means of the didactic planning course. Three
models are inferred during the course. The first one
is obtained at the beginning, when only the personal
information of the student and the first homework is
available. Although the prediction power of this
first model is very limited it offers a first grouping
of the students into potential failing, pass and
excellent students, useful for teachers to give them
feedback. Obviously, the models derived will have
more predictive power as they have access to more
information. Therefore, the knowledge derived from
the subsequent models is more relevant and reliable
in time. This incremental model strategy allows to
provide to the teacher and the student knowledge
about the learning process in real time.
REFERENCES
Castro, F., Vellido, A., Nebot, A. & Mugica, F. (2007).
Applying Data Mining Techniques to e-Learning
Problems. In L. C. Jain, R. A. Tedman & D. K.
Tedman, Evolution of Teaching and Learning
Paradigms in Intelligent Environment. Studies in
Computational Intelligence, 62 (pp. 183-221). Ger.:
Springer-Verlag.
Castro, F., Nebot, A., Múgica, F. (2009). On the extraction
of decision support rules from fuzzy predictive
models. In Revision at Applied Soft Computing (2009).
Klir, G. and Elias, D. (2002). Architecture of Systems
Problem Solving (2nd ed.). NY: Plenum Press.
Minaei-Bidgoli, B., Tan, P. N., Punch, W. F. (2004).
Mining Interesting Contrast Rules for a Web-based
Educational System. In Proceedings of The 2004
International Conference on Machine Learning and
Applications, ICMLA’04. Louisville, KY.
Nebot, A., Mugica, F. and Castro, F. (2009). Causal
Relevance to Improve the Prediction Accuracy of
Dynamical Systems using Inductive Reasoning. Inter.
Journal of General Systems, 331-358, 38 (2).
Romero, C., Ventura, S., De Bra, P., De Castro, C.(2003).
Discovering Prediction Rules in AHA! Courses. In
Proceedings of the User Modelling Conference.
Johnstown, Pennsylvania (pp. 35-44).
Van der Klink, M., Boon, J., Rusman, E., Rodrigo, M.,
Fuentes, C., Arana, C., Barrera, C., Hoke, I., Franco,
M. (2002). Initial Market Study, ALFanet/IST-2001-
33288 Deliverable D72. Open Universiteit Nederland.
URL: http://learningnetworks.org/downloads/alfanet-
d72-initialmarket -studies.pdf.
Van Rosmalen, P., Brouns, F., Tattersall, C., Vogten, H.,
van Bruggen, J., Sloep, P., Koper, R. (2005). Towards
an Open Framework for Adaptive Agent-Supported e-
Learning. Inter. Journal Continuing Engineering
Education and Lifelong Learning 15(3–6) 261-275.
SIMULTECH 2012 - 2nd International Conference on Simulation and Modeling Methodologies, Technologies and
Applications
108