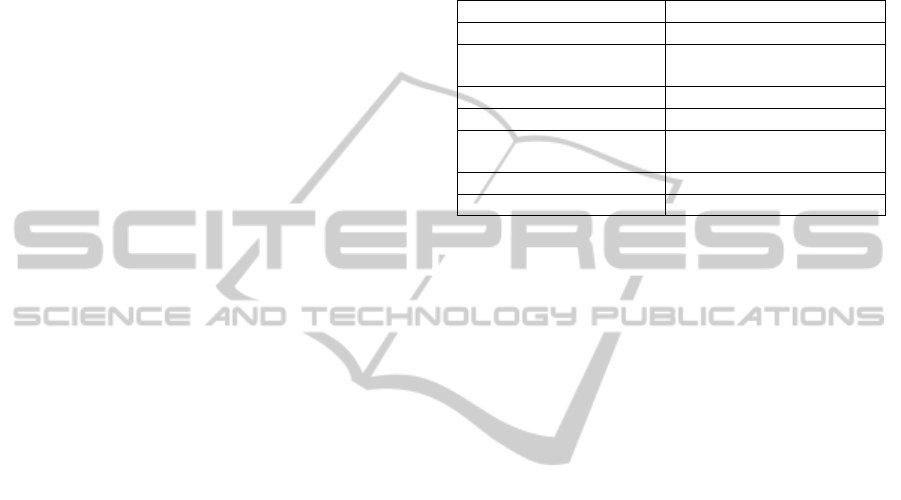
interactions. Agent-based models of human
movement address that individual agent is
autonomous. That is, surpassing conventional
mathematical analysis, one simply instantiates a
population having some distribution of initial states.
Individual agents representing walking human with
initial states are situated in a representation of an
environment and interact with the environment and
themselves, acting out possible macroscopic
emergent behaviours. Agent-based models is
“solved” merely by executing it, where results are
dynamic at each simulation runs.
Modeling pedestrian dynamics gathers more and
more attention because of safety issues in public
facilities. However, current models hardly try to
hypothesize complex passenger decision-making
which would dictate the likelihood of individual
agents entering discretionary areas (Kleinschmidt et
al. 2011). In order to enable more intuitive stochastic
passenger movements, Ma et al. (2011) tried to
incorporate more detailed passenger information into
the simulation model, and therefore provide airport
operators with more realistic passenger flow models.
In this paper, we envisaged an individual agent
decision model aiming to represent intuitive
passenger behaviours. In section 2 we addressed the
significance of studying stochastic passenger
behaviours in air terminals. In Section 3 we propose
Bayesian artificial intelligent for agent decision
model. In section 4 we demonstrate the approach by
have a Bays net simulation case study. In section 5,
we summarize our conclusions and propose some
future areas of research.
2 STOCHASTIC PASSENGER
DYNAMICS
Airport is a complex system. It consists of many
standard sub-systems such as Check-in, Security,
Custom and Boarding. However among the intervals
of them, passenger dynamics are regarded as
stochastic and complex. Passengers might have
difference preferences to use any (if all) in-airport
facilities outside standard processes, for example
duty-free shops, cafe, telephone and bank. We
phrased such events as discretionary activities of
passengers. Since passenger activities outside
standard processing areas account for large
significance regarding safety and airport revenue
(Takakuwa and Oyama 2003, Ma et al. 2011), we
found it is important to study the discretionary
behaviours of passengers.
In order to understand the activities that passengers
use discretionary facilities, we first investigate what
sorts of discretionary facilities an airport terminal
might have and then investigate corresponding impact
factors which have affection on passengers’ choice to
use those facilities (Table 1).
Table 1: Discretionary facilities and corresponding impact
factors.
Discretionary facilities Impact factors
Relaxation facilities Physical tired
Technological self-
service kiosks
Technology preference
Information kiosks New users
Currency service Cash in need
Communication service
Business/entertaining
purposes
Dietary places Hunger level
Shopping places Desire to shopping
Discretionary facilities can be categorised into
the following parts according to utilities. They are
relaxation facilities, technological self-service kiosks,
information kiosks, currency service,
communication service, dietary places and shopping
places. It is true that passenger must use standard
processing facilities to get access through airport.
However, when utilising discretionary facilities,
passengers would usually spend plenty more time.
Ma et al. (2011) use a revised social force model
to simulate the basic motion of passengers in airport
terminals. It enables passenger agents in the
simulation can avoid collision by the repulsive
forces.
Advance path choosing of each passenger agent
are governed by one of the artificial intelligence
decision theories. We choose Bayesian belief
network as the tool for the study in this paper (Kevin
B. K. and Ann E. N., 2011). Bayesian belief
networks is used to generate the possibility that
passengers choose to go to a certain service facility,
which is an innovation comparing to conventional
passenger flow models who pre-assign the
probabilities. We aim to find the relationship
between passenger traits and the possibility of using
certain service facilities. Besides basic traits of
passengers, such as age, gender, we also devise the
advanced traits of passengers in Section 3. They can
be inferred from basic traits within the graph model
of Bayesian networks, and will be used for
individual decision making in our simulation
environment.
Bayesian inference computes the posterior
probability by conditioning, according to the rule of
Bayes. Advanced traits stand for mental preferences
of passengers, which mean that passengers could
SIMULTECH2012-2ndInternationalConferenceonSimulationandModelingMethodologies,Technologiesand
Applications
110