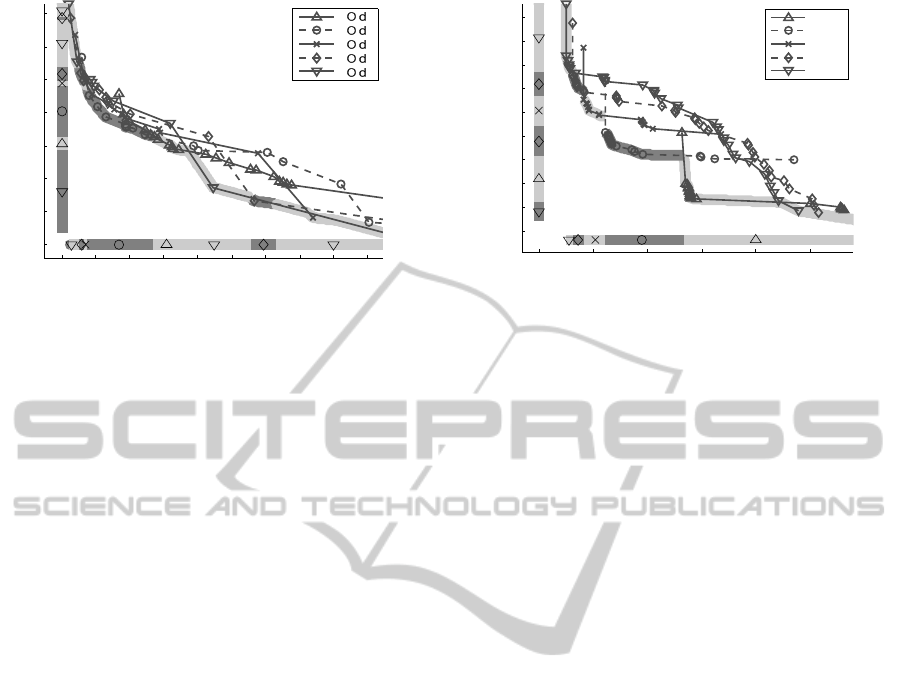
0 0.01 0.02 0.03 0.04 0.05 0.06 0.07 0.08 0.09
5
6
7
8
9
10
11
12
Reconstruction Error
Number of Parameters (log)
0. r er
1. r er
2. r er
3. r er
4. r er
Figure 4: Number of parameters depending on the recon-
struction error for different motion models. The algorithm
segmented to the fifth level was applied to the car sequence.
ideal segmentation level is marked on the pareto front
that is additionally plotted next to the axis. The points
corresponding to a block-wise segmentation for each
l
max
are additionally marked in Fig. 3.
As discussed in Sec. 2 the block-wise segmenta-
tion yields the same reconstruction error as the corre-
sponding graph with ε = 0, but needs less parameters
to encode. From the points on the pareto front we
can conclude that the desired reconstruction quality
or bitrate can be achieved by adapting the segmenta-
tion level. There is no overall best segmentation level,
rather an ideal level for the different requirements.
3.3 Error and Bitrate Depending on the
Model Order
Next we fix the segmentation level l
max
= 5 and com-
pare the differentmodel orders and variousquality pa-
rameters ε. The results on the car sequence are plot-
ted in Fig. 4. Fig. 5 shows the same algorithm on
the hand sequence of the CSAIL database which has
on the one hand a lot of motion discontinuities and
on the other hand large homogeneous regions. Each
curve represents one polynomial motion model and
different ε, starting with ε = 0. Like in Fig. 3 the
pareto front is marked and additionally plotted with
the corresponding model next to the axis. For both
sequences there are regions on the pareto front where
one model gives the best tradeoff between bitrate and
reconstruction quality. Small reconstruction errors re-
fer to larger models.
4 CONCLUSIONS
We provide a qualitative and quantitative analysis for
motion compensation in video coding to find the best
0 0.5 1 1.5 2 2.5
7.5
8
8.5
9
9.5
10
10.5
11
11.5
12
Reconstruction Error
Number of Parameters (log)
0.Order
1.Order
2.Order
3.Order
4.Order
Figure 5: Number of parameters depending on the recon-
struction error for different models. The algorithm seg-
mented to the fifth level was applied to the hand sequence.
tradeoff given compression and loss constraints. It is
possible to judge which segmentation granularity and
motion model complexity best fulfills the coding re-
quirements. The results stress the need for coding al-
gorithms that are adaptive in both the segmentation
level and motion model order.
REFERENCES
Baker, S., Scharstein, D., Lewis, J. P., Roth, S., Black, M. J.,
and Szeliski, R. (2007). A database and evaluation
methodology for optical flow. In Proc. IEEE Conf.
ICCV.
Karczewicz, M., Nieweglowski, J., and Haavisto, P. (1997).
Video coding using motion compensation with poly-
nomial motion vector fields. Signal Processing: Im-
age Communication, 10(1-3):63 – 91.
Lakshman, H., Schwarz, H., and Wiegand, T. (2010). Video
coding with cubic spline interpolation and adaptive
motion model selection. In SPCOM, Conf.
Liu, C., Freeman, W. T., Adelson, E. H., and Weiss, Y.
(2008). Human-assisted motion annotation. In Proc.
IEEE Conf. CVPR, pages 1–8.
Sikora, T. (2005). Trends and perspectives in image and
video coding. IEEE J.PROC., 93(1):6–17.
Sullivan, G. J. and Baker, R. L. (1994). Efficient quadtree
coding of images and video. IEEE J.PROC., 3.
Wiegand, T., Sullivan, G., Bjontegaard, G., and Luthra, A.
(2003). Overview of the h.264/avc video coding stan-
dard. IEEE Transactions on CSVT, 13(7):560 –576.
Zhang, K., Bober, M., and Kittler, J. (1997). Image se-
quence coding using multiple-level segmentation and
affine motion estimation. Selected Areas in Commu-
nications, IEEE Journal on, 15(9):1704 –1713.
SIGMAP2012-InternationalConferenceonSignalProcessingandMultimediaApplications
84