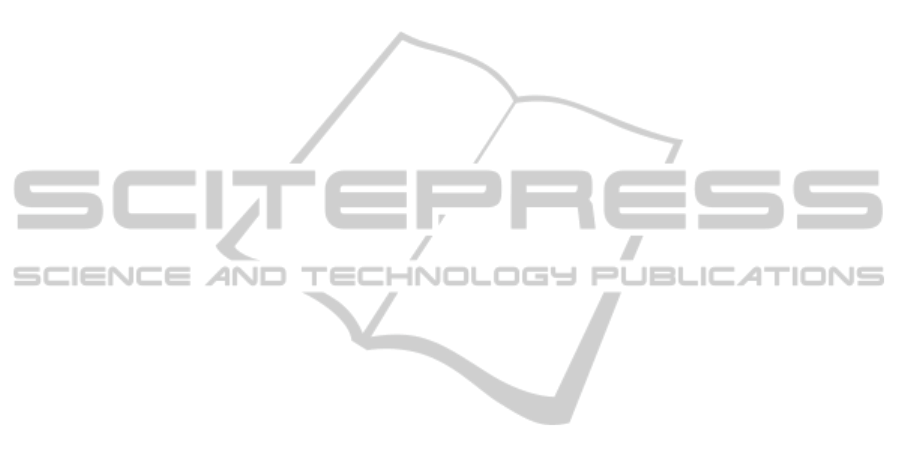
the associated adverse outcomes. From a systems
perspective it is clear that interventions focused on
prescribing behaviour can have implications beyond
the medical aspects of the system, and that a
multifaceted approach which also addresses illicit
use is warranted. The present study serves well to
demonstrate how a systems-level model may help to
evaluate the relative potential efficacy of
interventions to reduce opioid-related overdose
deaths.
ACKNOWLEDGEMENTS
Funding was provided by Purdue Pharma L.P., and
NIDA grant number 1R21DA031361-01A1. The
authors also gratefully acknowledge support from J.
David Haddox, John Fitzgerald, Jack Homer, Lewis
Lee, Louis Macovsky, Dennis McCarty, Lynn R.
Webster, and Aaron Gilson.
REFERENCES
Cavana, R. Y., and Tobias, M. 2008. “Integrative System
Dynamics: Analysis of Policy Options for Tobacco
Control in New Zealand.” Systems Research and
Behavioral Science, 25: 675-694.
Centers for Disease Control and Prevention. 2010.
“Unintentional Drug Poisonings in the United States.”
CDC Issue Brief. Retrieved on September 14, 2010
from
http://www.cdc.gov/HomeandRecreationalSafety/pdf/
poison-issue-brief.pdf
Cicero, T. J., Surratt, H., Inciardi, J. A., and Munoz, A.
2007. “Relationship between Therapeutic Use and
Abuse of Opioid Analgesics in Rural, Suburban, and
Urban Locations in the United States.”
Pharmacoepidemiol Drug Safety, 16(8): 827-840.
Colliver, J. D., Kroutil, L. A., Dai, L., and Gfroerer, J. C.
2006. “Misuse of Prescription Drugs: Data from the
2002, 2003, and 2004 National Surveys on Drug Use
and Health.” DHHS Publication No. SMA 06-4192,
Analytic Series A-28. Rockville, MD: Substance
Abuse and Mental Health Services Administration,
Office of Applied Studies.
Compton, W. M. and Volkow, N. D. 2006. “Major
Increases in Opioid Analgesic Abuse in the United
States: Concerns and Strategies.” Drug and Alcohol
Dependence, 81(2): 103-107.
Degenhardt, L., Hall, W., Warner-Smith, M., and
Lynskey, M. 2004. In M. Ezzati, A. D. Lopez, A.
Rodgers, and C. J. L. Murray (Eds.), “Comparative
Quantification of Health Risks: Global and Regional
Burden of Disease Attributable to Selected Major Risk
Factors, Vol. 1.” pp. 1109-1175. Geneva: Switzerland.
Dunn et al., 2010. “Opioid Prescriptions for Chronic Pain
and overdose.” Annals of Internal Medicine, 152(2):
85-92.
Fishbain et al., 2008. “What Percentage of Chronic
Nonmalignant Pain Patients Exposed to Chronic
Opioid Analgesic Therapy Develop Abuse/Addiction
and or Aberrant Drug-Related Behaviors? A
Structured, Evidence-Based Review.” Pain Medicine,
9(4): 444-459.
Fisher, B., et al., 2004. “Determinants of Overdose
Incidents among Illicit Opioid Users in 5 Canadian
Cities.” Canadian Medical Association Journal,
171(3): 235-239.
Fishman, S. M. et al., 2004. “Regulating opioid
prescribing through prescription monitoring programs:
Balancing drug diversion and treatment of pain.” Pain
Medicine, 5(3): 309-324.
Governale, L. 2008a. “Outpatient Drug Utilization Trends
for Extended Release Morphine Products”
[PowerPoint Presentation]. Retrieved on June 8, 2010
from http://www.fda.gov/ohrms/DOCKETS/ac/08/
slides/2008-4395s2-04-FDA-Governale.ppt
Governale, L. 2008b. “Outpatient Drug Utilization Trends
for Oxycodone Products” [PowerPoint Presentation].
Retrieved on June 8, 2010 from
http://www.fda.gov/ohrms/dockets/ac/08/slides/2008-
4395s1-04-FDA-Governale.ppt
Gureje, O., Simon, G. E., and Von Korff, M. 2001. “A
Cross-National Study of the Course of Persistent Pain
in Primary Care.” Pain, 92: 195-200.
Hall, A. J., et al. 2008. “Patterns of Abuse among
Unintentional Pharmaceutical Overdose Fatalities.”
Journal of the American Medical Association,
300(22): 2613-2620.
Hogarth, R. 1987. “Judgment and Choice” (2
nd
edition).
Chichester, England: John Wiley and Sons.
Højsted, J., and Sjøgren, P. 2007. “Addiction to Opioids in
Chronic Pain Patients: A Literature Review.”
European Journal of Pain, 11: 490–518.
Homer. J. B. 1993. “Projecting the Impact of Law
Enforcement on Cocaine Prevalence: A System
Dynamics Approach.” Journal of Drug Issues, 23(2):
281-295.
Hser, Y., Hoffman, V., Grella, C. E., and Anglis, M. D.
2001. “A 33-year follow-up of narcotics addicts.”
Archives of General Psychiatry, 58: 503-508.
Johnston, L. D., O’Malley, P. M., Bachman, J. G., and
Schulenberg, J. E. December 11, 2007. “Overall Illicit
Drug Use by American Teens Continues Gradual
Decline in 2007.” University of Michigan News
Service: Ann Arbor, MI. Retrieved on August 20,
2010 from www.monitoringthefuture.org.
Jones, A., Homer, J., Murphy, D., Essein, J., Milstein, B.,
and Seville, D. 2006. “Understanding Diabetes
Population Dynamics through Simulation Modeling
and Experimentation.” American Journal of Public
Health, 96: 488-494.
Joranson, D. E., Gilson, A. M., Dahl, J. L., and Haddox, J.
D. 2002. “Pain Management, Controlled Substances,
and State Medical Board Policy: A Decade of
DynamicSimulationofOpioidMisuseOutcomes
405