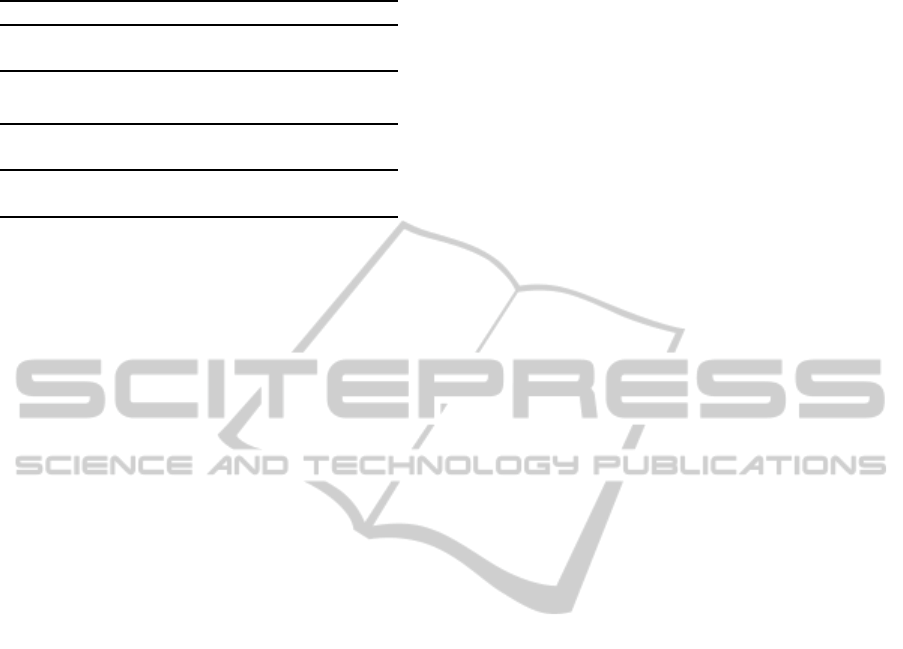
Table 2: Interactive processes.
@ 1 2 3
ˆµ
1
1 0.5 0.52
ˆµ
2
1 0.6 0.59
µ
D
G
1
(x
∗
,h
∗
1
, ˆp
∗
1
) 0.564271 0.514421 0.529412
µ
D
G
2
(x
∗
,h
∗
2
, ˆp
∗
2
) 0.564271 0.614421 0.599412
ˆp
∗
1
0.578193 0.562545 0.567250
ˆp
∗
2
0.551616 0.581684 0.572685
f
1
(x
∗
,h
∗
1
, ˆp
∗
1
) 84.3370 85.4053 85.0840
f
2
(x
∗
,h
∗
2
, ˆp
∗
2
) -311.601 -313.966 -313.258
In MINMAX5( ˆp, ˆµ), it is assumed that the
decision maker sets his/her permissible probabil-
ity levels as ˆp
1
= ˆp
2
= 0.75, and the reference
membership values as ˆµ
1
= ˆµ
2
= 1. Then, the
corresponding Pareto optimal solution is obtained
as f
1
(x
∗
,h
∗
1
,0.75) = 94.0338, f
2
(x
∗
,h
∗
2
,0.75) =
−290.269, µ
˜
G
i
( f
i
(x
∗
,h
∗
i
,0.75)) = 0.11176, i= 1, 2. In
our proposed algorithm, by solving MINMAX4(ˆµ)
for the reference membership values ˆµ
1
= ˆµ
2
=
1, the D
G
-Pareto optimal solution is obtained as
f
1
(x
∗
,h
∗
1
, ˆp
∗
1
) = 84.3370, f
2
(x
∗
,h
∗
2
, ˆp
∗
2
) = −311.601,
ˆp
∗
1
= 0.578193, ˆp
∗
2
= 0.551616 (see the first iteration
of Table 2). This means that a proper balance between
permissible probability levels and the corresponding
objective functions in a fractile optimization model is
attained in membership space.
7 CONCLUSIONS
In this paper, we have proposed an interactive fuzzy
decision making method for multiobjectivefuzzy ran-
dom linear programming problems to obtain a satis-
factory solution from among a Pareto optimal solu-
tion set. In the proposed method, the decision maker
is required to specify the membership functions for
the fuzzy goals of not only objective functions but
also the permissible probability levels. Pareto optimal
concepts called D
p
-Pareto optimal and D
G
-Pareto op-
timal are introduced. The satisfactory solution can be
obtained by updating the reference membership val-
ues and solving the corresponding minmax problem
based on the linear programming technique. At the
optimal solution of MINMAX2(ˆµ) or MINMAX4(ˆµ),
it is expected that a proper balance between permis-
sible possibility levels for a probability maximization
model and permissible probability levels for a fractile
optimization model is attained. In general, in order to
deal with MOFRLP, the decision maker must specify
many parameters in advance. Fuzzy operators such as
the fuzzy decision will lighten his/her burden to spec-
ify such parameters as fixed values.
REFERENCES
Birge, J. R. and Louveaux, F. (1997). Introduction to
Stochastic Programming. Springer.
Charnes, A. and Cooper, W. W. (1959). Chance constrained
programming. Management Science, 6(1):73–79.
Dantzig, G. B. (1955). Linear programming under uncer-
tainty. Management Science, 1(3-4):197–206.
Dubois, D. and Prade, H. (1980). Fuzzy Sets and Systems:
Theory and Applications. Academic Press.
Kall, P. and Mayer, J. (2005). Stochastic Linear Program-
ming Models, Theory, and Computation. Springer.
Katagiri, H., Ishii, H., and Itoh, T. (1997). Fuzzy random
linear programming problem. In Proceedings of Sec-
ond European Workshop on Fuzzy Decision Analysis
and Neural Networks for Management, Planning and
Optimization. Dortmund.
Kwakernaak, H. (1978). Fuzzy random variable-1. Infor-
mation Sciences, 15:1–29.
Lai, V. and Hwang, C. (1992). Fuzzy Mathematical Pro-
gramming. Springer.
Luhandjula, M. and Gupta, M. (1996). On fuzzy stochastic
optimization. Fuzzy Sets and Systems, 81:47–55.
Puri, M. and Ralescu, D. (1986). Fuzzy random variables.
Journal of Mathematical Analysis and Applications,
14:409–422.
Sakawa, M. (1993). Fuzzy Sets and Interactive Multiobjec-
tive Optimization. Plenum Press.
Sakawa, M., Nishizaki, I., and Katagiri, H. (2011). Fuzzy
Stochastic Multiobjective Programming. Springer.
Wang, G.-Y. and Qiao, Z. (1993). Linear programming with
fuzzy random variable coefficients. Fuzzy Sets and
Systems, 57:295–311.
Yano, H. and Matsui, K. (2011). Fuzzy approaches for mul-
tiobjective fuzzy random linear programming prob-
lems through a probability maximization model. In
Lecture Notes in Engineering and Computer Science:
Proceedings of The International MultiConference
of Engineers and Computer Scientists 2011, pages
1349–1354, Hong Kong.
Zimmermann, H.-J. (2011). Fuzzy Sets, Decision-Making
and Expert Systems. Kluwer Academic Publishers,
Boston.
IJCCI2012-InternationalJointConferenceonComputationalIntelligence
328