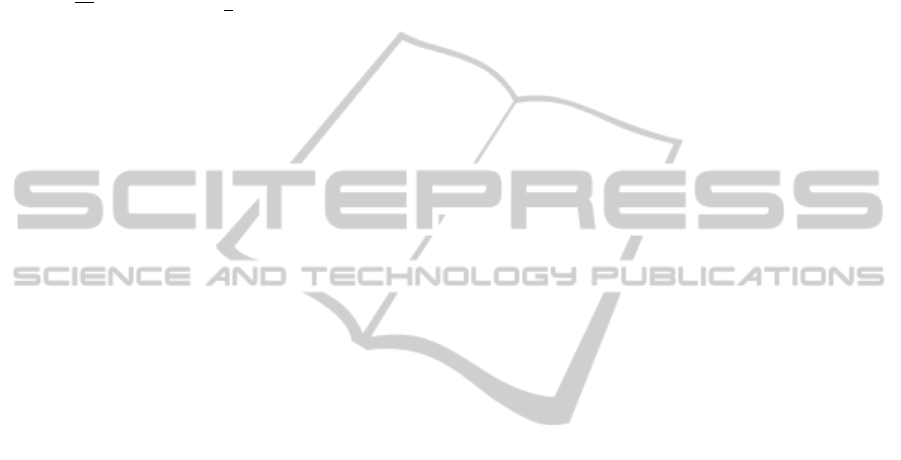
We will now compare our result obtained in
Theorem 1 with a previously reported corresponding
stability result which is given in the following:
Theorem 2 (Ozcan and Arik, 2006). Let f ∈
L . Then, the neural network model (2) is globally
asymptotically robust stable, if
σ = r − (||A
∗
||
2
+ ||A
∗
||
2
+ ||B
∗
||
2
+ ||B
∗
||
2
) > 0
where r =
c
m
µ
M
with c
m
= min(c
i
)and µ
M
= max(µ
i
).
Since ||P||
2
≤||A
∗
||
2
+ ||A
∗
||
2
, ||Q||
2
≤||B
∗
||
2
+
||B
∗
||
2
, Theorem 1 directly implies the result of The-
orem 2.The result of Theorem 2 can be considered a
special case of the result of Theorem 1.
3 CONCLUSIONS
By using a proper Lyapunov functional, we have ob-
tained a easily verifiable delay independent sufficient
condition for the global robust stability of the equilib-
rium point. We havealso compared our result with the
previous corresponding robust stability results pub-
lished in the previous literature, proving that our con-
dition is new and generalizes previously reported re-
sults.
REFERENCES
Arik, S. and Tavsanoglu, V. (2000). On the global asymp-
totic stability of delayed cellular neural networks.
IEEE Trans. Circuits and Syst.I, 47(5):571–574.
Cao, J. and Wang, J. (2003). Global asymptotic stability
of a general class of recurrent neural networks with
time-varying delays. IEEE Trans. Circuits and Syst.I,
50:34–44.
Cao, J. and Wang, J. (2005). Global asymptotic and ro-
bust stability of recurrent neural networks with time
delays. IEEE Trans. Circuits and Syst.I, 52:417–426.
Ensari, T. and Arik, S. (2010). New results for robust sta-
bility of dynamical neural networks with discrete time
delays. Expert Systems with Applications, 27:5925–
5930.
Forti, M. and Tesi, A. (1995). New conditions for global
stability of neural networks with applications to linear
and quadratic programming problems. IEEE Trans.
Circuits Syst., 42(7):354–365.
Li, X. M., Huand, L. H., and Zhu, H. (2003). Global stabil-
ity of cellular neural networks with constant and vari-
able delays. Nonlinear Analysis, 53:319–333.
Liao, T.-L. and Wang, F. C. (2000). Global stability for
cellular neural networks with time delay. IEEE Trans.
on Neural Networks, 11:1481–1485.
Liao, X., Chen, G., and Sanchez, E. N. (2002). Lmi-based
approach for asymptotic stability analysis ofdelayed
neural networks. IEEE Trans. Circuits and Syst.I,
49:1033–1039.
Liao, X. F., Wong, K. W., Wu, Z., and Chen, G. (2001).
Novel robust stability for interval-delayed hopfield
neural. IEEE Trans. Circuits and Syst.I, 48:1355–
1359.
Liao, X. F. and Yu, J. (1998). Robust stability for interval
hopfield neural networks with time delay. IEEE Trans.
Neural Networks, 9:1042–1045.
Mohamad, S. (2001). Global exponential stability in
continuous-time and discrete-time delayed bidirec-
tional neural networks. Physica D, 159:233–251.
Ozcan, N. and Arik, S. (2006). Global robust stability
analysis of neural networks with multiple time delays.
IEEE Trans. Circuits and Syst.I, 53(1):166–176.
Singh, V. (2007). Global robuststability of delayed neural
networks: Estimating upper limit of norm of delayed
connection weight matrix. Chaos, Solitons and Frac-
tals, 32:259263.
Sun, C. and Feng, C. B. (2003). Global robust exponen-
tial stability of interval neural networks with delays.
Neural Processing Letters, 17:107–115.
Wang, K. and Michel, A. N. (1996). On the stability of
family of nonlinear time varying systems. IEEE Trans.
Circuits Syst., 43(7):517–531.
Yi, Z. and Tan, K. (2002). Dynamicstability conditions for
lotka-volterra recurrent neural networks with delays.
hysical Review E, 66:011910.
IJCCI2012-InternationalJointConferenceonComputationalIntelligence
606