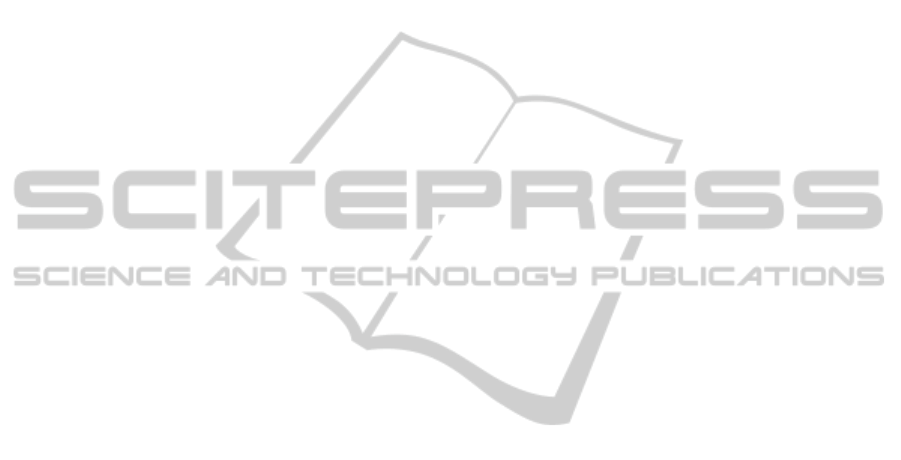
References
1. Baayen, R. H., Davidson, D. J. & Bates, D. M. 2008, "Mixed effects modelling with
crossed random effects for subjects and items.", Journal of Memory and Language, vol. 59,
no. 4, pp. 390-412.
2. Boersma, P. & Weenink, D. 2011, Praat: Doing phonetics by computer [Computer
Program] version 5.3.
3. Cunillera, T., Gomila, A. & Rodriguez-Fornells, A. 2008, "Beneficial effects of word final
stress in segmenting a new language: evidence from ERPs", BMC Neuroscience, vol. 9, no.
23.
4. Cutler, A. & Norris, D. 1988, "The Role of Strong Syllables in Segmentation for Lexical
Access", Journal of Experimental Psychology: Human Perception and Performance, vol.
14, no. 1, pp. 113-121.
5. Hanulikova, A., McQueen, J. M. & Mitterer, H. 2010, "Possible words and fixed stress in
the segmentation of Slovak speech", The Quarterly journal of experimental psychology,
vol. 63, no. 3, pp. 555-579.
6. Kabak, B., Maniwa, K. & Kazanina, N. 2010, "Listeners use vowel harmony and word-
final stress to spot nonsense words: A study of Turkish and French", Laboratory
Phonology, vol. 1, no. 1, pp. 207-224.
7. Kabak, B. & Vogel, I. 2001, "The phonological word and stress assignment in Turkish",
Phonology, vol. 18, no. 03, pp. 315.
8. Kager, R. 1989, A metrical theory of stress and destressing in English and Dutch, Foris,
Dordrecht.
9. Levi, S. V. 2005, "Acoustic correlates of lexical accent in Turkish", Journal of the
International Phonetic Association, vol. 35, no. 01, pp. 73.
10. Mattys, S. L., White, L. & Melhorn, J.F. 2005, "Integration of Multiple Speech
Segmentation Cues: A Hierarchical Framework", Journal of Experimental Psychology:
General, vol. 134, no. 4, pp. 477-500.
11. McQueen, J. M., Norris, D. & Cutler, A. 1994, "Competition in Spoken Word Recognition:
Spotting Words in Other Words", Journal of Experimental Psychology: Learning, Memory,
and Cognition, vol. 20, no. 3, pp. 621-638.
12. Norris, D., McQueen, J. M. & Cutler, A. 1995, "Competition and Segmentation in Spoken-
Word Recognition", Journal of Experimental Psychology: Learning, Memory, and
Cognition, vol. 21, no. 5, pp. 1209-1228.
13. Quené, H. & Koster, M. L. 1998, "Metrical Segmentation in Dutch: Vowel Quality or
Stress?", Language & Speech, vol. 41, no. 2, pp. 185-201.
14. Quené, H. & van den Bergh, H. 2008, "Examples of mixed-effects modeling with crossed
random effects and with binomial data.", Journal of Memory and Language, vol. 59, no. 4,
pp. 413-425.
15. Revithiadou, A., Kaili, H., Prokou, S. & Tiliopoulou, M. 2006, "Turkish accentuation
revisited: Α compositional approach to Turkish stress", Advances in Turkish Linguistics:
Proceedings of the 12th International Conference on Turkish Linguistics, Yağcioğlu, S., A.
Cem Değer, Ö. Koşaner & A. Çeltek (eds.), , pp. 37-50.
16. Sezer, E. 1981, "On non-final stress in Turkish", Journal of Turkish Studies, vol. 5, pp. 61-
69.
17. Sluijter, A.M.C. & van Heuven, V.J. 1996, "Spectral balance as an acoustic correlate of
linguistic stress", The Journal of the Acoustical Society of America, vol. 100, no. 4, pp.
2471-2485.
18. Toro-Soto, J. M., Rodriguez-Fornells, A. & Sebastian-Galles, N. 2007, "Stress placement
and word segmentation by Spanish speakers", Psicologica; Vol 28, No 2 (2007), .
19. Trommelen, M. & Zonneveld, W. 1989, Klemtoon en metrische fonologie, Dick Coutinho,
Muiderberg.
83