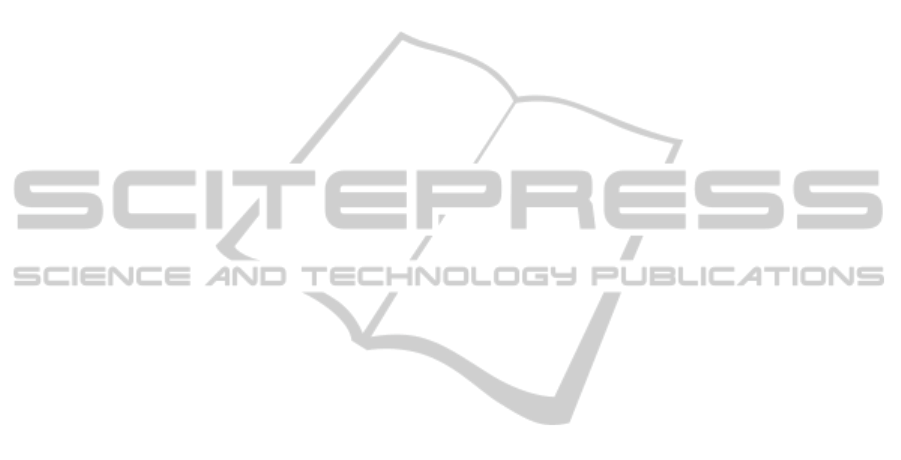
6 Conclusions
This work is, to our knowledge, the first one to use the generation of REs as a task for
learning a new language. As stressed at the beginning, learning a language is more
than just learning to produce grammatically correct forms. As anything can be
expressed via various means (lexical, grammatical, ...), one also needs to learn when
to use what specific resource. And in this respect REs are an interesting application,
as even native speakers do occasionally make 'mistakes', not at the linguistic level, but
at the pragmatic side.
We have extensively drawn on features of the TUNA challenge [3]. For example,
the selection of discriminating features is based on an algorithm that was very
successful within this context. We adapted this algorithm to be able to produce
various acceptable forms for a given target while being able to address in an efficient
and useful way for the learner some of the inherent ambiguities of the target. It would
be interesting now to confront our system with the real world and test it with language
learners. To this end we will certainly revise the icons used, and more importantly,
see what kind of principles could be used to produce expressions that are not only
grammatically correct, but also natural, i.e. corresponding to the forms used by
'normal' people, that is, a majority.
References
1. Dale, R. (1992). Generating Referring Expressions: Constructing Descriptions in a Domain
of Objects and Processes. MIT Press, Cambridge, MA.
2. Dale, R. and Reiter, E. (1995). Computational interpretations of the Gricean maxims in the
generation of referring expressions. Cognitive Science, 19(2):233–263.
3. Gatt, A., Belz, A. & Kow, E. (2009). The TUNA-REG challenge 2009 : Overview and
evaluation results. In ENLG ’09: Proceedings of the 12th European Workshop on Natural
Language Generation, pages 174–182, Morristown, NJ, USA.
4. Grice, P. (1975). "Logic and conversation". In Syntax and Semantics, 3: Speech Acts, ed. P.
Cole & J. Morgan. New York: Academic Press
5. Krahmer, E., van Erk, S. & Verleg, A. (2003). Graph-based generation of referring
expressions. Computational Linguistics, 29(1):53–72.
6. Olson D.R. (1970). Language and Thought: Aspects of a Cognitive Theory of Semantics.
Psychological Review, 77:257-273.
7. Pechmann, T. (1989). Incremental speech production and referential overspecification.
Linguistics, 27, 89-110.
8. Reiter, E.. (1990). Generating descriptions that exploit a user's domain knowledge. In
Current research in natural language generation, Dale, R., Mellish, C. & M. Zock (Eds.).
Academic Press Cognitive Science Series, Vol. 4. Academic Press Professional, Inc., San
Diego, CA, USA, pp. 257-285.
9. van Deemter, K., van der Sluis, I. & Gatt, A. (2006). Building a semantically transparent
corpus for the generation of referring expressions. In INLG ’06: Proceedings of the Fourth
International Natural Language Generation Conference, pages 130–132, Morristown, NJ,
USA.
10. Yousfi-Monod, M. (2010). WebREG: un outil pour apprendre les expressions référentielles,
http://atour.iro.umontreal.ca/drupal7/sites/default/files/publis/WebREG-06-2010.pdf, inter-
nal report, RALI - Université de Montréal
129