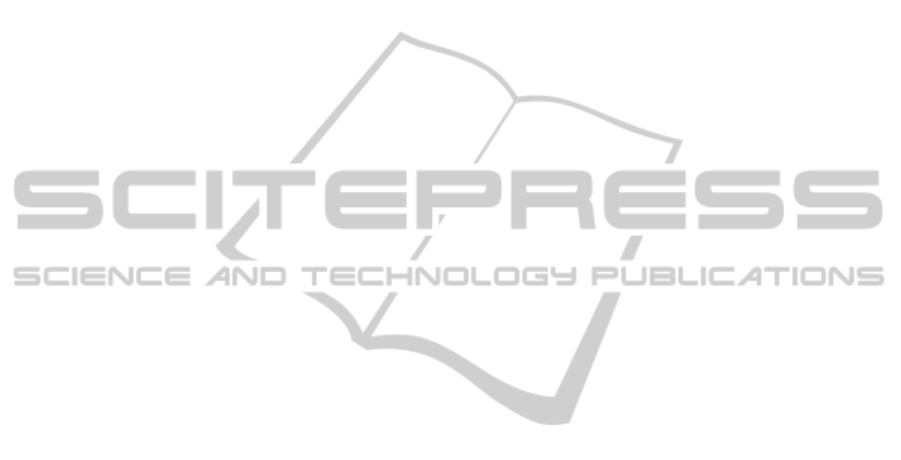
resulting low vale of knowledge sharing. This can
result people will not be able to share knowledge.
The value of knowledge transferability and
knowledge complexity can be put in fuzzy logic
system to explain in high, medium, or low levels.
There are some limitations in our prototype as
follow. In the process of finding transferability and
complexity value we implement sense set which is
extracted from the electronic lexical database
WordNet. By using WordNet, we can only define
ontology concept as a single word which mean it can
only be noun and cannot be adjective, verb, or
adverb. In transferability measurement process, we
only in this paper consider is-a relationship omitting
properties (i.e. object property and datatype
property), constraints, and concept relations e.g.
siblings. Nevertheless, we assess the new knowledge
complexity after finding its transferability
considered above mentioned ontology attributes.
7 CONCLUSIONS AND FUTURE
WORK
We have addressed knowledge complexity and
knowledge transferability as key variables for
knowledge sharing. We then proposed the ontology
based approach which measures ontology
complexity and transferability to correspond to
knowledge complexity and knowledge
transferability respectively. Experimental studies
were given taking Pizza domain and a prototype has
been developed for proof of concept.
For future work a key variable of trust especially
in form of competency trust and benevolence trust
will be incorporated to measure knowledge sharing
in business intelligent applications. Our approach
can be applied to other domains for example e-
commerce and health domains. Future work also
includes a better complexity measurement which
will incorporate depth of concepts i.e. properties
(object property and datatype property) and
constraints, and breadth of concepts i.e. concept
relations e.g. siblings. Comparative evaluation will
also be needed in future work in order to compare
result with other researches in areas of knowledge
sharing measurement and alike.
REFERENCES
Andrew, B.-J., C. S. Veda, et al. (2005). "A semiotic
metrics suite for assessing the quality of ontologies."
Data Knowl. Eng. 55(1): 84-102.
Anthony, M. O., Y. Haining, et al. (2006). "Coupling
Metrics for Ontology-Based Systems." IEEE Softw.
23(2): 102-108.
Anthony, M. O., Y. Haining, et al. (2007). "Indicating
ontology data quality, stability, and completeness
throughout ontology evolution: Research Articles." J.
Softw. Maint. Evol. 19(1): 49-75.
Argote, L., B. Mcevily, et al. (2003). "Managing
knowledge in organizations:An integrative framework
and review of emerging themes." Management Science
49(4): 571-582.
Babcock, P. (2004). Shedding light on knowledge
management. HR Magazine 49: 46-50.
Bakker, M., R. T. A. J. Leenders, et al. (2006). "Is trust
really social capital? Knowledge sharing in product
development projects." The Learning Organization 13:
594−605.
Connelly, C. E. and E. K. Kelloway (2003). "Predictors of
employees' perceptions of knowledge sharing
cultures." Leadership & Organization Development
Journal 24: 294−301.
Cummings, J. N. (2004). "Work groups, structural
diversity, and knowledge sharing in a global
organization." Management Science 50(3): 352−364.
Davenport, T. H. and L. Prusak (2003). Working
knowledge: How organization manage what they
know, Harvard Business School Press.
Dazhou, K., X. Baowen, et al. (2004). A complexity
measure for ontology based on UML. Distributed
Computing Systems, 2004. FTDCS 2004.
Proceedings. 10th IEEE International Workshop on
Future Trends of.
DeLong, D. W. and L. Fahey (2000). "Diagnosing cultural
barriers to knowledge management." Academy of
Management Executive 14(4): 113−127.
Dodgson, M. (1993). "Learning, trust and technological
collaboration." Human Relations 46(1): 77-95.
Doney, P. M. and J. P. Cannon (1997). "An examination
of the nature of trust in Buyer-Seller relationships."
Journal of Marketing 61: 35-51.
Drummond, N., M. Horridge, et al. (2007). "Pizza
Ontologyv1.5" Retrieved May 31, 2010, from
http://www.co-ode.org/ontologies/pizza/2007/02/12/.
Gruber, T. R. (1993). Toward principles for the design of
ontologies used for knowledge sharing. International
Workshop on Formal Ontology in Conceptual
Analysis and Knowledge Representation, Padova,
Italy, Kluwer Academic Publishers, Deventer, The
Netherlands.
Heffernan, T. (2004). "Trust formation in cross-cultural
business to business relationships." Qualitative
research-An international Journal 7: 114-125.
His, I. (2004). Analyzing the Conceptual Coherence of
Computing Applications through Ontological
Excavation PhD Thesis Proposal.
Jap, S. D. (2001). "The strategic role of the saleforce in
developing customer satisfaction across the
relationship." The Journal of Personal Selling and
Sales Management 21: 95-109.
OntologybasedKnowledgeTransferabilityandComplexityMeasurementforKnowledgeSharing
13