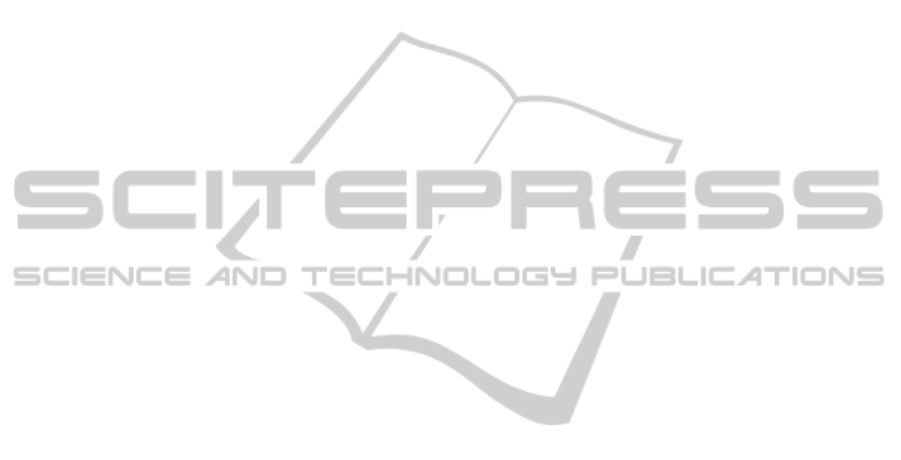
point of view, the experiment is a longitudinal study,
particularly a panel-cohort study (Grau et al., 2004).
Actually, we consider all the students that are in the
last year of their careers in the academic course
2009-2010, but we added all the students that began
its studies in the course 2005-2006 (cohort) although
some of them are not in the last year now because
they have not success during all the years, and so
they will not finish degree on time. Finally, the gen-
eral sampling has 1007 students from 12 faculties
and 25 careers. The cohort is composed with 803
students that began in the academic course 2005-
2006.
The efficiency measure or objective function to
predict efficiency is the dichotomy variable “Finish
degree on time” (Yes/Not). Predictive variables are
some individual student data, previous to the begin-
ning of their university studies, and additionally, the
Faculty and the Career where they are now. It is
important to notice that we used only “epidemiolog-
ical” predictive data and we do not use “clinical”
data, for example, the student performance in the
first/second year. It is a good idea to use only previ-
ous data because it will allow us to obtain a predic-
tion for every student before the first class in his first
course.
In the first step of this methodology it is used the
Association Univariate Analysis based on Crosstabs
Tables. There have been used, particularly, classical
measures as Chi-square, Φ and Cramer’s V (Cramer,
2011) and the classical epidemiological measure of
Relative Risk (Prentice and Farewell, 1986). We try
to build an “integral score of risk to not-finish on
time”. In order to do that, we split each variable
using Decision Trees with the CHAID growing
method: Chi-squared Automatic Interaction Detec-
tion (Decision Trees, 2011). We calculated a statistic
that represents the presence of relative risk weighted
by its relevance, quantified by Cramer’s V.
The multivariate unsupervised Analysis is devel-
oped in two directions. First of all the work is fo-
cused on the predictive data in order to form clusters
of students which have similar previous characteris-
tics. There are used Two-step clusters to determine
the optimal cluster number (with Schwarz’s a Bayes-
ian Information Criterion) and the clusters them-
selves (Bacher et al., 2004). Afterwards is employed
the Categorical Principal Component Analysis
(CATPCA) with the variables to reduce them to two
dimensions (Meulman et al., 2002). In order to vali-
date the clusters and the dimensions found, the re-
sults of both techniques are cross tabulated with the
efficiency measure. But it is important to notice that
unsupervised results are independent from the objec-
tive function and so they could be used if another
objective function is selected. Finally, Decision
Trees in a supervised analysis are used again with
our efficiency measure, but this time we use CRT:
Classification and Regression Trees.
3 KNOWLEDGE DISCOVERING
3.1 Univariate Analysis
The Univariate procedure can be illustrated with the
variable Faculty. The Decision Tree forms three
groups of Faculties that exhibit a Chi-square statistic
with high significance. Students that finish on time
are predominant but in different proportions. We
constructed three dichotomic variables respectively
associated with these nodes and calculate: the Chi-
square statistic, the Cramer’s V (Φ), the Relative
Risk and its 95% confidence interval in their cross
tabulation with the efficiency measure. This proce-
dure is repeated for every possible predicting varia-
ble. From the KD point of view, it is already inter-
esting to know which variables show risk or protec-
tion (negative risk) in some of their categories.
An integral-univariate risk score in any student is
calculated. Essentially, we should sum the present
risk Φ weighted by Cramer’s, standardized and
compare it with a threshold that it is optimized with
a Curve ROC (Fawcett, 2004). The accuracy results
65%. It is not a spectacular result but at least prove
that we can obtain a classification with this Univari-
ate analysis.
3.2 Multivariate Unsupervised Analysis
We separated the unsupervised analysis (clusters)
from data and variables (principal components).
3.2.1 Discovering Clusters
The Bayesian Information Criterion in the Two-Step
Clustering technique found that two is the optimal
number of clusters. Cluster 1 has 309 students
(47.7% of the total analyzed). Cluster 2 has 339
students (52.3%). Due to the missing values (specif-
ically Academic Index in the previous school and
Scale to get admission) 359 students were excluded
in the clustering procedure. It determined the varia-
bles that essentially distinguish the clusters.
So, this cluster analysis allows us to classify the
students according to the predictive variables. This
analysis was repeated also within the cohort and the
results were similar: 2 clusters were formed; the
KDIR2012-InternationalConferenceonKnowledgeDiscoveryandInformationRetrieval
316