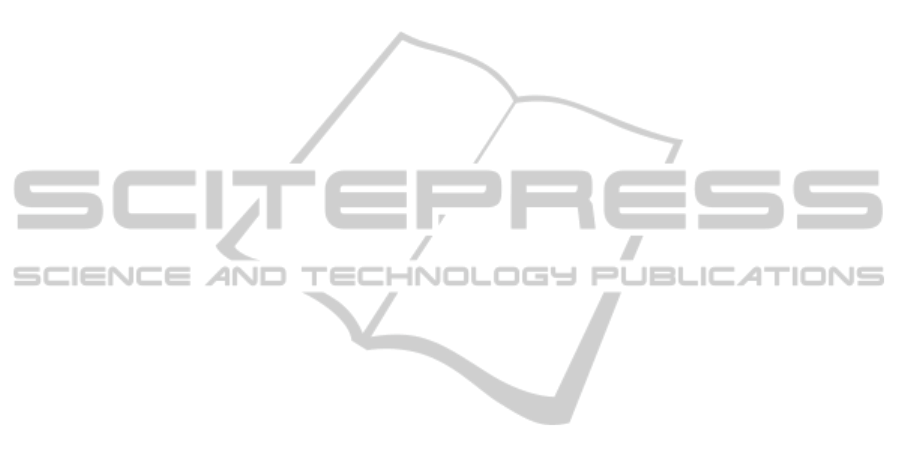
flects a rather close tracking of the respective relia-
bility parameter. Thus, by deciding upon a certain
R
min
, one can tune the success rate to the extreme.
However, there is a clear trade-off between success
rate and matching ratio: e.g., configuring the system
such that it yields a 90% success rate significantly de-
creases the matching ratio to 0.01. Consequently, a
signal is generated only approximately twice per year
and is concerned with only forecasting movements of
the following day. Obviously such a sparse existence
of very short-term signals renders this result virtually
useless in practice, although its quality is outstand-
ing. If instead one would for example use a relia-
bility of 70% (i.e., intentionally accepting occasional
false signals), the resulting success rate would still be
highly satisfactory but, on average, signals are gener-
ated more than once per week. Thus, assuming that
a correct prediction yields profits, the overall gain of
any trading strategy employing this parameter setting
would result in significantly higher gains.
To compare these results to conventional ap-
proaches, the table also lists the results of applying
the pattern descriptions from (Morris, 2006) to the
test data set (i.e., static pattern matching without any
adaptive component) as well as the results of using
these manual descriptions for training the network
(i.e., a supervised learning task). A comparison of
these results clearly shows that the proposed unsuper-
vised method is able to outperform conventional ap-
proaches with respect to both the number of detected
patterns as well as the resulting success rate.
Tests with forecasts of various durations were able
to confirm the statements from (Morris, 2006) regard-
ing the forecasting ability: a forecast three days into
the future with R
min
= 0.7 resulted in a success rate
of roughly 60% while the success rate of a four-day
forecast was not able to exceed 50% significantly and
therefore can hardly be of any use in practice.
5 SUMMARY
For candlestick-based prognoses, well-defined
knowledge regarding significant patterns is required,
as discussed in Section 2. In accordance with
previous work, a probabilistic learning approach is
used to infer prognoses based on a set of known
significant patterns. Rather than merely using the
inference procedures for computing prognoses
based on predefined patterns, the learning process is
extended to an earlier stage of the data processing.
By pursuing the approach of unsupervised pattern
detection, a self-contained knowledge base can be
created which allows for truly adaptive forecasting
systems. Through the ability of directly identifying
latent patterns from training data, the resulting
system is able to cope with securities that may exhibit
varying behavior. Most importantly, the system’s
performance is independent of any manually spec-
ified knowledge and is therefore insusceptible to
any knowledge deficiencies in both quantity and
precision, which are virtually inevitable if a human
domain expert is tasked with specifying his expertise
in a machine-readable form. By replacing the manu-
ally specified pattern sets of the approaches discussed
in Section 3, the presented procedure of identifying
significant candlestick patterns can be combined with
various methods of inferring the actual forecasts
and thus can be used as a an extension for existing
systems. Additionally, it is interesting to note that the
feature selection used by human analysts is obviously
a suitable choice for a machine-learning approach as
well.
REFERENCES
Chou, S., Hsu, H., Yang, C., and Lai, F. (1997). A stock
selection DSS combining AI and Technical Analysis.
Annals of Operations Research, 75:335–353.
Lee, C.-H. L., Liaw, Y.-C., and Hsu, L. (2011). Invest-
ment decision making by using fuzzy candlestick pat-
tern and genetic algorithm. In Fuzzy Systems (FUZZ),
2011 IEEE International Conference on, pages 2696
–2701.
Lee, K. and Jo, G. (1999). Expert system for predicting
stock market timing using a candlestick chart. Expert
Systems with Applications, 16(4):357 – 364.
Lin, X., Yang, Z., and Song, Y. (2011). Intelligent stock
trading system based on improved technical analysis
and Echo State Network. Expert Systems with Appli-
cations, 38(9):11347 – 11354.
Ma;, G. G. C. and Wu, J. (2007). Data Clustering: The-
ory, Algorithms, and Applications. SIAM, Society for
Industrial and Applied Mathematics.
Morris, G. L. (2006). Candlestick Charting Explained:
Timeless Techniques for Trading Stocks and Futures.
McGraw-Hill Professional, 3rd edition.
Ng, W. W. Y., Liang, X.-L., Chan, P. P. K., and Yeung, D. S.
(2011). Stock investment decision support for Hong
Kong market using RBFNN based candlestick mod-
els. In Machine Learning and Cybernetics (ICMLC),
2011 International Conference on, volume 2, pages
538 –543.
Nison, S. (1991). Japanese Candlestick Charting Tech-
niques: A Contemporary Guide to the Ancient Invest-
ment Techniques of the Far East. New York Institute
of Finance.
Nison, S. (2003). The Candlestick Course. Wiley & Sons.
Tsay, R. S. (2010). Analysis of Financial Time Series (Wi-
ley Series in Probability and Statistics). Wiley, 3rd
edition.
KDIR2012-InternationalConferenceonKnowledgeDiscoveryandInformationRetrieval
150