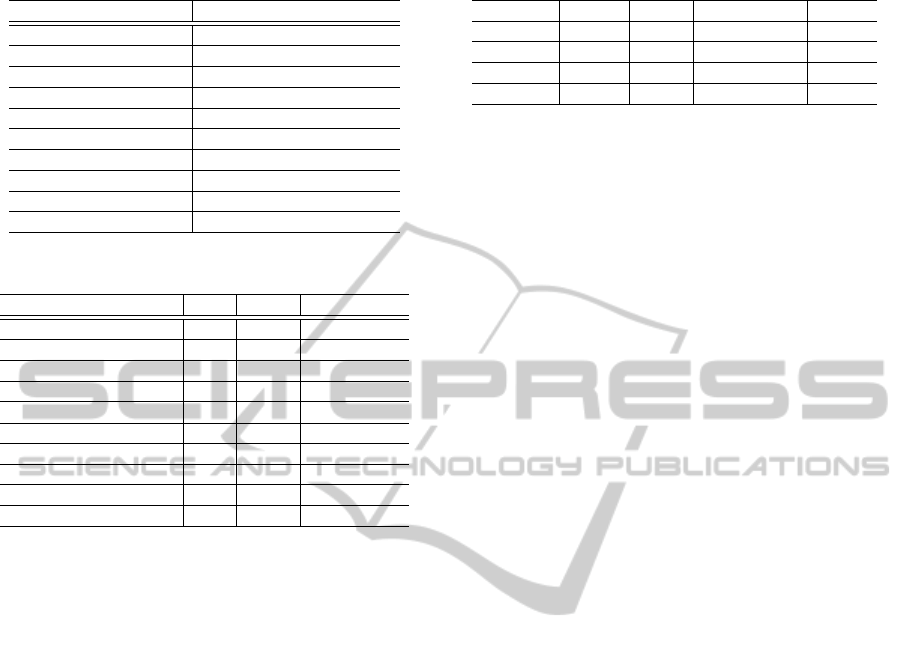
Table 1: Topics assigned to categories in the TDT.
Category Topics
Elections U.S. Mid-Term Elections
Scandals/hearings Olympic Bribery Scandal
Legal/criminal Pinochet Trial
Natural disasters Hurricane Mitch
Accidents Nigerian Gas Line fire
Violence or war Car Bomb in Jerusalem
Science and discovery Leonid Meteor Shower
Finances IMF Bailout of Brazil
New laws Anti-Doping Proposals
Sports Australian Yacht Race
Table 2: The number of candidate senses.
Category Doc Total Cand.(%)
Elections 518 3,837 1,970(51.3)
Scandals/hearings 108 3,566 1,775(49.7)
Legal/criminal 860 3,396 1,805(53.1)
Natural disasters 286 3,496 1,851(52.9)
Accidents 82 2,828 916(32.3)
Violence or war 234 3,495 1,741(49.8)
Science and discovery 186 3,955 1,969(49.7)
Finances 540 3,428 1,945(56.7)
New laws 7 3,304 1,646(49.8)
Sports news 326 3,428 1,957(57.0)
3 EXPERIMENTS
We used the TDT3 corpus which comprises a set of
eight English news sources collected from October to
December 1998. It consists of 34,600 stories. A set
of 60 topics are defined for evaluation in 1999, and
another 60 topics for evaluation in 2000. Of these
topics, we used 78 topics, each of which is classified
into 10 categories. Table 1 illustrates categories and
some examples of topics assigned to these categories.
3.1 Assignment of Domain-specific
Senses
We divided TDT documents into two: training and
test data in text classification. The size of training data
for each category is two-third of documents, and the
remaining is test data. All documents were tagged by
Tree Tagger (Schmid, 1995). For each category, we
collected the topmost 500 noun words with high TF-
IDF weight from the TDT3 corpus. We used Word-
Net 3.0 to assign senses. Table 2 shows the number
of training documents, the total number of senses, and
the number of candidates senses (Cand.) that the clas-
sification accuracy of each category was higher than
the result without word replacement. We used these
senses as an input of the MRW model.
Table 3: The result against SFC resource.
Cat ADSS SFC SFC & TDT Recall
Finances 390 125 81 0.648
New law 358 1,628 193 0.437
Science 389 671 176 0.699
Sports 395 1,947 8 1.000
There are no existing sense-tagged data for these
10 categories that could be used for evaluation.
Therefore, we selected a limited number of words
and evaluated these words qualitatively. To this end,
we used the Subject Field Codes (SFC) resource
(Magnini and Cavaglia, 2000) annotating WordNet
2.0 synsets with domain labels. The SFC consists of
115,424 words assigning 168 domain labels with hi-
erarchy. It contains ”finances”, ”laws”, ”science” and
”sports” labels. We used these four labels and its chil-
dren labels in a hierarchy, and compared the results
with SFC resource. The results are shown in Table
3. ”ADSS” shows the number of senses assigned by
our approach. ”SFC” refers to the number of senses
appearing in the SFC resource. ”SFC & TDT” de-
notes the number of words (senses) appearing in both
SFC and the TDT corpus. We note that the corpus
we used was TDT corpus, while SFC assigns domain
labels to the words appearing in the WordNet. There-
fore, we used recall as the evaluation measure where
it refers to the number of senses matched in our ap-
proach and SFC divided by the total number of senses
appearing in both SFC and TDT. ”Recall” in Table
3 refers to the best performance among the varying
number of senses according to the rank scores. As we
can see from Table 3 that word replacement improved
text classification performance as the former was 0.06
F-score, while that of the latter was only 0.01. One
reason is the length of the gloss text in the WordNet;
the average length of gloss text assigned to ”law” was
5.75, while that for ”sports” was 8.96. The method of
assigning senses depends on the size of gloss text in
the WordNet. Efficacy can be improved if we can as-
sign example sentences to WordNet based on corpus
statistics. This is a rich space for further exploration.
It is interesting to note that some senses of words
that were obtained correctly by our approach did not
appear in the SFC resource because of the difference
in WordNet version, i.e., we used WordNet 3.0 and
the TDT corpus for ADSS, while SFC is based on
WordNet 2.0. Table 4 illustrates some examples ob-
tained by our approach but that did not appear in the
SFC. These observationssupport the usefulness of our
automated method.
For evaluating subject detection, we randomly se-
lected one topic for each category, and manually
checked whether the words assigning domain-specific
senses are the subject or not. Table 5 shows the re-
TopicandSubjectDetectioninNewsStreamsforMulti-documentSummarization
169