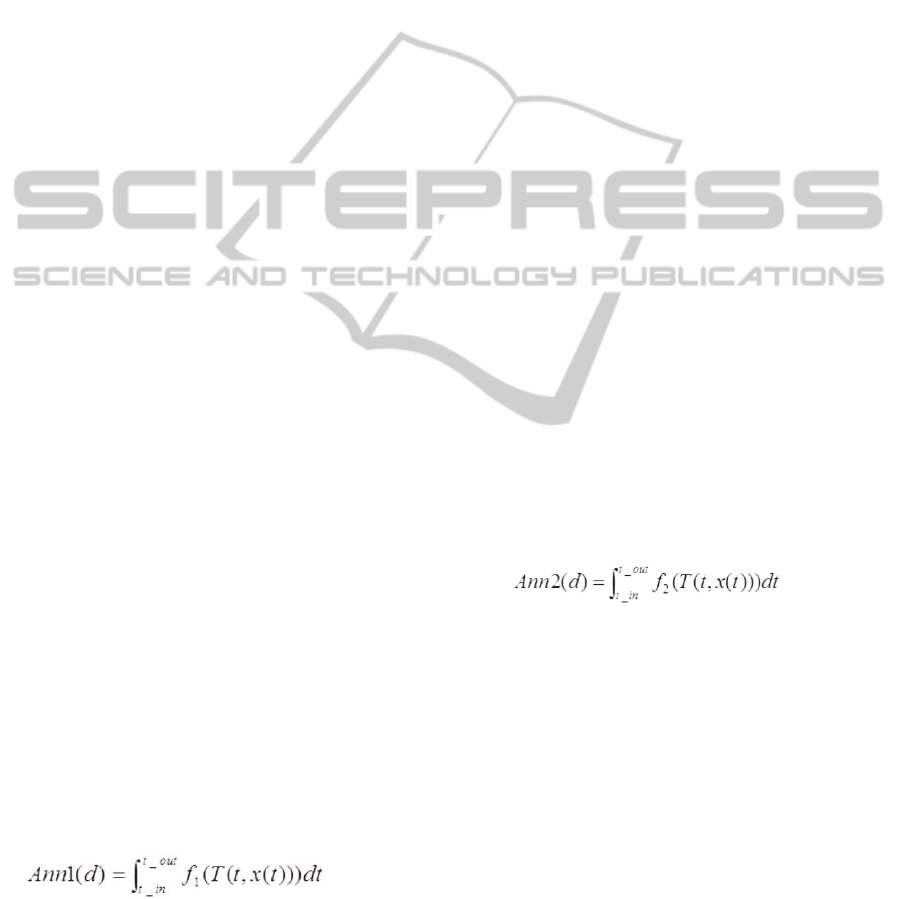
passage of the coil in each zone of the furnace
applying the model of heating of the annealing
furnace explained in Par. 4. Control of the heating
furnace is the calculation of heating points to be
permanently transferred to each area of the furnace,
with the aim of accomplishing the following: Attain
a decrease in temperature as close as possible to the
desired temperature, accommodation of operating
conditions according to the ratio variations on the
temperature of the furnace, minimize the
consumption of energy by optimizing heating
methods, classification of coils according to their
behaviour in the oven and prediction of the furnace
operating points based on initial conditions.
Data Mining tools and multivariate statistics are
useful when there is a significant historical volume
and good quality (Chapple, 2002). The thermal
energy received by each of the coil while in the
furnace can be calculated (Spinola, 2004). To do
this, the temperatures applied to each coil in each
area, top and bottom, are obtained from the data files
where they have been continuously registered. The
next step will be to rank the coils, depending on the
energy received as “bad annealed” or “well annealed
according to their characteristics, size and type of
steel. With all of this a model of annealing will be
made and a table of values of temperature and speed
set points will be obtained. Studying coil population
using ANN and classification to obtain an improved
model it is possible to reduce annealing transition
time between coils of different steel grades and
dimensions, optimizing the thermal transitions of the
different types of coils.
4 HOW TO OBTAIN THE
ANNEALING VALUE
In order to make easy the analysis and visualization
of the annealing of a complete coil, the process
consists in integrating the heat-up curve of all of the
points of the heated material along the furnace and
determines the temperature set points of the other
points of the heated coil. To summarize heating
information of each element we define the function
Ann1(d) as the integral in Eq 1.
(1)
Where d is the position of the coil element, t_in and
t_out are the time when the coil element enters and
exits the furnace and T(t,x(t)) is the temperature at
time t and position x(t) along the trajectory of the
element inside the furnace.
Let Tr be the annealing temperature. Function f1
is 0 below Tr and it is equal to T above it, as the
annealing is performed above this specific
temperature. If the temperature is below this value,
the coil is heated, but the grain structure is not
recrystallized and the contribution to the Ann1 value
is null. The physical dimension of Ann1(d) is
temperature by time (ºC* sec), and represents the
amount of effective thermal energy received by the
coil element d. But the function Ann1 depends
deeply on the critical value Tr. Although only high
temperatures recrystallize the stainless steel,
possibly there is not such a key value and
temperatures just below Tr also affect the metal. For
this reason an alternative function, Ann2, was
proposed.
It integrates function f2. We chose an interval
Tm – Ta which should contain the critical value Tr.
The function slope above Ta is m2 which should be
0 or slightly above. This formula has a different
physical meaning. If we choose m2=0 and a coil
element is heated inside the furnace with a constant
temperature greater than Ta, Ann2(x) will be the
total time the element has been inside the furnace.
The time the element is exposed to a temperature
below Tm does not count at all, but the time the coil
is heated with a temperature from Tm to Ta is
proportionally counted. So, this function calculates
the annealing compensated time an element stays in
the furnace. If we choose m2>0, temperatures higher
than Ta overcompensate the annealing time, as the
annealing process speed and the temperature are
related. The parameter m2 reflects that fact (Spinola,
2004).
(2)
5 TOOLS AND METHODS
In order to classify the annealing of stainless steel
coils, a kind of neural network, self-Organizing
Maps (SOM) has been used. The software tools used
to implement the Classification program are Matlab
7.0 and The Self-Organizing Map Program Pakage
by Kohonen, that implements the techniques of
neural networks we need (Kangas, 1997). The SOM
consists of a two-dimensional lattice that contains a
number of neurons (Kohonen, 1992).
The Gaussian function has been chosen as the
neighbourhood function and the rectangular
structure as the topology of the map as we can see in
the figure 2. A prototype vector is associated with
IJCCI2012-InternationalJointConferenceonComputationalIntelligence
608