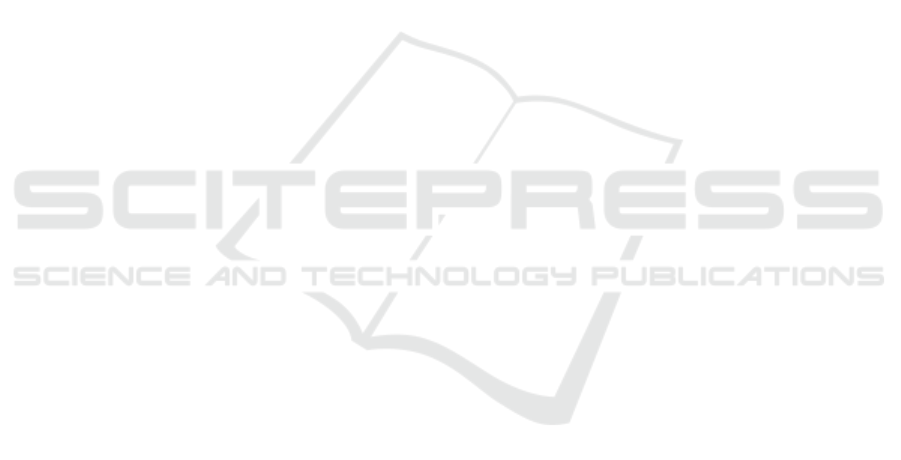
Prediction of the Behaviours by the Prismatic Beams
with Polypropylene Fibers under High Temperature Effects
through Artificial Neural Networks
Fatih Altun and Tamer Dirikgil
Department of Civil Engineering, Erciyes University, Kayseri, Turkey
Keywords: Polypropylene Fiber, Concrete, High Temperature, Flexure Strength, Multilayer Perceptron, Radial Basis
Function Neural Network.
Abstract: In order to improve the mechanical qualities of a concrete, various kinds of fibers are added to the concrete.
In the studies, polypropylene (PP) fibers are employed as a fiber type. It has a significant place in the
researches that PP fibers not only improve the mechanical qualities of the concrete under normal
temperatures, but also prevents the bursting of the concrete with the internal vapour compression under high
temperatures. The distributions and locations of the fibers in the concrete and the variables employed for
experimental proceedings affect the mechanical results. This makes it difficult to link the obtained results to
each other. In order to establish a complicated link, it is inevitable to create a learning mechanism. In this
study, multilayered perceptrons (MLP) and radial basis function artificial neural network (RBFNN) models
were used and their flexure strengths were sought to be predicted. Both of the neural network models put in
a successful performance and enabled the prediction of the experimental results with a satisfying
approximation.
1 INTRODUCTION
It is practically not possible to empirically state the
effects of locations of the fibers, homogeneity of the
fibers and the different temperatures on the
mechanical qualities of a concrete. Therefore, it is
complicated to predict the behaviours of the concrete
with similar qualities by the data in hand. To model
and anticipate the complicated systems depending
on the input-output data and/or unknown behaviours,
methods to develop mathematical models in various
fields of civil engineering have been employed
(Astrom and Eykhoff, 1971).
In this study, bending tensile strengths of the
concrete with PP fiber addition are predicted using
the multilayered perceptron neural network and
radial basis function artificial neural network
models. For both of the models, the same input,
validation, and testing data were used. Addition of
split tensile strength obtained through experimental
studies to the variables on which experimental
proceedings were applied in the entry parameters
made it more difficult to link between the input-
output data. Ultimately, the performance of the two
different artificial neural network models was found
to be satisfying.
2 EXPERIMENTAL PROCEDURE
The samples produced during the experimental study
comprised of members with 40 MPa characteristic
compressive strength and in the C35/45 concrete
class (
TS EN 206/1, 2002). Samples were cured in
periods of 7,28 and 90 days and made ready. In
addition to the room temperature (24.5 ºC), five
more temperature effects of 100 ºC, 200 ºC, 400 ºC,
600 ºC and 800 ºC were employed. The samples
under the room temperature were assessed as the
reference samples for the other temperature effects.
Following the heating period, the temperature in the
oven was left to cool by itself with its lid closed until
it decreased to room temperature in order to keep the
experimental samples from exposing to the effects of
abrupt temperature changes. Table 1 and Table 2
shows the 216 cylinder and prismatic samples
according to each fiber type, volumetric fiber ratio,
and cure period and temperature value.
611
Altun F. and Dirikgil T..
Prediction of the Behaviours by the Prismatic Beams with Polypropylene Fibers under High Temperature Effects through Artificial Neural Networks.
DOI: 10.5220/0004114006110615
In Proceedings of the 4th International Joint Conference on Computational Intelligence (NCTA-2012), pages 611-615
ISBN: 978-989-8565-33-4
Copyright
c
2012 SCITEPRESS (Science and Technology Publications, Lda.)