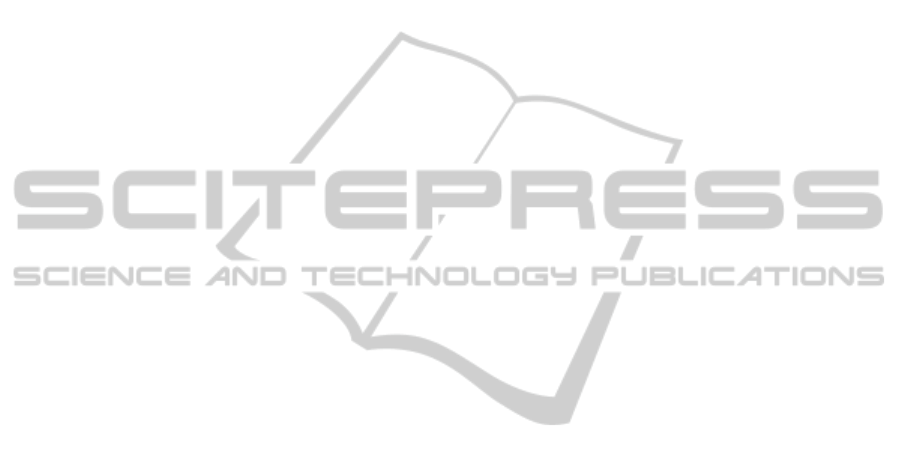
sual perception is subjective and difficult to quantify.
Relevance feedback is another important step to be
considered. Different similarity functions associated
to relevance feedback may enhance the effectiveness
of image retrieval, as the user’s preferences are more
rapidly met. The method’s ability to retrieve images
of the same group may qualify image retrieval as a po-
tential knowledge discovery tool. It implements new
levels of supporting environments and opens new per-
spectives to exploratory research in image databases.
ACKNOWLEDGEMENTS
This work was supported by FAPEMIG (PPM
00416/11), CNPq (481989/2010-2, 301907/2010-2)
and INCT-MM (FAPEMIG: CBB-APQ-00075-09 /
CNPq 573646/2008-2. The author is grateful to the
University of Pennsylvania for sharing the callosum
data.
REFERENCES
Attalla, E. and Siy, P. (2005). Robust shape similarity re-
trieval based on contour segmentation polygonal mul-
tiresolution and elastic matching. Pattern Recogn,
38(12):2229–2241.
Biswas, S., Aggarwal, G., and Chellappa, R. (2010). An
efficient and robust algorithm for shape indexing and
retrieval. IEEE T Multimedia, 12(5):372–385.
Bookstein, F. L. (1997). Landmark methods for forms with-
out landmarks:morphometrics of group differences in
outline shape. Med Image Anal, 1(3):225–243.
Cootes, T. and Taylor, C. J. (1999). A mixture model for
representing shape variation. Image Vision Comput,
17(8):567–574.
Gee, J. C. (1999). On matching brain volumes. Pattern
Recogn, 32:99–111.
Golland, P., Grimson, W. E. L., Shenton, M. E., and Kiki-
nis, R. (2001). Deformation analysis for shape based
classification. LNCS, 2082:517–530.
Gonzalez, R. C. and Woods, R. E. (2002). Digital Image
Processing. Prentice-Hall, Upper Saddle River.
Iakovidis, D. K., Pelekis, N., Kotsifakos, E. E., Kopanakis,
I., Karanikas, H., and Theodoridis, Y. (2009). A pat-
tern similarity scheme for medical image retrieval.
IEEE T Inf Technol B, 13(4):442–450.
Le Briquer, L. and Gee, J. C. (1997). Design of a Statistical
Model of Brain Shape. LNCS, 1230:477–482.
Lew, M., Sebe, N., Djeraba, C., and Jain, R. (2006).
Content-based multimedia information retrieval: State
of the art and challenges. ACM T Multimedia Comput,
2(1):1–19.
Lew, M. S. (2010). Principles of Visual Information Re-
trieval. Spring-Verlag, London.
Machado, A., Gee, J. C., and Campos, M. (2004). Structural
shape characterization via exploratory factor analysis.
Artif Intell Med, 30:97–118.
Mallik, J., Samal, A., and Gardner, S. L. (2010). A content
based image retrieval system for a biological speci-
men collection. Comput Vision Image Und, 114:745–
757.
Mohammed, A. A., Minhas, R., Wu, Q., and Sid-Ahmed,
M. A. (2011). Human face recognition based on mul-
tidimensional pca and extreme learning machine. Pat-
tern Recogn, 44:2588–2597.
Muller, H., Michoux, N., Bandon, D., and Geissbuhler, A.
(2004). A review of content-based image retrieval sys-
tems in medical applications-clinical benefits and fu-
ture directions. Int J Med Inform, 73(1):1–23.
Oliveira, J., Machado, A., Chavez, G., Lopes, A., Deserno,
T., and Arajo, A. A. (2010). Mammosys: a content-
based image retrieval system using breast density pat-
terns. Comput Meth Prog Bio, pages 289–297.
Reyment, R. and J
¨
oreskog, K. (1996). Applied Factor Anal-
ysis in the Natural Sciences. Cambridge University
Press, Cambridge.
Sclaroff, S. and Pentland, A. (1995). Modal matching for
correspondence and recognition. IEEE T Pattern Anal,
17(6):545–561.
Shu, X. and Wu, X. (2011). A novel contour descriptor
for 2d shape matching and its application to image re-
trieval. Image Vision Comput, 29:286–294.
Xie, J., Heng, P., and Shah, M. (2008). Shape matching
and modeling using skeletal context. Pattern Recogn,
41(5):1756–1767.
Xu, C. J., Liu, J. Z., and Tang, X. (2009). 2d shape
matching by contour flexibility. IEEE T Pattern Anal,
31(1):180–186.
Zhang, D. S. and Lu, G. J. (2004). Review of shape repre-
sentation and description techniques. Pattern Recogn,
37:119.
KDIR2012-InternationalConferenceonKnowledgeDiscoveryandInformationRetrieval
180