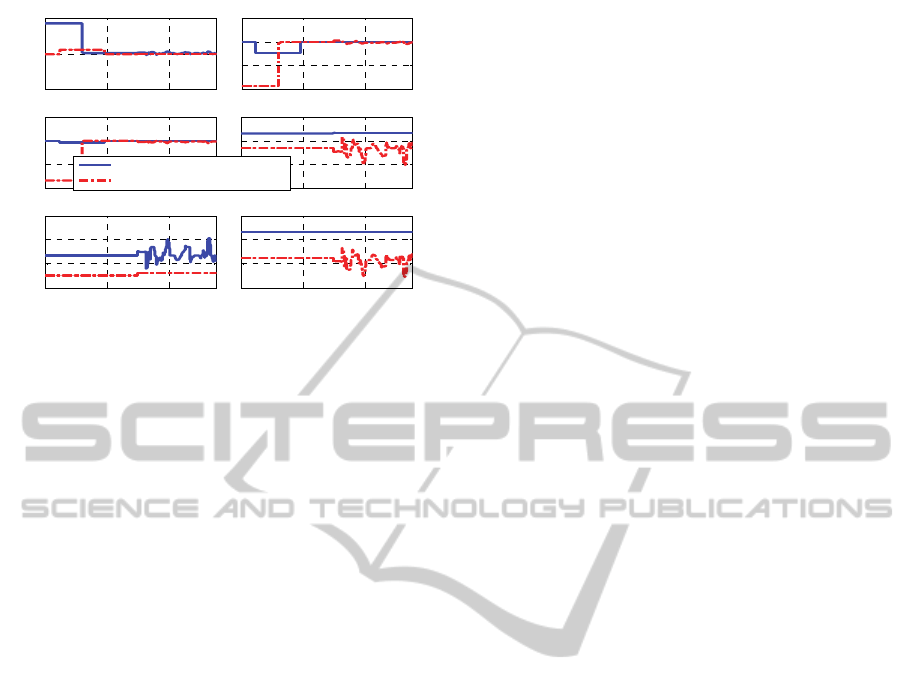
Figure 6: The feedforward and feedback control of the
most representative joints in the system.
7 CONCLUSIONS
The core of this paper was the implementation of
fuzzy controller along with estimation of kinematic
coefficients to formulate the feedback for a robotic
arm with antagonistically coupled compliant drives.
Since the suggested control algorithm relies on
the experience and fuzzy logic, it is expected to be
applicable to a wider class of robots. The only
modification would be different training data –
experience base should be customized for the
specific robot skills. Since the experience acquiring
stage in feedforward phase is time consuming,
further research can explore solution to speed up this
process. As our control depends on base resolution,
the future work would consider developing of more
sophisticated method to increase precision of the
control algorithm (e.g. to make more complex fuzzy
engine).
ACKNOWLEDGEMENTS
The research leading to these results has received
funding from the European Community's Seventh
Framework Programme FP7/2007-2013 - Challenge
2- Cognitive Systems, Interaction, Robotics - under
grant agreement no. 231864 - ECCEROBOT; and
partly by the Serbian Ministry of Science and
Technological Development under contracts 35003
and 44008.
REFERENCES
Chhabra, M., Jacobs, A., 2008. Learning to combine
motor primitives via greedy additive regression. The
Journal of Machine Learning Research. vol. 9. pp.
1535-1558.
Gonzalez-Olvera, M., Rodriguez-Morales, A., Tang, Y.,
2009. Black-box modeling of a 2-dof manipulator in
the image plane using recurrent neurofuzzy networks.
In Proc. of the IEEE Int. Conf. on Decision and
Control, pp. 8440-8445.
Haruno, M., Wolpert, D., Kawato, M., 2001. MOSAIC
model for sensorimotor learning and control. Neural
Computation. vol. 13. pp. 2201 – 2220.
Holland, O., Knight, R., 2006. The anthropomimetic
principle. In Proc. of The Symposium on Biologically
Inspired Robotics edited by J. Burn and M. Wilson.
Ljung, L., 2001. Black-box models from input-output
measurements. Proceedings of the 18th IEEE
Instrumentation and Measurement Technology
Conference. vol.1. pp. 138 - 146.
Potkonjak, V., Svetozarevic, B., Jovanovic, K., Holland,
O., 2010. Biologically inspired control of a compliant
anthropomimetic robot. In Proc. of the IASTED Int.
Conf. on Robotics and Applications. pp. 182 – 189.
Potkonjak, V., Svetozarevic, B., Jovanovic, K., Holland,
O., 2012. The puller-follower control of compliant and
noncompliant antagonistic tendon drives in robotic
system, International Journal of Advanced Robotic
Systems, vol. 8, pp 143-155.
Rivals, I., Personnaz, L., 1996. Black-box modeling with
state-space neural networks. Neural Adaptive Control
Technology. vol. 15. pp. 237-264.
Stulp, F., Fedrizzi, A., Zacharias, F., Tenorth, M.,
Bandouch, J., Beetz, M., 2009. Combining analysis,
imitation, and experience-based learning to acquire a
concept of reachability in robot mobile manipulation.
In Proc. of the IEEE Int. Conf. on Humanoid Robotics.
pp. 161-167.
Tal’nov, A., Serenko, S., Strafun, S., Kostyukov, A., 1999.
Analysis of the electromyographic activity of human
elbow joint muscles during slow linear flexion
movements in isotorque conditions. Neuroscience. vol.
90. pp. 1123-1136.
Van Mulders, A., Schoukens, J., Volckaert, M., Diehl, M.,
2010. Two nonlinear optimization methods for black
box identification compared. Automatica. vol. 46. pp.
1675–1681.
Zadeh, L., 1965. Fuzzy sets. Information and Control. vol.
8, pp. 338–353.
0 2 4
-5
0
5
right shoulder X joint
0 2 4
-10
-5
0
5
right shoulder Y joint
0 2 4
-10
-5
0
5
right elbow Y joint
Control inputs [V]
0 2 4
-1
-0.5
0
0.5
right elbow Z joint
0 2 4
-1
-0.5
0
0.5
right wrist Y joint
0 2 4
-0.5
0
0.5
1
right wrist X joint
Time [s]
Agonist control signal
Antagonist control signal
IJCCI2012-InternationalJointConferenceonComputationalIntelligence
394