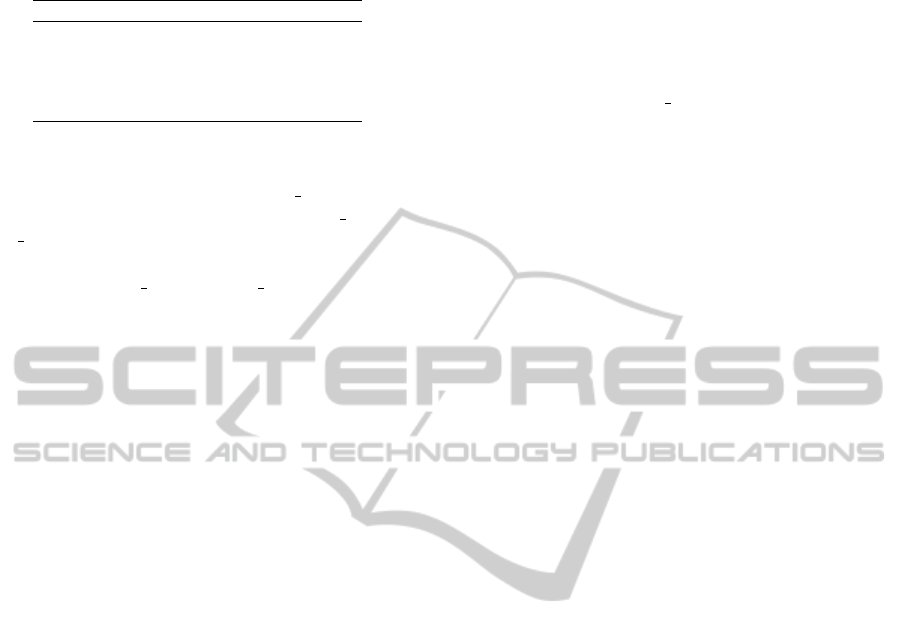
Table 2: Patterns extracted using Q
f
⇔ {conf ≥ 0.8 ∧
coh ≥ 0.8∧ conf
B
≥ 0.5} as selection criteria.
k=0.02 s k=0.03 s k=0.04 s
hL,M,Ni hL,M,Ni hL,M,Ni
hG,H,G,Hi hG,H,G,Hi hM,N, M,Ni
hE,F,G,Hi hF,G,F, Gi hE,F,G,Hi
hE,F,G,Hi hE, F,G, F,Gi
hG,H,G,H,G,Hi
extracted using one or several of the proposed crite-
ria are shown. For k not equal to 0.01 s the num-
ber of patterns using the criteria of min coh is smaller
than those extracted using criteria of min con f or
min con f
B
, respectively. With combination of two
criteria, the best result (smaller number of patterns) is
obtained using min con f and min coh. However, the
combination of the three criteria delivers much better
results.
Table 2 shows the patterns extracted with the com-
bination of the three criteria (conf ∧ coh ∧ conf
B
)
for k=0.02 s to k=0.04 s. The two patterns hL,M,Ni
and hE, F,G,Hi embedded in the sequence were ex-
tracted satisfactorily (except for k=0.01 s). As the
constrain of maximal gap is relaxed (k increases),
other frequent patterns involving mainly the frequent
events F, G, and H begins to be significant.
This example shows that the proposed indexes
of cohesion (coh) and backward-confidence (conf
B
)
may be helpful in the selection of the most significant
patterns, improving the results obtained by the simple
extraction of maximal episodes or episode rules.
5 CONCLUSIONS
The problem of discovering significance of episodes
(patterns) has been analysed and two new indexes
called cohesion and backward-confidence of the
episodes have been proposed to improve pattern dis-
covery from frequent episodes. A new method to find
the maximal number of serial and parallel occurrences
has also been presented. Experimental results using a
synthetic sequence show that both, the indexes and
the algorithms proposed, are useful to search signifi-
cant patterns in sequences of events.
Set the properties of the method as well as as-
sessing their performance against similar frameworks
using real and synthetic data, is part of the work in
progress.
ACKNOWLEDGEMENTS
This work was supported by the research project
“Monitorizaci
´
on Inteligente de la Calidad de la En-
erg
´
ıa El
´
ectrica” (DPI2009-07891) funded by the
Ministerio de Ciencia e Innovaci
´
on (Spain) and
FEDER. Also with the support of the
Comis-
sionat per a Universitats i Recerca del Departament
d’Innovacio, Universitats i Empresa
of the Generali-
tat de Catalunya and also the European Social Fund
under the FI grant 2012FI B2 00119.
REFERENCES
Agrawal, R. and Srikant, R. (1994). Fast algorithms for
mining association rules. In Int. Conf. Very Large
Data Bases (VLDB’94).
Agrawal, R. and Srikant, R. (1995). Mining sequential
patterns. In Int. Conf. Data Engineering (ICDE’95),
pages 3–14.
Casas-Garriga, G. (2003). Discovering unbounded episodes
in sequential data. In Lavrac, N., Gamberger, D.,
Todorovski, L., and Blockeel, H., editors, Knowledge
Discovery in Databases: PKDD 2003, volume 2838
of Lecture Notes in Computer Science, pages 83–94.
Springer Berlin / Heidelberg.
Doucet, A. and Ahonen-Myka, H. (2006). Fast extraction
of discontiguous sequences in text: a new approach
based on maximal frequent sequences. Proceedings
of IS-LTC, 2006:186–191.
Gan, M. and Dai, H. (2010). A study on the accuracy of fre-
quency measures and its impact on knowledge discov-
ery in single sequences. In Data Mining Workshops
(ICDMW), 2010 IEEE International Conference on,
pages 859–866.
Gan, M. and Dai, H. (2011). Fast mining of non-derivable
episode rules in complex sequences. In Torra, V.,
Narakawa, Y., Yin, J., and Long, J., editors, Model-
ing Decision for Artificial Intelligence, volume 6820
of Lecture Notes in Computer Science, pages 67–78.
Springer Berlin / Heidelberg.
Iwanuma, K., Ishihara, R., Takano, Y., and Nabeshima, H.
(2005). Extracting frequent subsequences from a sin-
gle long data sequence a novel anti-monotonic mea-
sure and a simple on-line algorithm. In Data Mining,
Fifth IEEE International Conference on, page 8 pp.
Laxman, S., Sastry, P., and Unnikrishnan, K. (2007). Dis-
covering frequent generalized episodes when events
persist for different durations. Knowledge and Data
Engineering, IEEE Transactions on, 19(9):1188–
1201.
Laxman, S., Sastry, P. S., and Unnikrishnan, K. P. (2004).
Fast algorithms for frequent episode discovery in
event sequences. Technical report, CL-2004-04/MSR,
GM R&D Center, Warren.
Mannila, H., Toivonen, H., and Verkamo, A. I. (1997). Dis-
covery of frequent episodes in event sequences. Data
Mining and Knowledge Discovery, 1(3):259–289.
Patnaik, D. (2006). Application of frequent episode frame-
work in microelectrode array data analysis. Master’s
thesis, Dept. Electrical Engineering, Indian Institute
of Science, Bangalore.
Zhou, W., Liu, H., and Cheng, H. (2010). Mining closed
episodes from event sequences efficiently. In Proceed-
ings of the 14th Pacific-Asia Conference on Knowl-
edge Discovery and Data Mining(1), pages 310–318.
KDIR2012-InternationalConferenceonKnowledgeDiscoveryandInformationRetrieval
328