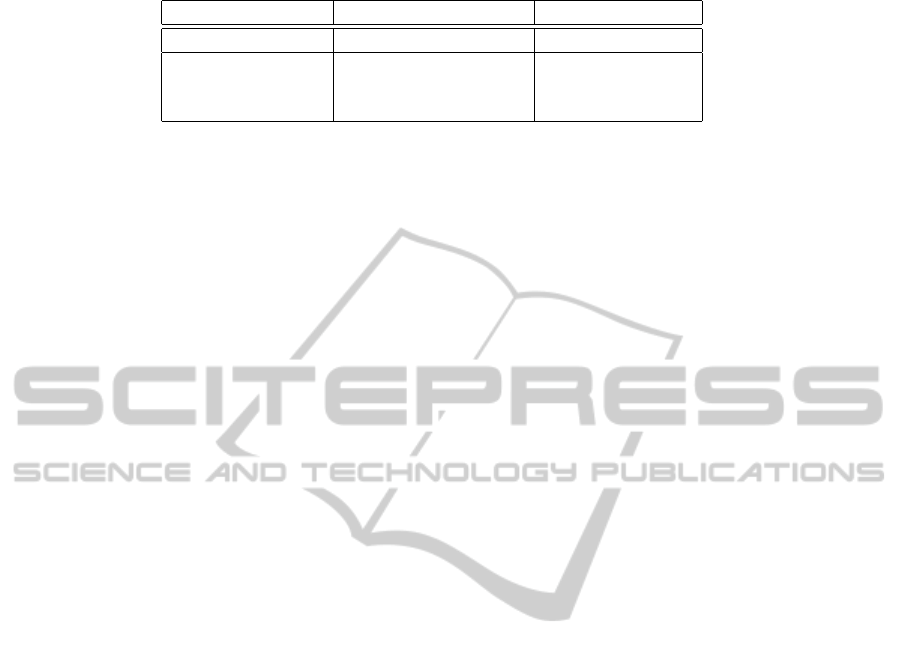
Table 3: Averaged rewards of the different approaches.
Approach Packet-Delivery-Ratio Inverse-Overhead
Default values 0.4006 0.1772
Selective 0.4509(+12.6%) 0.1923(+8.5%)
Multi-dimensional 0.4495(+12.2%) 0.1928(+8.8%)
Cumulative 0.4438(+10.8%) 0.1921(+8.4%)
term flexibility is used and defined what we want to
achieve in technical systems. Afterwards, we ex-
plained the need for novel techniques and mecha-
nisms to allow for a flexible system behaviour in case
of learning and organic systems. Therefore, the basic
Observer/Controller pattern and its technical imple-
mentation have been mentioned.
The evaluation part introduced three different ap-
proaches to achieve flexibility for the rule-based on-
line learning activities. According to the Organic
Network Control system as example application, we
compared the different concepts in a realistic envi-
ronment. Based on these first insights, we will con-
tinue to find solutions that keep the existing experi-
ence in case of changing goals and allow for an effi-
cient learning. The paper showed that flexibility is an
important issue and needs further research activities.
In upcoming OC systems, flexibility will gain in-
creasing attention. Therefore, future work will explic-
itly have to cope with related mechanisms and strate-
gies. One possibility to investigate the problem sepa-
rated from the limitations and noisy effects of realistic
applications is the previously mentioned animat sce-
nario (see section 2). This scenario will serve as one
example and basis for more abstract investigations of
the flexibility problem.
REFERENCES
Becker, C. (2011). Untersuchung von Mechanismen zur
technischen Umsetzbarkeit von Flexibilitaet in Organ-
ischen Systemen. Diploma thesis, Leibniz Universit
¨
at
Hannover.
Benjaafar, S. and Ramakrishnan, R. (1996). Modeling,
Measurement and Evaluation of Sequencing Flexibil-
ity in Manufacturing Systems. Int. J. of Production
Research, 34:1195 – 1220.
Compton, K. (2004). Flexibility Measurement of Domain-
Specific Reconfigurable. In ACM/SIGDA Symp. on
Field-Programmable Gate Arrays, pages 155 – 161.
Eden, A. H. and Mens, T. (2006). Measuring software flex-
ibility. IEE Proceedings - Software, 153(3):113–125.
Grefenstette, J. J. and Ramsey, C. L. (1992). An Approach
to Anytime Learning. In Proc. of the 9th Int. Workshop
on Machine Learning, pages 189–195.
Hassanzadeh, P. and Maier-Speredelozzi, V. (2007). Dy-
namic flexibility metrics for capability and capacity.
Int. J. of Flexible Manufacturing Systems, 19:195 –
216.
Kephart, J. O. and Chess, D. M. (2003). The Vision of
Autonomic Computing. IEEE Computer, 36(1):41–
50.
Kunz, T. (2003). Reliable Multicasting in MANETs. PhD
thesis, Carleton University.
Naujoks, B. and Beume, N. (2005). Multi-objective optimi-
sation using S-metric selection: Application to three-
dimensional solution spaces. In Proc. of CEC’05,
pages 1282 – 1289. IEEE.
Schmeck, H. (2005a). Organic Computing. K
¨
unstliche In-
telligenz, 3:68 – 69.
Schmeck, H. (2005b). Organic Computing – A new vision
for distributed embedded systems. In Proc. of the 8th
IEEE Int. Symp. on Object-Oriented Real-Time Dis-
tributed Computing, pages 201 – 203.
Schmeck, H., M
¨
uller-Schloer, C., C¸ akar, E., Mnif, M., and
Richter, U. (2010). Adaptivity and self-organization
in organic computing systems. ACM Transactions on
Autonomous and Adaptive Systems (TAAS), 5(3):1–32.
Shuiabi, E., Thomson, V., and Bhuiyan, N. (2005). Entropy
as a measure of operational flexibility. European Jour-
nal of Operational Research, 165(3):696 – 707.
Tennenhouse, D. (2000). Proactive computing. Communi-
cations of the ACM, 43(5):43–50.
Tomforde, S. (2012). Runtime adaptation of tech-
nical systems: An architectural framework for
self-configuration and self-improvement at runtime.
S
¨
udwestdeutscher Verlag f
¨
ur Hochschulschriften.
ISBN: 978-3838131337.
Tomforde, S., Hurling, B., and H
¨
ahner, J. (2011). Dis-
tributed Network Protocol Parameter Adaptation in
Mobile Ad-Hoc Networks. In Informatics in Control,
Automation and Robotics, volume 89 of LNEE, pages
91 – 104. Springer.
Williams, B. and Camp, T. (2002). Comparison of broad-
casting techniques for mobile ad hoc networks. In
Proc. of the 3rd ACM int. symp. on Mobile ad hoc net-
working & computing, pages 194–205. ACM.
Wilson, S. W. (1994). ZCS: A zeroth level classifier system.
Evolutionary Computation, 2(1):1–18.
Wilson, S. W. (1995). Classifier fitness based on accuracy.
Evolutionary Computation, 3(2):149–175.
ICINCO2012-9thInternationalConferenceonInformaticsinControl,AutomationandRobotics
292