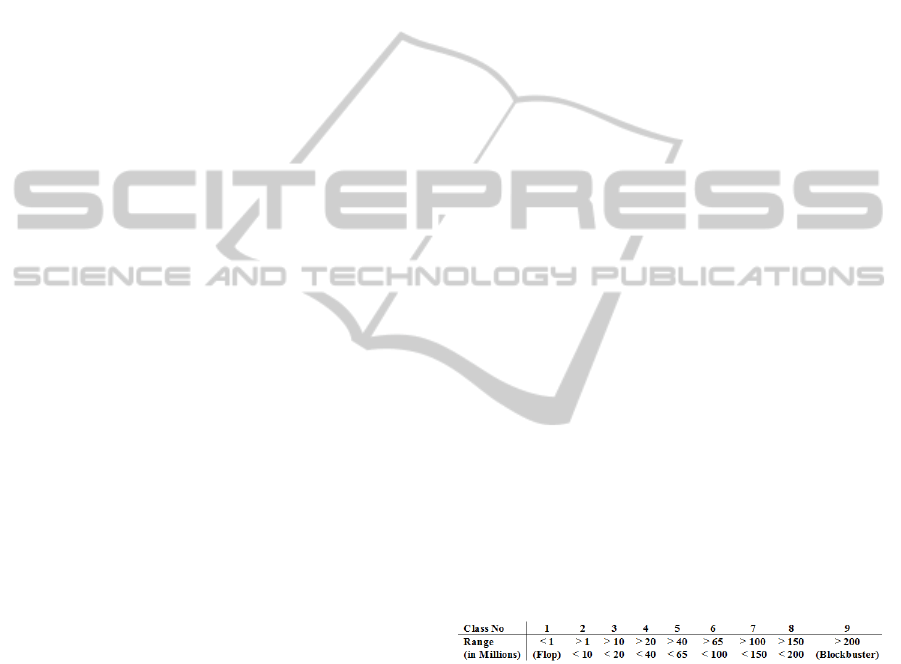
follows. The next section briefly reviews the
literature on forecasting the box office success of
theatrical movies. Section three provides the details
of our methodology by specifically talking about the
data, the model types, the experimental design used
in this study. Next, the prediction results are
presented and briefly explained. The last section of
the paper discusses the overall contribution of this
study along with its limitations and further research
directions.
2 LITERATURE REVIEW
Literature on forecasting financial success of new
motion pictures can be classified based on the type
of forecasting model employed: (i)
Econometric/Quantitative Models—those that
explore factors that influence the box office receipts
of newly released movies (Litman, 1983); (Litman
and Kohl, 1989); (Sochay, 1994); (Litman and Ahn,
1998); (Elberse and Eliashberg, 2002), and (ii)
Behavioral Models—those that primarily focuses on
the individual’s decision making process with
respect to selecting a specific movie from a vast
array of entertainment alternatives (Eliashberg and
Sawhney, 1994); (Sawhney and Eliashberg, 1996);
(Zufryden, 1996); (De Silva, 1998), (Eliashberg et
al., 2000). These behavioral models usually employ
a hierarchical framework where behavioral traits of
consumers are combined (mostly in a sequential
process) with the econometric factors in developing
the forecasting models. Another classification is
based on the timing of the forecast: (i) Before the
Initial Release—that is forecasting the financial
success of the movies before their initial theatrical
release (Litman, 1983); (Litman and Kohl, 1989);
(Sochay, 1994); (Zufryden, 1996); (De Silva, 1998);
(Eliashberg et al., 2000), (ii) After the Initial
Release—that is forecasting the financial success of
the movies after their initial theatrical release where
the first week of receipts are known (Sawhney and
Eliashberg, 1996); (Ravid, 1999). Forecasting
models that fall into the category of “after the initial
release” tend to generate more accurate forecasting
results due to the fact that those models have more
explanatory variables including box-office receipts
from the first week of viewership, movie critics, and
word-of-mouth effects. Our study falls into the
category of quantitative models for model type
classification, and into the category of before the
initial release in timing of the forecast classification.
Following is a chronological review of the most
relevant and the most cited literature published in
the field of forecasting financial success of theatrical
movies.
3 RESEARCH METHODOLOGY
In this section, we briefly explain (1) the nature of
data SET used for the experimentations, (2) the
machine learning methods selected and used, (3) the
experimentation methodology utilized, and (4) the
performance metrics used for prediction accuracy.
3.1 The Data
In our study, we used 386 movies released between
2009 and 2010. The sample data was drawn
(partially purchased) from IMBD.com, ShowBiz
Data Inc., among others. The dependent variable in
our study is the box-office gross revenues, not
including auxiliary revenues such as video rentals,
international market revenues, toy and soundtrack
sales, etc. Another important difference between our
study and previous efforts is that we convert the
forecasting problem into a classification problem.
Rather than forecasting the exact amount of the
dependent variable (box-office receipts), we classify
a movie based on its box-office receipts in one of
nine categories, ranging from a “flop” to a
“blockbuster.” This process of converting a
continuous variable in a limited number of classes is
commonly called in literature as “discretization” or
“binning.” In this study, we discretized the
dependent variable into nine classes using the
following breakpoints. These breakpoints are
determined largely based on our consultations with
several decision makers in the movie business.
We used a large number of independent variables.
Our choice of independent variables is based
partially on the previous studies conducted in the
field. Each independent categorical variable is
converted into an appropriate representation, which
created a number of pseudo variables increasing the
independent variable count.
3.2 The Machine Learning Methods
Used
In this study, three most popular classification
methods are used (and compared to each other):
decision trees, artificial neural networks and logistic
regression. These prediction methods are selected
ICINCO2012-9thInternationalConferenceonInformaticsinControl,AutomationandRobotics
654