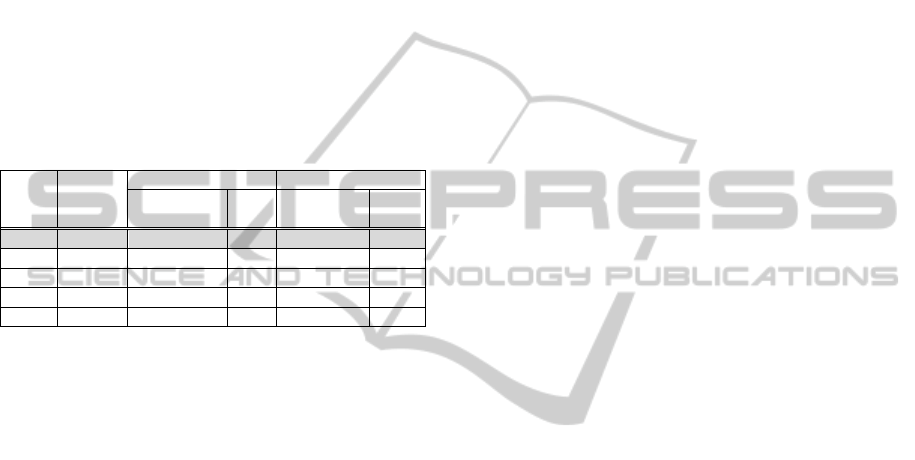
of each population. Then, our system monitored 256
normal executable files and 122 virus executable
files transmitted for testing in test-bed network. As a
result, all executable files have been extracted
perfectly. Finally, the byte distribution values of the
extracted executable files were compared with the
clustering central values of each population. Here,
the truncation size of each region is determined as
the size which most well can distinguish between
normal executable file and malicious executable file
through learning tests of several times. Basically, the
size of each region is more than the minimum 100
bytes for the data confidence. Most of all, we made a
greater effort for minimizing the false positive rate,
and maximizing the detection rate.
Table 1: Experimental results.
+ A.(All), D.(DOS header), P.(PE header), S.T.(Section Table),
S.(Sections), C.(Count)
Our experimental result in this way is shown in
the table 1. In the case of the extracted normal
executable files, 230 executable files were altogether
normally determined in each region. In the case of
the other side, only 8 executable files were
altogether normally determined in each region. That
is, the normal executable files were normally judged
with about 90% among 256 normal executable files
for testing. On the other hand, the malicious
executable files were as detected as 93% degree
among 122 virus executable files for testing.
5 CONCLUSIONS
In this paper, we present the network-based
executable file extraction and analysis technique for
malware detection. The proposed technique can
detect not only the known malicious software but
also unknown malicious software. Most of all, our
approach easily can detect the malicious software
without the complicated command analysis.
Therefore, it can minimize the load on the system
execution. Besides, it can perform the real-time
malware detection as a network inline-mode by
using in reconfiguring hardware. Finally, we
reported the experimental results of our approach.
As shown in the experimental result, our approach
showed a false positive rate under 10% and a
detection rate over 90% beyond expectation. In
future, we need to focus on reducing its false rate as
the further study through more experimental results.
Also, we will keep up our efforts for improvement in
performance of detection mechanism on real world
environment.
REFERENCES
Liu Wu, Ren Ping, Liu Ke, and Duan Hai-xin, 2011,
‘Behavior-based Malware Analysis and Detection’, In
Proceedings of the 2011 First International Workshop
on Complexity and Data Mining, Nanjing, China, pp.
39–42.
Brijesh Kumar and Constantine Katsinis, 2010, ‘A
Network Based Approach to Malware Detection in
Large IT Infrastructures’, In Proceedings of the 2010
Ninth IEEE International Symposium on Network
Computing and Applications, MA, USA, pp. 188–191.
Ikkyun Kim, Daewon Kim, Byoungkoo Kim, Yangseo
Choi, Seoungyong Yoon, Jintae Oh, and Jongsoo Jang,
2009. ‘A case study of unknown attack detection
against zero-day worm in the honeynet environment’,
In Proceedings of the 11th international conference on
Advanced Communication Technology, NJ, USA, pp.
1715–1720.
Wei-Jen Li, Ke Wang, Salvatore J. Stolfo, and Benjamin
Herzog, 2005. ‘Fileprints: Identifying File Types by n-
gram Analysis’, In Proceedings of the 2005 IEEE
Workshop on Information Assurance and Security,
West Point, NY, USA, pp. 64–71.
Ke Wang, Gabriela Cretu, and Salvatore J. Stolfo, 2005.
‘Anomalous Payload-based Worm Detection and
Signature Generation’, In Symposium on Recent
Advances in Intrusion Detection, Seattle, WA, USA,
pp. 227–246.
Stig Andersson, Andrew Clark, and George Mohay, 2004.
‘Network-Based Buffer Overflow Detection by
Exploit Code Analysis’, In Proceedings of the
AusCERT Asia Pacific Information Technology
Security Conference, Gold Coast, Australia, pp. 23–27.
C. Kr¨ugel, T. Toth, and E. Kirda, 2002. ‘Service Specific
Anomaly Detection for Network Intrusion Detection’,
In Proceedings of the 2002 ACM symposium on
Applied computing, NY, USA, pp. 201–208.
W. Lee and D. Xiang, 2001. ‘Information-theoretic
measures for anomaly detection’, In Proceedings of
the 2001 IEEE Symposium on Security and Privacy,
Washington, DC, USA, pp. 130–143.
Network-based Executable File Extraction and Analysis for Malware Detection
433