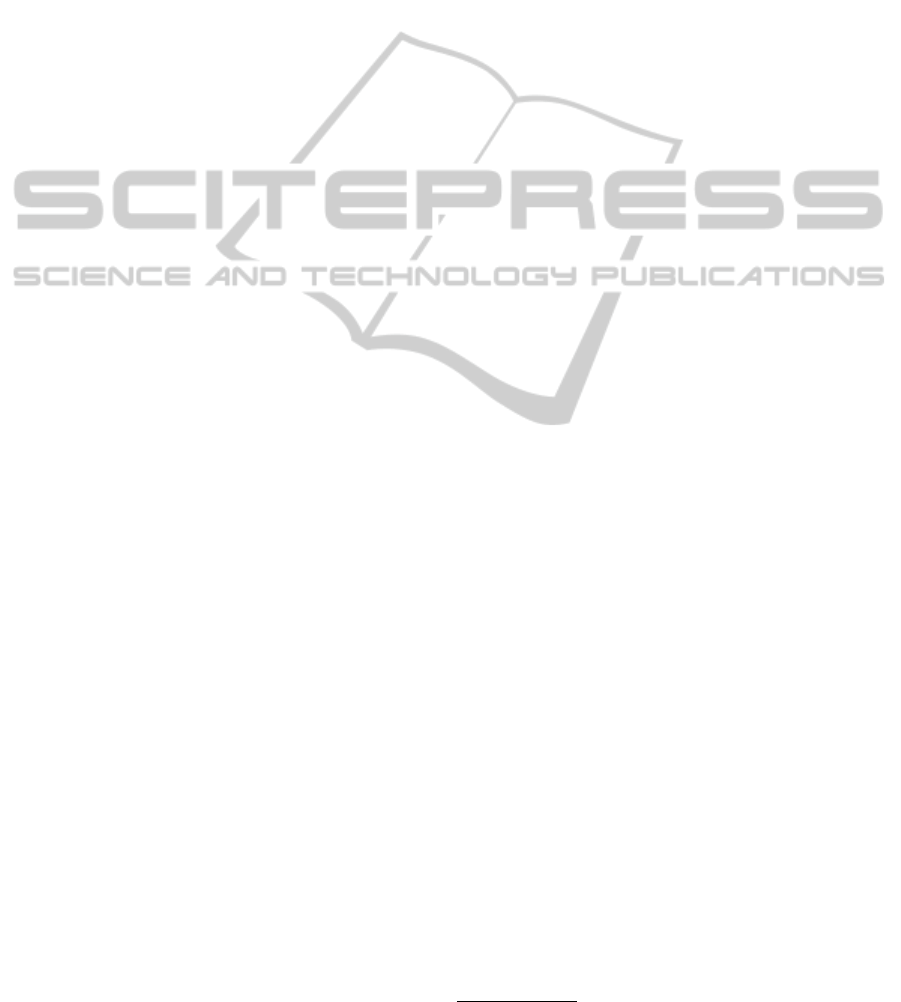
And finally, regarding with the evaluation, Li et
al. (Li and Motta, 2010) analysed evaluation meth-
ods in semantic representations. They used other
areas and methods to classify three types of eval-
uations: application-driven ontology, gold standard
based ontology, and corpus coverage ontology. More-
over, they provided a list of evaluation measures:
recall-based, sentence-rank-based, and content-based.
They applied these observations in two previous ap-
proaches (Peroni et al., 2008; Zhang et al., 2007) us-
ing financial and biosphere ontologies.
In order to introduce our approach, we have consid-
ered noteworthy the relativity of this subject: the sum-
marization depends entirely on the person who do it
or on the final nature that it has the application. Thus,
we decided to carry on a brief questionnaire where the
volunteers did not know nothing about ontologies and
the aim of the same, but most of them were university
students and the rest was research staff.
We realize some expected results. The most sim-
ple is a structure, the most easier is the selection of
elements. A taxonomy is more simple visually than a
graph and a simple text, and the centrality of the el-
ements by means of other concepts determine its sig-
nificance. Thus, the organization/layout is the key of
the selection. When all the elements are isolated in a
layout people choose those words that are more sim-
ple and more frequents independently of the context.
Furthermore, people answered that the context is vital
for select elements, but the position inside the repre-
sentation is not crucial.
Roughly speaking, human criterion for determin-
ing the importance of elements in complex structure
representations such as ontologies is not objective.
In addition, in this type of graphs, the cardinality
and complex OWL axioms are not included. Perhaps
other type of representations could be more useful but
it is not our study. In addition, as the notion of ‘impor-
tance’ is relative, the importance of a elements may be
not set by the position. It is more useful to take into
account the frequency or the sparseness ratio. Finally,
the evaluation of ontology summarization approaches
should avoid the human judgements and it should be
based on quantitative measures from computational
issues such as: performance indices, data-driven ap-
plications and reasoning tasks.
3 OUR APPROACH
Our design try to achieve some basic notions. (i) The
representation and its interpretation sets the size of
the summarization, the final size depends on the orig-
inal source. For that, text summarization is variable
according with human criteria. As we have checked,
each person decides that concepts are more useful. In
this way, our design extracts a number undetermined
of classes and their respective properties in function
of the whole representation. With this design, the
evaluation is transparent to the decision of setting a
size. As we see experimentally, this indetermination
remains constant under a certain percentage of ele-
ments. (ii) The ratio between utility and summariza-
tion computational cost should be low. It seems more
useful to use all ontology elements that the summa-
rization when the second process has a bigger com-
putational cost. This fact impacts in the design of
the pruning measures. (iii) According with (Peroni
et al., 2008), the pruning measures should mark the
elements that are information-rich, that is known by
the notion of density. (iv) The previous fact implies
that those concepts have a great number of triples and
for example, it could be useful in caching techniques.
Thus, ontology elements are clustered by their num-
ber of triplets, which it means that they are more men-
tioned.
In contrast with (Peroni et al., 2008), our prun-
ing measures does not consider the label of a con-
cept since the interpretation of labels only is used in
human evaluations. Moreover, the number of com-
pound nouns is around 56% considering only con-
cepts. We analysed 693 labels concepts form 13 on-
tologies which are part of Ontology Alignment Eval-
uation Initiative (OAEI)
1
in the dataset of conference
2010. In our opinion, this group of ontologies can
be considered as ‘real ontologies’ since they present
a wide set of OWL axioms and they are not simple
taxonomies.
Peroni and Zhang mentioned the notion of den-
sity/coverage and centrality/re-ranking weight the
classes. Basically, in the first case, the number of
subclasses, properties and instances are weighted and
divided by the ratio of distance. The final score of
a classes depends on three weighted components: it
measures this density, another one the popularity hit-
based on Yahoo queries, and the last one component
considers the label string. In the second approach,
the notion of centrality is based on a ratio of input-
output degree of vertexes, plus an algorithm to cal-
culate the shortest-path and another based on eigen-
vector. They apply these algorithms on RDF sen-
tences. In conclusion, OWL axioms are not consid-
ered for selecting classes, only the ‘centrality’ and
‘citation’ of classes is the common criteria. At the
same time, other factors as queries are used to select
classes. In the fig. 1, it is represented biosphere ontol-
ogy where some classes have some extra-circles ac-
1
http://oaei.ontologymatching.org/
KEOD2012-InternationalConferenceonKnowledgeEngineeringandOntologyDevelopment
340