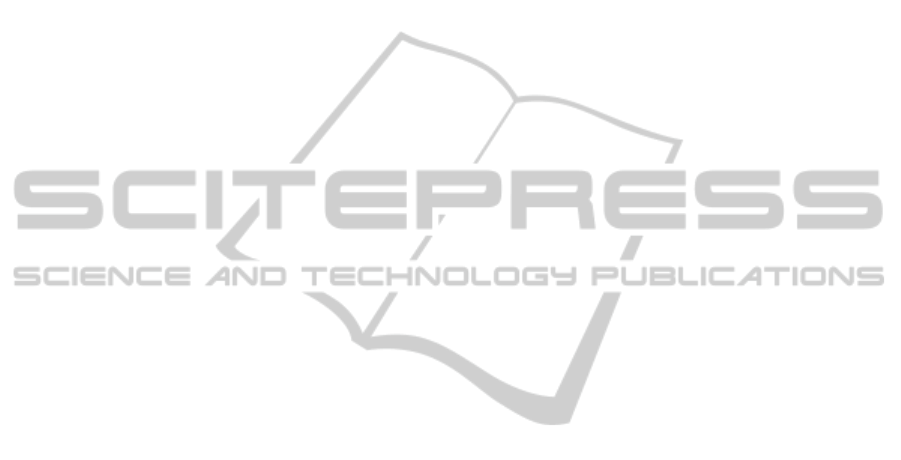
5 CONCLUSIONS
As the images and videos databases become huge, it is
essential to find efficient, fast, but also accurate tech-
niques to annotate and enrich multimedia contents in
an automatic way. Both automatic processing and
manual annotation methods have advantages, and we
propose to merge them in a hybrid system that links
user-generated annotations with visual content anal-
ysis techniques. Resulting enriched multimedia con-
tent will allow us to improve current television ex-
perience (interaction, personalization, sharing, etc.),
in order to meet expectations of the users of the next
generation television systems. As a starting point in
this vision, we would like to build a dynamic recom-
mender system, that exploits both video content anno-
tations and a user profile builder based on behavioral
analysis.
REFERENCES
Akrivas, G., Papadopoulos, G., Douze, M., Heinecke,
J., O’Connor, N., Saathoff, C., and Waddington, S.
(2007). Knowledge-based semantic annotation and
retrieval of multimedia content. In Proc. of 2nd Inter-
national Conference on Semantic and Digital Media
Technologies, pages 5–6, Genoa, Italy.
Andreetto, M., Zelnik-Manor, L., and Perona, P. (2008).
Unsupervised learning of categorical segments in im-
age collections. Proc. of Computer Vision and Pattern
Recognition Workshops, 2008. CVPRW ’08., pages 1–
8.
Ballan, L., Bertini, M., Bimbo, A. D., Meoni, M., and Serra,
G. (2010). Tag suggestion and localization in user-
generated videos based on social knowledge. In Proc.
of second ACM SIGMM Workshop on Social Media
(WSM), pages 3–8.
Bertini, M., Amico, G. D., Ferracani, A., Meoni, M., and
Serra, G. (2010). Web-based Semantic Browsing of
Video Collections using Multimedia Ontologies. In
Proceedings of the international conference on Mul-
timedia - MM’10, pages 1629–1632, Firenze, Italy.
ACM.
Bizer, C. (2009). The Emerging Web of Linked Data. IEEE
Intelligent Systems, 24(5):87–92.
Blei, D. M., Ng, A. Y., and Jordan, M. I. (2003). Latent
Dirichlet Allocation. Journal of Machine Learning
Research, 3:993–1022.
Bosch, A. and Zisserman, A. (2006). Scene classification
via pLSA. Proc. of ECCV.
Cai, D., Mei, Q., Han, J., and Zhai, C. (2008). Modeling
hidden topics on document manifold. In Proc. of the
17th ACM conference on Information and knowledge
management, pages 911–920.
Cooper, W. (2008). The interactive television user experi-
ence so far. Proc. of the 1st international conference
on Designing interactive user experiences for TV and
video (UXTV), 44:133.
Damme, C. V., Hepp, M., and Siorpaes, K. (2007). Folk-
sOntology: An Integrated Approach for Turning Folk-
sonomies into Ontologies. Social Networks, 2:57–70.
Dong, A. and Li, H. (2006). Multi-ontology Based Mul-
timedia Annotation for Domain-specific Information
Retrieval. IEEE International Conference on Sensor
Networks, Ubiquitous, and Trustworthy Computing -
Vol 2 - Workshops, 2:158–165.
Guillaumin, M., Mensink, T., Verbeek, J., and Schmid, C.
(2009). Tagprop: Discriminative metric learning in
nearest neighbor models for image auto-annotation. In
Computer Vision, 2009 IEEE 12th International Con-
ference on, pages 309 –316.
Hauptmann, A. G., Christel, M. G., and Yan, R. (2008).
Video retrieval based on semantic concepts. In Pro-
ceedings of the IEEE, volume 96, pages 602–622.
Hofmann, T. (2001). Unsupervised Learning by Probabilis-
tic Latent Semantic Analysis. Mach. Learn., pages
177–196.
H¨orster, E., Lienhart, R., and Slaney, M. (2007). Image re-
trieval on large-scale image databases. Proc. of Con-
ference on Image and video retrieval, pages 17–24.
Hu, D. (2009). Latent Dirichlet Allocation for Text, Images,
and Music. cseweb.ucsd.edu, pages 1–19.
Kennedy, L. S., Chang, S. F., and Kozintsev, I. V. (2006).
To search or to label?: predicting the performance of
search-based automatic image classifiers. Proc. of the
8th ACM international workshop on Multimedia In-
formation Retrieval (MIR), pages 249–258.
Lew, M. S., Sebe, N., Djereba, C., and Jain, R. (2006).
Content-Based Multimedia Information Retrieval :
State of the Art and Challenges. ACM Transactions
on Multimedia Computing, Communications, and Ap-
plications (TOMCCAP), 2(1):1–19.
Li, X. and Snoek, C. (2009). Visual categorization with neg-
ative examples for free. In Proc.s of ACM Multimedia,
pages 661–664.
Li, X., Snoek, C. G. M., and Worring, M. (2009). Learn-
ing Social Tag Relevance by Neighbor Voting. IEEE
Transactions on Multimedia, 11:1310–1322.
Lienhart, R. and Slaney, M. (2007). pLSA on large scale
image databases. Proc. of IEEE International Con-
ference on Acoustics, Speech and Signal Processing,
(ICASSP), pages 1217–1220.
Makadia, A., Pavlovic, V., and Kumar, S. (2008). A new
baseline for image annotation. In Proc. ECCV, pages
316–329.
Meyer, D. (2001). Support Vector Machines. R News,
2(2):23–26.
Monay, F. and Gatica-Perez, D. (2004). PLSA-based im-
age auto-annotation: constraining the latent space. In
Proc. of ACM Multimedia, pages 348–351.
Nguyen, C., Phan, X., and Horiguchi, S. (2009). Web
Search Clustering and Labeling with Hidden Topics.
ACM Transactions on Asian Language Information
Processing (TALIP), 8(3).
Phan, X., Nguyen, L., and Horiguchi, S. (2008). Learning
to classify short and sparse text & web with hidden
SIGMAP2012-InternationalConferenceonSignalProcessingandMultimediaApplications
196