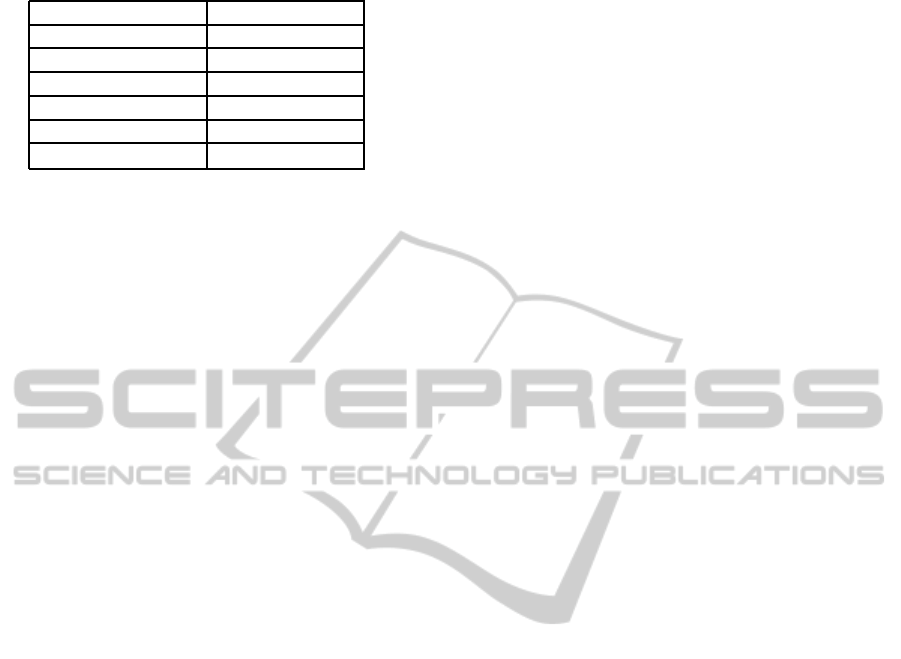
Table 1: Corresponding pairs of phonemes.
Unvoiced phoneme Voiced phoneme
f v
k g
s z
ˇ
s
ˇz
t d
ˇ
t
ˇ
d
4 EXPERIMENTS
Two different approaches were tested and compared
together (for both training corpuses). In the first one
the basic speech unit was monophone in contrast to
triphone in the second one. In all our experiments
the individual basic speech unit was represented by
a three-state HMM with a continuous output prob-
ability density function assigned to each state. As
the number of the Czech triphones is large, phonetic
decision trees were used to tie the states of the tri-
phones. Several experiments were performed to de-
termine the best recognition results according to the
number of clustered states and also to the number of
mixtures. The prime Gaussians triphone/monophone
acoustic model trained with the Maximum Likelihood
(ML) criterion was made with HTK-Toolkit v.3.4.
The special systems using phonemes mapping
were built for testing of speech recognition. The main
idea of the experiment is based on the vocalization of
all produced phonemes. In this case no difference be-
tween results given by system without mapping and
phonemes mapping system should be detected. In
specific case the accuracy of recognition could even
be improved due to reduction of the system perplex-
ity. The system does not use the full phonetic set.
Conversely, in case of nonlarygectomees the re-
duction of the phonetic set could lead to reducing the
accuracy. Remember that the source data were cho-
sen with an emphasis of inclusion of all Czech tri-
phone/monophone in corresponding representation.
The test set consists of 500 sentences for both
training corpuses (nonlarygectomees and laryngec-
tomee speech). This portion of sentences (10% of
the original training set) contains approximately 1
hour of speech for each speaker. In all recognition
experiments, a language model based on zerogram
as well as a trigram-based one were applied in or-
der to judge a quality of developed acoustic models.
The perplexity of the zerogram language model was
2885 (in other words, the recognition lexicon con-
tained 2885 words) and there were no OOV words.
The trigram languages models were trained by SRI
Language Modeling Toolkit (Stolcke, 2002) using
modified Kneser-Ney smoothing that proved to be
efficient in our previous language modeling experi-
ments (Praˇz´ak et al., 2008). We have collected large
corpus containing the data from newspapers (520 mil-
lion tokens), web news (350 million tokens), subtitles
(200 million tokens) and transcriptions of some TV
programs (175 million tokens). The model contained
the most frequent 360K words with OOV amounting
to 3.8%. The perplexity of the recognition task was
3380.
The verification of the assumption was realized by
an acoustic models using triphone/monophone for the
speech recognition. All models are created for both
speakers. Firstly the baseline acoustic model with-
out mapping was created. Then the model that maps
only voiceless triphones/monophones. Due to identi-
fication of the influence of each phoneme on system
accuracy four more models were built.
• acoustic model with mapping ’f’ on ’v’;
• acoustic model with mapping ’k’ on g;
• acoustic model with mapping ’s’,’ ˇs’ on ’z’, ’ˇz’;
• acoustic model with mapping ’t’, ’
ˇ
t’ on ’d’, ’
ˇ
d’;
For verification of our assumptions 24 acoustic
models were created (6 monophone model and 6 tri-
phone models for each speaker). Obtained recog-
nition accuracy is given in Table 2 for monophone
model with zerogrambased language model in case of
and Table 3 for monophone model with trigram lan-
guage model with 360K words lexicon.
From these tables it could be seen that every
change of phonetic set causes reducing of speech
recognition accuracy for nonlaryngectomee. How-
ever, for total laryngectomees it is not possible to con-
firm this assumption clearly. From computed results
it is possible to obtain information about accuracy,
thus about decreasing of accuracy due to replacing
unvoiced monophones/triphones by voiced one. The
same character of result was obtained from phoneme
mapping ’t’, ’
ˇ
t’ → ’d’, ’
ˇ
d’ and ’f’ → ’v’. Conversely,
if ’k’ was replaced by ’g’ then the higher speech
recognition accuracy was obtained than for baseline
model. From replacing ’s’,’ˇs’ → ’z’,’ˇz’ the obtained
results were not clear. Therefore the further work will
be focused on solution of this problem.
5 CONCLUSIONS
We have presented our initial investigations into the
challenging problem of transcribing electrolaryngeal
substitute speech of total laryngectomees. We have
SIGMAP2012-InternationalConferenceonSignalProcessingandMultimediaApplications
206