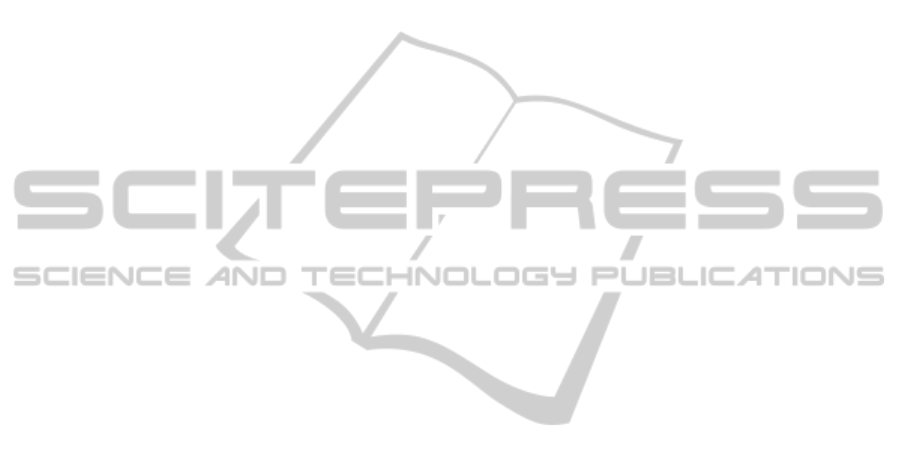
continuous evaluation of the efforts performed by
each student and a clear measure of their efficiency
in attaining the targeted starting values. More
specifically, these parameters can be appropriately
used to derive specific suggestion as rectify each
student’s path when calibration reveals necessary.
Finally, it is important to note that EVA analysis,
tools and charts would be a significant addition to
the educational roadmap developed in this paper.
5 CONCLUSIONS
In this paper we have presented an innovative
approach in using and applying Educational Data
Mining. An educational roadmap that reliably and
greatly endows both advisors and students a
straightforward manual for success. This roadmap is
built using Regression Trees with different types
elaborated in the frame of a continuous and close
follow-up of students’ performance. Correlation
Analysis and study of different splitting values of
the regression tress were extensively examined to
choose the best readable and useful tree/roadmap
while keeping a high rate of precision in the
estimation process. In this context, two types of trees
were developed and elaborately analyzed. The first
type identifies, on a yearly-basis, courses and their
respective grades which have greater impact on
students’ cumulative GPA upon graduation. The
second type is an iterative tree that re-injects the
current students’ GPA into the dataset as an
additional attribute. This proved to be a powerful
tool for decision making and endorsed the results
and the computed precision. The trees were trained,
cross-validated and tested. Calculation of the
precision and Correlation Analysis were achieved
based on untrained data to significantly enhance the
reliability of the outcome. Also a detailed
perspective of this study was presented under Earned
Value Analysis as a future scope of work and an
added tool for advising and monitoring students’
efforts, performance and evolution throughout their
engineering curricula.
REFERENCES
Kovačić, Z., Green, J., 2010, Research in Higher
Education Journal, Predictive working tool for early
identification of ‘at risk’ students, Open Polytechnic,
Wellington, New Zealand.
Sembiring, S., Zarlis, M., Hartama, D., Ramliana, S.,
Wani, E., 2011, International Conference on
Management and Artificial Intelligence, Prediction of
Student Academic Performance by an Application of
Data Mining Techniques, IACSIT Press vol. 6.
Wook, M., Yahaya, Y., Wahab, N., Isa, M., Awang, N. F.,
Seong, H. Y., 2009, In Proceedings of the Second
International Conference on Computer and Electrical
Engineering, Predicting NDUM Student’s Academic
Performance Using Data Mining Techniques, IEEE
computer society.
Al-Radaideh, Q., Al-Shawakfa, E., Al-Najjar, M. I., 2006,
The 2006 International Arab Conference on
Information Technology, ACIT'2006, Mining Student
Data Using Decision Trees.
Kovačić, Z., 2010, Proceedings of Informing Science & IT
Education Conference (InSITE). Early Prediction of
Student Success: Mining Students Enrolment Data
Portnoi, L. M., & Kwong, T. M., 2011, Journal of Student
Affairs Research and Practice, volume 48, No. 4.,
Enhancing the academic experiences of first-
generation master’s students.
Oladokun, V. O., Adebanjo, A. T., Charles-Owaba, O. E.,
2008, The Pacific Journal of Science and Technology,
Volume 9. Number 1., Predicting Students’ Academic
Performance using Artificial Neural Network: A Case
Study of an Engineering Course
Chadha, A., Kumar, V., 2011, International Journal of
Advanced Computer Science and Applications, Vol. 2,
No. 3., An Empirical Study of the Applications of Data
Mining Techniques in Higher Education
Pal, S., Baradwaj, B. K., 2011, International Journal of
Advanced Computer Science and Applications, Vol. 2,
No. 6., Mining Educational Data to Analyze Students’
Performance
Eshghi, A., Haughton, D., Li, M., Senne, L., Skaletsky M.,
Woolford, S., 2011, Journal of Institutional Research,
vol. 16, No.2., Enrolment Management in Graduate
Business Programs: Predicting Student Retention
Dowdy, S. Wearden, S., 1983, Statistics for Research,
Wiley. ISBN 0471086029 pp 230.
Domingos, P., 2007, Proceedings of Data Min. Knowledge
and Discovery, 21-28, Volume 15, Issue 1., Toward
Knowledge-Rich Data Mining
Fayyad, U. M.; Piaetsky-Shapiro, G.; Smyth, P., 1996,
Advances In Knowledge Discovery and Data Mining,
From Data Mining to Knowledge Discovery: An
Overview. AAAI/MIT press, Cambridge mass.
Witten, I. H., Frank, E., Hall, M. A., 2011, Data Mining:
Practical Machine Learning Tools and Techniques.
Morgan Kaufmann, Third Edition.
J., Han, M., Kamber, J., Pei, 2011, Data Mining: Concepts
and Techniques, Morgan Kaufmann, Third Edition.
Nock, R., Nielsen, F., 2006, IEEE Trans. on Pattern
Analysis and Machine Intelligence, Vol. 28, No. 8, On
Weighting Clustering, August 2006, p. 1–13.
Agrawal; R., Imielinski; T., Swami, A., 1993, Proceedings
of the ACM SIGMOD International Conference on
Management of Data, Mining Association Rules
between Sets of Items in Large Databases
Piatetsky-Shapiro, G., 1991, Knowledge Discovery in
Databases, Discovery, analysis, and presentation of
CreatinganEducationalRoadmapforEngineeringStudentsviaanOptimalandIterativeYearlyRegressionTreeusingData
Mining
51