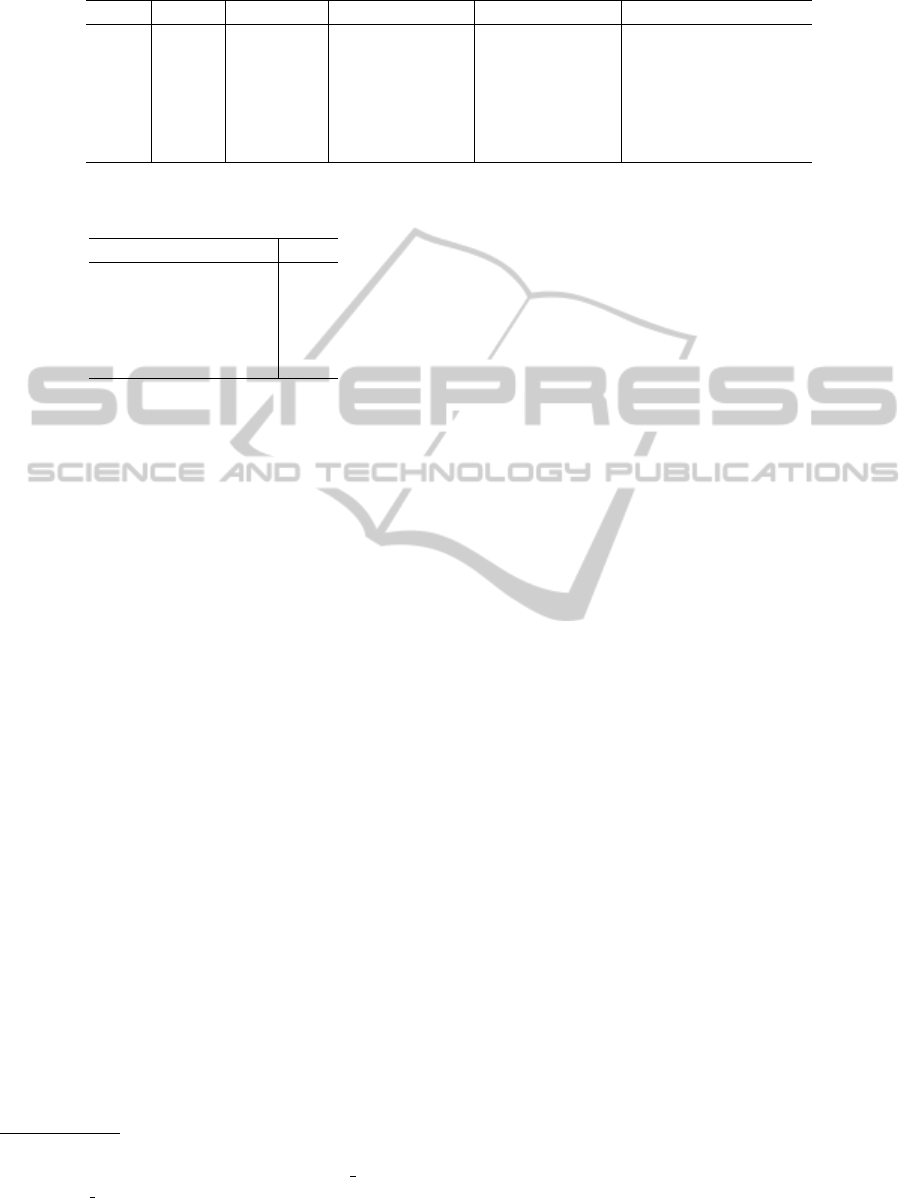
Table 3: Hotel recommendation list for guest ID 7630.
Rank Tr Rev (pos) Rev (pos&neg) Tr & Rev (pos) Tr & Rev (pos&neg)
1 28506 1529 80549 1529 15056
2 943 15683 8298 25110 1633
3 4929 25110 8298 15056 70194
4 54491 8298 1989 1633 931
5 1529 1633 15683 15683 11019
6 52322 80549 15056 80549 5146
Table 4: Similarities between the ranking hotel and correct
hotel.
Method sim
Tr 2.65
Rev (pos) 2.17
Rev (pos&neg) 2.30
Tr & Rev (pos) 2.04
Tr & Rev (pos&neg) 1.87
such as word-based sentiment analysis and Basket-
Sensitive Random Walk (Li et al., 2009), and (iii) ap-
plying the method to other data such as grocery stores:
LeShop
3
, TaFeng
4
and movie data: MovieLens
5
to
evaluate the robustness of the method.
REFERENCES
Balabanovic, M. and Shoham, Y. (1997). Fab Content-
based Collaborative Recommendation. In Communi-
cations of the ACM, 40:66–72.
Breese, J. S., Heckerman, D., and Kadie, C. (1998). Em-
pirical Analysis of Predictive Algorithms for Collab-
orative Filtering. In Proc. of the 14th Conference on
Uncertainty in Artificial Intelligence, pages 42–52.
Bremaud, P. (1999). Markov Chains: Gibbs Fields, Monte
Carlo Simulation, and Queues. Springer-Verlag.
Brin, S. and Page, L. (1998). The Anatomy of a Large-
scale Hypertextual Web Search Engine. Computer
Networks, 30(1-7):107–117.
Cane, W. L., Stephen, C. C., and Fu-lai, C. (2006). Integrat-
ing Collaborative Filtering and Sentiment Analysis. In
Proc. of the ECAI 2006 Workshop on Recommender
Systems, pages 62–66.
Deshpande, M. and Karypis, G. (2004). Item-based Top-N
Recommendation Algorithms. ACM Transactions on
Information Systems, 22(1):143–177.
Huang, Z., Chen, H., and Zeng, D. (2004). Applying Asso-
ciative Retrieval Techniques to Alleviate the Sparsity
Problem in Collaborative Filtering. ACM Trans. on
Information Systems, 22(1):116–142.
Joachims, T. (1998). SVM Light Support Vector Machine.
In Dept. of Computer Science Cornell University.
3
www.beshop.ch
4
aiia.iis.sinica.edu.tw/index.php?option=com docman&
task=cat view&gid=34&Itemid=41
5
http://www.grouplens.org/node/73
Li, M., Dias, B., and Jarman, I. (2009). Grocery Shopping
Recommendations based on Basket-Sensitive Ran-
dom Walk. In Proc. of the 15th ACM SIGKDD, pages
1215–1223.
Liu, N. N. and Yang, Q. (2008). A Ranking-Oriented Ap-
proach to Collaborative Filtering. In Proc. of the 31st
ACM SIGIR, pages 83–90.
Matsumoto, Y., Kitauchi, A., Yamashita, T., Hirano, Y.,
Matsuda, Y., Takaoka, K., and Asahara, M. (2000).
Japanese Morphological Analysis System Chasen Ver-
sion 2.2.1. In Naist Technical Report.
Niklas, J., Stefan, H. W., c. M. Mark, and Iryna, G. (2009).
Beyond the Stars: Exploiting Free-Text User Reviews
to Improve the Accuracy of Movie Recommendations.
In Proc. of the 1st International CIKM workshop on
Topic-Sentiment Analysis for Mass Opinion, pages
57–64.
Park, S. T., Pennock, D. M., Madani, O., Good, N., and
DeCoste, D. (2006). Naive Filterbots for Robust Cold-
Start Recommendations. In Proc. of the 12th ACM
SIGKDD, pages 699–705.
Turney, P. D. (2002). Thumbs Up or Thumbs Down? Se-
mantic Orientation Applied to Un-supervised Classi-
fication of Reviews. In Proc. of the 40th ACL, pages
417–424.
Xue, G. R., Yang, Q., Zeng, H. J., Yu, Y., and Chen, Z.
(2005). Exploiting the Hierarchical Structure for Link
Analysis. In Proc. of the 28th ACM SIGIR, pages 186–
193.
Yates, B. and Neto, R. (1999). Modern Information Re-
trieval. Addison Wesley.
Yildirim, H. and Krishnamoorthy, M. S. (2008). A Random
Walk Method for Alleviating the Sparsity Problem in
Collaborative Filtering. In Proc. of the 3rd ACM Con-
ference on Recommender Systems, pages 131–138.
KDIR2012-InternationalConferenceonKnowledgeDiscoveryandInformationRetrieval
198