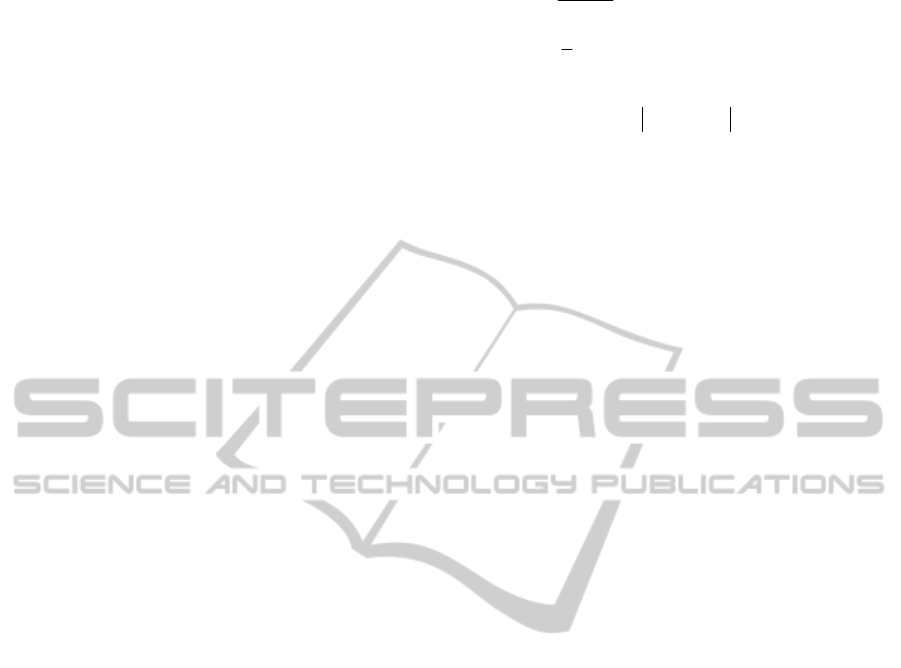
The concept list:
C = {c
i
| i=1, 2, … , m}
where c
i
denotes a concept (noun phrase)
captured from a CAE task, i=1, 2, … , m
defines the sequence of concepts
The potential trouble pool:
P ={(v , c)| c∈C, v is a verb }
The number of concepts in C is confined to m —
when a new concept is added, the oldest concept in
C is deleted and all subscripts are adjusted. For the
construction of P, other than manually building a
static lexicon, we choose to extract verb-object pairs
from the ever-evolving Internet corpus. To do this,
for noun phrase c
i
in C, we construct four quoted
queries:
“how to * <c
i
’s singular form> ”
“how to * <c
i
’s plural form> ”
“cannot * <c
i
’s singular form> ”
“cannot * <c
i
’s plural form> ”
Every query is to be submitted to a search engine
that supports wildcard and exact string match. After
the search engine has returned results for the
constructed queries, we use a part-of-speech tagger
to assign POS tags to matched texts, and any
contained verb-object structures using c
i
as argument
are extracted according to the following rule (in
BNF):
(“how to”|“cannot”) [adverb]<verb>[pronoun]
[adjective]<noun phrase c
i
>
We adopt above query patterns for three reasons:
first, we are mainly concerned with know-how
experiences; second, though there exists other
syntaxes such as “how can/do I/you do a thing” for
people to query know-how knowledge, a few search
trials can tell that with the same semantic meaning,
petitions beginning with “how to” and “I cannot”
surpass others in quantity; third, under the
redundancy surmise of Internet content, a single
syntax should have questioned most aspects of a
concept.
2.2 Trouble Detection
As a CAE task proceeds, the concept list C and
potential trouble pool P are dynamically changing.
At any time the task owner encounters difficulty and
stops to check the experience feeder, we must assess
the task context and come up with remedies for the
trouble that the task owner is most probably facing.
This is done by approximating the probability P(t|C)
for every potential trouble t in P:
(,)
(|)
()
1
( , )
p
q max{ ( ) ( , )}
z max{ ( ) { ( , , )}}
ij
ij
ij
ij x
ij i x
Pt
Pt
P
Pt
wxPt c
wx ev c c
C
C
C
C
(1)
where
subscript i ranges over the m concepts in
C, and subscript ij denotes the jth verb
that has c
i
as its direct object ;
x
≠i ;
w(x) is weight function ;
e(v
ij
,c
i
,c
x
) denotes any experience piece
that contains the three keywords: v
ij
, c
i
and c
x
;
z is normalizer.
The three approximate equalities each have its
meaning:
The first approximate equality means the
chance of any specific concept series is treated
as equal.
The second approximate equality significantly
reduces the scale of joint distribution of
concepts out of computational complexity
concern. Besides, we add a weight function
here to gain some control over the choice of
concepts. An instinctive idea is to use more
recent concepts to infer possible troubles.
The third approximate equality relaxes the
verb-object constraint between v
ij
and c
i
when
using them to retrieve evidence. This is
because it is impractical to parse every
sentence while searching a gigantic corpus.
Whenever a new concept is captured and
changes C, we recalculate P(t|C) for every potential
problem in P. For some most probable troubles, we
would search for relevant experiences and
recommend them to the task owner.
2.3 Experience Retrieval
To retrieve a piece of experience as candidate
remedy for sensed trouble (suppose the most
troublesome is t
ij
), we use three features of textual
experience for match making: trouble mentioning,
context overlap, and procedural marks.
Trouble mentioning: an empirical remedy should
explicitly mention the target trouble, t
ij
, which is
described by a verb-object pair. To do this, a natural
language parser is needed.
Context overlap: for a piece of textual experience,
KEOD2012-InternationalConferenceonKnowledgeEngineeringandOntologyDevelopment
344