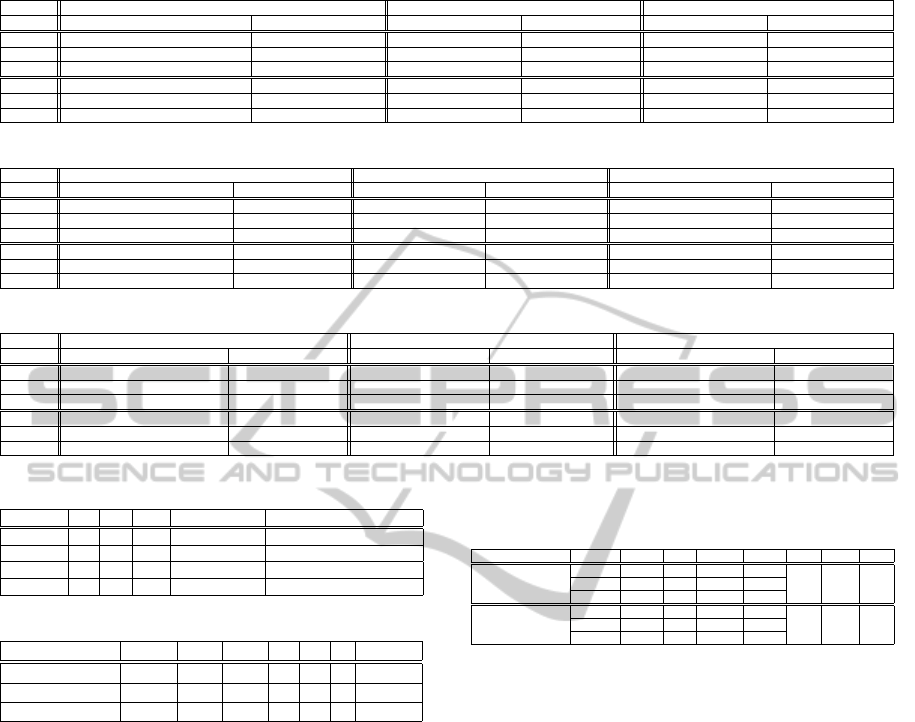
Table 2: Clustering result for the adult data set.
Algorithm Semi-supervised Conversion Splitting
Data type Numerical Categorical Numerical Categorical Numerical Categorical
DB Index 3.09 ±1.09[0.42,3.44,3.77] 1.22 ±0.14[1.10,1.12,1.40] 11.53 ±7.70[1.48,12.31,26.72] 1.50 ± 0.23[1.22,1.43, 1.92] 3.29 ± 0.001[3.29,3.29, 3.29] 1.15 ± 0.09[1.11,1.12, 1.37]
Silh. Index 0.29 ±0.18[0.18,0.21,0.71] 0.25 ±0.01[0.23,0.24,0.27] 0.07 ±0.05[−0.02,0.08,0.17] 0.22 ±0.02[0.19,0.21,0.25] 0.21 ±0.0[0.21,0.21,0.21] 0.25 ± 0.01[0.24,0.24, 0.27]
Dunn Index 1.1e − 4 ±4.2e − 4[1.2e − 5,1.2e − 5,1.1e − 3] 0.125 ±0.0[0.125,0.125,0.125] 0 ±0.00[0.00,0.00,0.00] 0.00 ± 0.00[0.00,0.00, 0.00] 0 ±0.00[0.00,0.00,0.00] 0.125 ± 0[0.125,0.125,0.125]
Purity 0.62 ±0.07[0.52,0.60,0.75] 0.59 ±0.06[0.50,0.56,0.67] 0.62 ±0.11[0.25,0.71,0.75] 0.56 ± 0.04[0.53,0.55, 0.65] 0.64 ± 0.001[0.64,0.64, 0.64] 0.59 ± 0.05[0.55,0.55,0.67]
Entropy 0.77 ± 0.03[0.72,0.78,0.79] 0.73 ±0.02[0.71,0.73,0.78] 0.73 ±0.06[0.69,0.69,0.81] 0.73 ± 0.02[0.70,0.74, 0.75] 0.71 ± 0.00[0.71, 0.71,0.71] 0.73 ± 0.01[0.71,0.73, 0.73]
NMI 0.06 ±0.03[0.02,0.05,0.10] 0.09 ±0.001[0.08,0.09,0.11] 0.08 ±0.07[2.1e− 4, 0.13,0.13] 0.09 ± 0.02[0.07,0.08, 0.12] 0.10 ± 0.00[0.10, 0.10,0.10] 0.09 ± 0.01[0.08,0.08, 0.11]
Table 3: Clustering result for the heart disease data set.
Algorithm Semi-supervised Conversion Splitting
Data type Numerical Categorical Numerical Categorical Numerical Categorical
DB Index 1.73 ±0.15[1.54, 1.71,2.14] 0.80 ±0.17[0.65, 0.77,1.42] 2.97± 0.56[0.21,2.95, 5.16] 1.13 ± 0.09[0.98,1.09, 1.35] 1.65 ±0.003[1.65, 1.65,1.65] 0.75 ±0.06[0.75, 0.75,0.77]
Silh. Index 0.33± 0.04[0.26, 0.33,0.41] 0.29± 0.02[0.23, 0.30,0.31] 0.26 ± 0.07[0.16,0.25, 0.75] 0.18 ±0.005[0.16, 0.18,0.19] 0.36 ±0.005[0.36, 0.36,0.36] 0.31 ±0.005[0.29, 0.31,0.31]
Dunn Index 3.3e −3 ± 2.2e − 3[1.2e − 5, 2.3e − 4, 0.35] 0.14 ± 0[0.14, 0.14,0.14] 0.04 ± 0.14[0.015, 0.015,0.98] 0.13 ± 0.04[0.07,0.15,0.23] 4.6e − 3 ±0[4.6e − 3,4.6e − 3,4.6e − 3] 0.14 ±0[0.14, 0.14,0.14]
Purity 0.72 ±0.03[0.65, 0.72,0.76] 0.78 ±0.03[0.71, 0.77,0.81] 0.77± 0.11[0.47,0.82, 0.82] 0.78 ± 0.02[0.75,0.76, 0.83] 0.75 ±0.003[0.75, 0.75,0.75] 0.81 ±0.01[0.78, 0.81,0.81]
Entropy 0.84 ±0.03[0.79, 0.84,0.91] 0.74 ±0.04[0.70, 0.75,0.87] 0.72± 0.11[0.67,0.67, 0.99] 0.74 ± 0.04[0.64,0.75, 0.81] 0.80 ±0.003[0.80, 0.80,0.81] 0.71 ±0.02[0.69, 0.69,0.76]
NMI 0.15 ±0.03[0.08, 0.16,0.20] 0.25 ±0.04[0.13, 0.24,0.30] 0.28 ± 0.11[2.1e − 4,0.32,0.32] 0.26 ± 0.04[0.18, 0.25,0.36] 0.19 ± 0.004[0.18,0.19, 0.19] 0.29± 0.02[0.23,0.30, 0.30]
Table 4: Clustering result for the credit card data set.
Algorithm Semi-supervised Conversion Splitting
Data type Numerical Categorical Numerical Categorical Numerical Categorical
DB Index 1.98 ±0.63[0.01,2.06,3.81] 1.41± 0.31[0.97,1.38, 1.95] 4.94 ±2.44[0.10, 4.87,8.57] 1.75 ± 0.22[1.32,1.69, 2.44] 1.89± 0.35[0.18, 1.97,1.97] 1.81 ±0.25[1.37,1.83,2.87]
Silh. Index 0.56 ±0.14[0.20,0.55,0.97] 0.23± 0.05[0.16,0.23, 0.36] 0.35 ±0.27[0.12, 0.29,0.92] 0.17 ± 0.02[0.13,0.16, 0.21] 0.63± 0.06[0.62, 0.62,0.95] 0.23 ±0.01[0.19,0.23,0.24]
Dunn Index 0.0078 ± 0.0497[1.2e −5, 2.3e − 4,0.35] 0.12 ± 0.03[0.11,0.11, 0.22] 0.06 ± 0.15[1.1e − 3, 0.011,0.77] 0.07 ± 0.002[0.07,0.07, 0.08] 0.003 ± 0.012[1.1e −4,1.1e − 4,0.06] 0.12 ± 0.01[0.11,0.12, 0.13]
Purity 0.65 ±0.05[0.47, 0.66,0.70] 0.73 ± 0.08[0.54,0.77, 0.80] 0.65± 0.12[0.48, 0.56,0.81] 0.77 ± 0.02[0.69,0.78, 0.82] 0.64± 0.02[0.56,0.64, 0.64] 0.79 ±0.01[0.76, 0.79,0.82]
Entropy 0.91 ±0.04[0.84,0.91,0.99] 0.80± 0.08[0.70,0.78, 0.98] 0.86 ±0.13[0.68, 0.97,0.99] 0.77 ± 0.03[0.67,0.76, 0.87] 0.93± 0.01[0.93, 0.93,0.98] 0.73 ±0.02[0.65,0.73,0.78]
NMI 0.10 ±0.04[1.3e− 4, 0.09,0.18] 0.19 ±0.08[0.01, 0.22,0.30] 0.13 ± 0.13[1.2e − 4,0.03, 0.31] 0.23 ±0.03[0.12, 0.23,0.31] 0.08 ± 0.01[0.03,0.08, 0.08] 0.26± 0.02[0.22, 0.27,0.36]
Table 5: Basic characteristics of the Iris data set.
Attribute Min Max Mean Standard deviation Class Correlation (Pearson‘s CC)
Sepal length 4.3 7.9 5.84 0.83 0.7826
Sepal width 2.0 4.4 3.05 0.43 −0.4194
Petal length 1.0 6.9 3.76 1.76 0.9490
Petal width 0.1 2.5 1.20 0.76 0.9565
Table 6: Overview of the experiments.
Experiment Number Data set T
1
T
2
k
T
1
k
T
2
K Threshold
1 Iris {1} {3} 2 3 3 0.6
2 Iris {2} {4} 2 3 3 0.6
3 Iris {1, 3} {2, 4} 2 3 3 0.6
ize for different domains, because we do not exploit
any attribute-based distance measure between the dif-
ferent data domains (as would be the case for really
different domains). The Iris data set is a benchmark
set that contains 3 classes of 50 data instances each,
where each class refers to a type of iris plant: iris Se-
tosa, iris Versicolour, iris Virginica.
4.3.2 Experiments
All three experiments were performed on the Iris data
set. We repeated each experiment 10 times and re-
port only the best results. For validation purposes, we
used the class labels. For class - cluster assignment
we used the Jaccard coefficient, meaning that a class
will be assigned to the cluster with highest Jaccard
coefficient.
Experiment 1. We first take only the first and third
features of the IRIS data set. Let T
1
be the sepal length
(first feature) and T
2
be the petal length (third feature).
Table 7: Experiment 1: Evaluation measures for the semi-
supervised merging algorithm and k-means (bold results are
best).
Algorithm Class Precision Recall F-measure Accuracy Purity Entropy NMI
K-means
Setosa 0.9804 1.0 0.9901 0.9933
0.8800 0.2967 0.7065
Versicolour 0.7758 0.9 0.8333 0.88
Virginica 0.9224 0.74 0.8132 0.8868
Semi-supervised merging
Setosa 1.0 1.0 1.0 1.0
0.9467 0.1642 0.8366
Versicolour 0.8889 0.96 0.9231 0.9467
Virginica 0.9565 0.88 0.9167 0.9467
We chose those two features because the first feature
has a low class correlation index while the third fea-
ture has a high class correlation index. The first ex-
periment was performed in the following steps:
1. Cluster the data in domain T
1
using k-means
(k = 2)
2. Cluster the data in domain T
2
using k-means
(k = 3)
3. Merge the two clustering results using the merg-
ing algorithm described in Algorithm 1. Notice
that one of the output parameters is K, which is
the number of clusters after merging. We set the
overlap threshold parameter to th = 0.6.
4. Compare this result with the clustering result of
k-means using k = K clusters, performed on do-
mains T
1
and T
2
together.
Table 7 shows the results in terms of classification ac-
curacy, precision, recall, F-measure, purity, entropy
and NMI for each algorithm (the results in bold are
better).
Experiment 2. We next performed a similar exper-
iment to the above but this time, domain T
1
is the 2nd
KDIR2012-InternationalConferenceonKnowledgeDiscoveryandInformationRetrieval
52