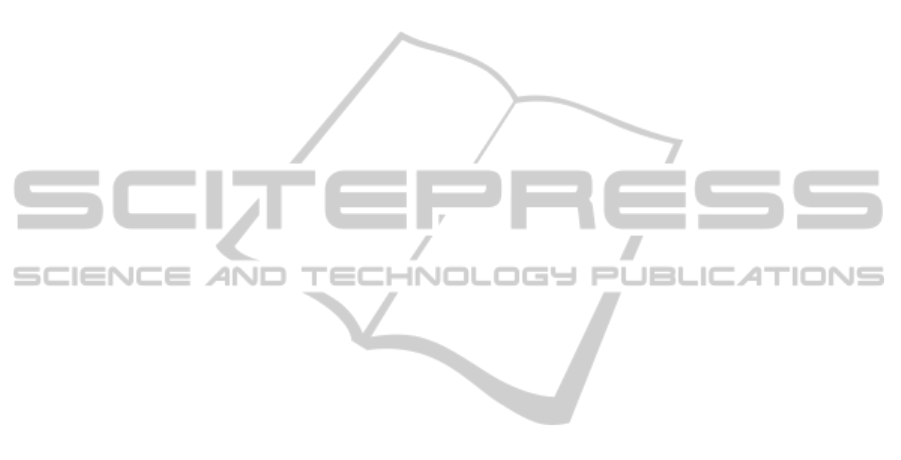
liminary term grouping. Even when only morpho-
syntactic features are available, the results are of a
quality good enough to be used as a starting point
for further processing which may involve manual cor-
rection. The achieved F-measure of about 57% is
not high, but in case of this task, which also proved
difficult for well trained annotators, can be seen as
good enough to be utilized in further domain ontology
development. However, it turned out that morpho-
syntactic information is not sufficient to build reli-
able clusters—additional sources of information will
be searched for to improve quality of the results e.g.
Wordnet which can be used to define semantic simi-
larity between head elements of the different terms.
REFERENCES
Amig´o, E., Gonzalo, J., Artiles, J., and Verdejo, F. (2009).
A comparison of extrinsic clustering evaluation met-
rics based on formal constraints. Information Re-
trieval, 12(5):613.
Bagga, A. and Baldwin, B. (1998). Algorithms for scoring
coreference chains. In LREC Workshop on Linguistics
Coreference, pages 563–566.
Baneyx, A., Charlet, J., and Jaulent, M.-C. (2006). Method-
ology to build medical ontology from textual re-
sources. AMIA Annual Symposium proceedings,
2006:21–25.
Cimiano, P. (2006) Ontology Lerning and Population from
Text. pages 85–184. Springer.
Fernndez, A. and Gmez, S. (2008). Solving non-uniqueness
in agglomerative hierarchical clustering using multi-
dendrograms. Journal of Classification, 25:43–65.
Frantzi, K., Ananiadou, S., and Mima,2000). Automatic
recognition of multi-word terms: the C-value/NC-
value method. Int. Journal on Digital Libraries,
3:115–130.
Ittoo, A. and Maruster, L. (2009). Ensemble similar-
ity measures for clustering terms. In Congres on
Computer Science and Information Engineering, vol-
ume 4, pages 315–319.
Le Moigno, S., Charlet, J., Bourigault, D., Degoulet, P., and
Jaulent, M.-C. (2002). Terminology extraction from
text to build an ontology in surgical intensive care. In
Proceedings of the Workshop Machine Learning and
Natural Language Processing for Ontology Engineer-
ing.
Lin, D. and Pantel, P. (2001). Induction of semantic classes
from natural language text. In KDD’01, pages 317–
322.
Navigli, R., Velardi, P., and Gangemi, A. (2003). Ontology
learning and its application to automated terminology
translation. Intelligent Systems, IEEE, 18(1):22 – 31.
Nenadi´c, G., Spasi´c, I., and Ananiadou, S. (2004). Auto-
matic discovery of term similarities using pattern min-
ing. Int. Journal of Terminology, 10(1):55–80.
Nenadic, G., Spasic, I., and Ananiadou, S. (2006). Term
clustering using a corpus-based similarity measure.
In Sojka, P., Kopecek, I., and Pala, K., editors, Text,
Speech and Dialogue, volume 2448 of LNCS, pages
89–109. Budapest, Hungary.
Pedersen, T., Pakhomov, S. V., Patwardhan, S., and Chute,
C. G. (2007). Measures of semantic similarity and re-
latedness in the biomedical domain. J. of Biomedical
Informatics, 40(3):288–299.
Piasecki, M. (2007). Polish tagger TaKIPI: Rule based
construction and optimisation. Task Quarterly, 11(1–
2):151–167.
Ushioda, A. (1996). Hierarchical clustering of words. In
Proceedings of the 16th conference on Computational
linguistics - Volume 2, COLING ’96, pages 1159–
1162, Stroudsburg, PA, USA. ACL.
Woliski, M. (2006). Morfeusz—a Practical Tool for
the Morphological Analysis of Polish. In Kopo-
tek, M., Wierzcho, S., and Trojanowski, K., eds,
Intelligent Information Processing and Web Mining,
IIS:IIPWM’06, pages 503–512. Springer.
ClusteringofMedicalTermsbasedonMorpho-syntacticFeatures
219