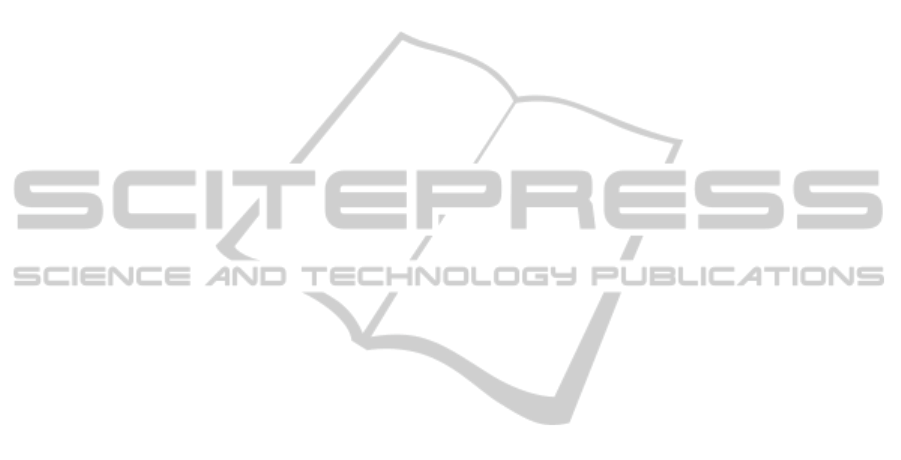
rate is affected differently by opening the tank or a
flowmeter fault. Using ontologies and the R language,
we have been able to go beyond the limits of the sys-
tems generally used.
The component developed uses an ontology for
symbolic data and the R language to fit the curves on
measurements using a non parametric method (spline
estimator) under constraints. The curve shape depend
on the event sequence. To ensure a good fit, the num-
ber of nodes and the constraints are generated by us-
ing the event ontology and a new dedicated one on-
tology for impacts. This ontology of application was
designed to classify impacts of events on kinetics and
to be able to automatically generate a specific R treat-
ment. So, this component uses the fitted curve, the
type of event (operation or fault) and comments from
experts (Neveu et al., 2008) to validate or invalidate
the measurements. To quantify the efficiency of the
application, we analysed 20 fermentations with many
operations and malfunctions. A detailed analysis of
the dCO
2
/dt curves by experts pointed out 242 wrong
or litigious measurements (among more than 20 000).
Using the application, we found very similar results,
with only 21 misdiagnoses, i.e. less than 9% differ-
ence. This result indicates a very good rate of data
invalidation and should prove a good behavior of our
ontologies.
Perspectives: Determination of the Usefulness of
Fermentations. Another concern of users is the
confidence they can have in the fermentation and
whether the data can be used in other contexts for an-
alytical and diagnostic purposes. Certain faults (de-
fault of temperature regulation, for example) happen
at key moments and are therefore disastrous, prevent-
ing the subsequent use of the fermentation data. A de-
scription of this knowledge as a function of the type
and time of the fault is essential, to enable the in-
formation system to determine whether a data set is
valid. This requires taking the investigation further
and seeing whether the whole data set can be inter-
preted.
5 CONCLUSIONS
Advances in alcoholic fermentation monitoring and
control necessitate new tools for data management.
Databases need to be associated with tools for the
representation and management of knowledge. This
study shows an example of a reliable and user-
friendly application based on ontologies, with the
aid of Semantic Web tools and technologies (JENA,
CORESE, PROTEGE, etc.). These languages and
softwares, such as RDF(S) and OWL provide reason-
ing and they are of great interest for the management
of complex data.
The applications developed automatically detect
the inconsistencies caused by the principal malfunc-
tions during the fermentations and distinguishes be-
tween those of little importance and those that might
disrupt the process. Disruptions related to enological
operations (such as cap punching, pumping over) are
also detected and analysed. The application was vali-
dated using a database containing about 4500 fermen-
tations. It might represent a powerful tool to improve
fermentation management and control in the near fu-
ture.
The method of formalization that separates the
expert knowledge allows to take into account eas-
ily changing business practices. It allows to easily
adapted the developed software for other cases in food
process (cheese making, brewinb, etc).
REFERENCES
Aguera, E., Bes, M., Roy, A., Camarasa, C., and Sablay-
rolles, J.-M. (2010). Partial removal of ethanol during
fermentation to obtain reduced-alcohol wines. Ameri-
can Journal of Enology and Viticulture, 61 (1):53–60.
Corby, O., Dieng-Kuntz, R., and Faron-Zucker, C. (2004).
Querying the semantic web with corese search engine.
Proceedings of European Conference on Artificial In-
telligence (ECAI) Valencia, Spain, pages 705–709.
Hignette, G., Buche, P., Dibie-Barth
´
elemy, J., and Haem-
merl
´
e, O. (2007). Semantic annotation of data tables
using a domain ontology. In Discovery Science, pages
253–258. Springer.
Neveu, P., Rossard, V., Aguera, E., Perez, M., Picou, C.,
and Sablayrolles, J.-M. (2008). Gestion de donn
´
ees
et de connaissances pour les bioproc
´
ed
´
es. Extrac-
tion et Gestion des Connaissances, Atelier : fouille
de donn
´
ees temporelles:21–28.
Noy, N. and McGuinness, D. (2001). Ontology develop-
ment 101. Knowledge Systems Laboratory, Stanford
University.
Sall, O., Lo, M., Gandon, F., Niang, C., and Diop, I. (2009).
Using xml data integration and ontology reuse to share
agricultural data. International Journal of Metadata,
Semantics and Ontologies, 4:93–105.
KEOD2012-InternationalConferenceonKnowledgeEngineeringandOntologyDevelopment
372