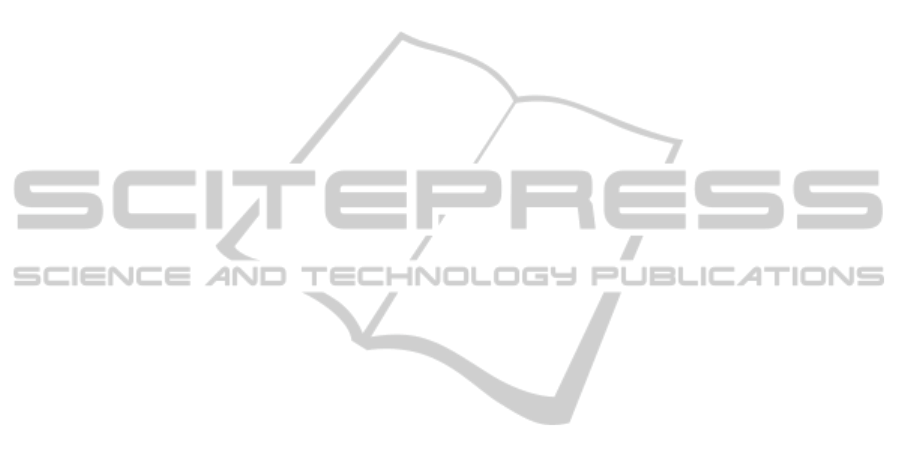
Here the concept DOG is represented as a
subconcept of MAMMAL. Since DL networks can
express only necessary and/or sufficient conditions,
some details of the representation are very loose. For
example, according to fig. 3, a DOG may or may not
have a tail (this is the expressed by the number
restriction 0/1 imposed on the attribute has_tail),
and has an unspecified number of limbs (since some
dogs could have lost limbs, and teratological dogs
could have more than four legs). LASSIE and RIN
TIN TIN are represented as individual instances of
DOG (of course, concepts describing individual
instances can be further detailed, fully specifying for
example the values of the attributes inherited from
parent concepts).
Prototypes describing typical instances of
concepts are represented as data structures that are
external to the DL knowledge base. Such structures
could, for example, be lists of (possibly weighted)
attribute/value pairs that are linked to the
corresponding concept. Some attributes of the list
should correspond to attributes of the DL concept,
which value can be further specified at this level.
For example, the prototypical dog is described as
having a tail, and exactly four legs. Other attributes
of the prototype could have no counterpart in the
corresponding DL concept.
As far as the exemplar-based component of the
representations is concerned, exemplars are directly
represented in the DL knowledge base as instances
of concepts. (It may also happen that some
information concerning exemplars is represented
outside the DL component, in the form of Linked
Data. Typically, this could be the case of “non
symbolic” information, such as images, sounds,
etc.).
It must be noted that prototypical information
about concepts (either stored in the form of
prototypes or extracted from the representation of
exemplars) extends the information coded within the
DL formalism. The semantic network provides
necessary and/or sufficient conditions for the
application of concepts, as a consequence, such
conditions hold for every instance of concepts, and
cannot be violated by any specific exemplar. So,
what can be inferred on the basis of prototypical
knowledge can extend, but can in no way conflict
with what can be deduced from the DL based
component.
6 CONCLUSIONS
In conclusion, we assume that a hybrid
prototype/exemplar based representation of non
classical concepts could make ontological
representation of common-sense concepts more
flexible and realistic, thus avoiding at the same time
some frequent misuses of DL formalisms.
As a further development of the work presented
here, we are currently investigating the possibility of
adopting conceptual spaces (Gärdenfors, 2000) as an
adequate framework for representing both
prototypes and exemplars in many different
contexts. Gärdenfors (2004) and others (Adams and
Raubal, 2009) proposed conceptual spaces as a tool
for representing knowledge in the semantic web.
From our point of view, conceptual spaces could
offer a common, computational framework do
develop our proposal of representing concepts in
terms of both prototypes and exemplars.
REFERENCES
Adams B., and Raubal M., 2009. The Conceptual Space
Markup Language (CSML): Towards the Cognitive
Semantic Web. 3
rd
IEEE Int. Conf. on Semantic
Computing (ICSC 2009), Berkeley, CA, 253-260.
Baader F., D. Calvanese, D. McGuinness, D. Nardi, P.
Patel-Schneider, 2010. The Description Logic
Handbook, 2
nd
edition, CP, Cambridge, UK.
Brachman R., Levesque, H. (eds.), 1985. Readings in
Knowledge Representation, Morgan Kaufmann, Los
Altos, CA.
Brachman R., J. G. Schmolze, 1985. An overview of the
KL-ONE knowledge representation system, Cognitive
Science 9, 171-216.
Frixione, M., Lieto, A., 1910. The Computational
Representation of Concepts in Formal Ontologies:
Some General Consideration, Proc. KEOD 2010,
Valencia, Spain, 396-403.
Frixione, M., Lieto, A., in press. Representing Concepts in
Formal Ontologies: Compositionality vs. Typicality
Effects, to appear in Logic and Logical Philosophy.
Gärdenfors, P., 2000. Conceptual Spaces: The Geometry
of Thought. The MIT Press, Cambridge, MA.
Gärdenfors, P. 2004. How to make the Semantic Web
more Semantic. Proc. 3rd Int. Conf. on Formal
Ontology in Inf. Syst. (FOIS), Torino, Italy.
Machery E., 2009. Doing without Concepts. Oxford
University Press, Oxford.
Malt B. C., 1989. An on-line investigation of prototype
and exemplar strategies in classification. J. Exp. Psyc.:
Learning, Memory, and Cognition 15(4), 539-555.
Medin D. L., Schaffer M. M., 1978. Context theory of
classification learning. Psychol. Rev. 85(3), 207-238.
Medin D. L., Schwanenflugel P. J., 1981. Linear
separability in classification learning. J. Exp. Psyc.:
Human Learning and Memory 7, 355-368.
Murphy G. L., 2002. The Big Book of Concepts. The MIT
Press, Cambridge, MA.
Nosofsky R. M., 1986. Attention, similarity, and the
PrototypesVsExemplarsinConceptRepresentation
231